On Resource-Efficient Bayesian Network Classifiers and Deep Neural Networks
international conference on pattern recognition(2021)
摘要
We present two methods to reduce the complexity of Bayesian network (BN) classifiers. First, we introduce quantization-aware training using the straight-through gradient estimator to quantize the parameters of BNs to few bits. Second, we extend a recently proposed differentiable tree-augmented naive Bayes (TAN) structure learning approach by also considering the model size. Both methods are motivated by recent developments in the deep learning community, and they provide effective means to trade off between model size and prediction accuracy, which is demonstrated in extensive experiments. Furthermore, we contrast quantized BN classifiers with quantized deep neural networks (DNNs) for small-scale scenarios which have hardly been investigated in the literature. We show Pareto optimal models with respect to model size, number of operations, and test error and find that both model classes are viable options.
更多查看译文
关键词
neural networks,resource-efficient
AI 理解论文
溯源树
样例
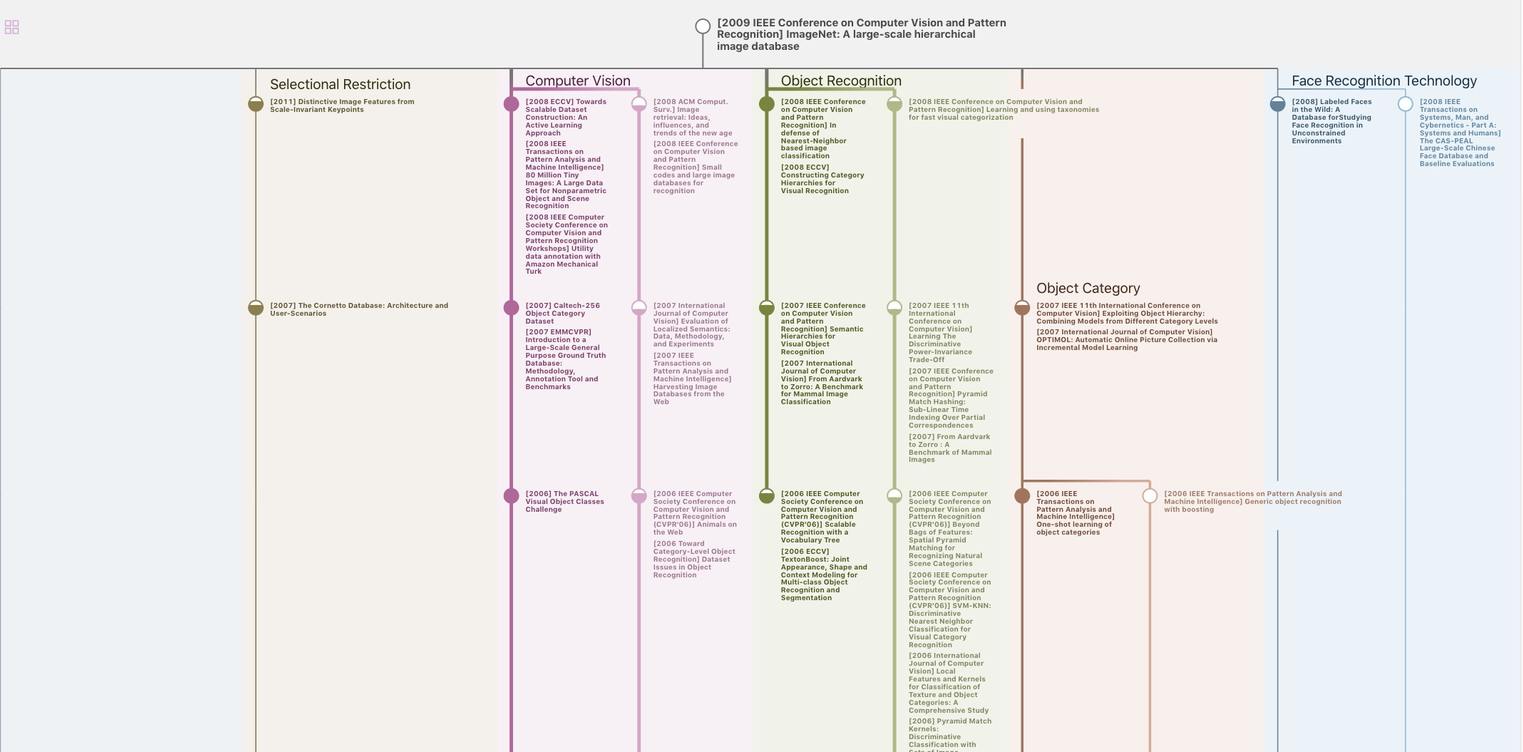
生成溯源树,研究论文发展脉络
Chat Paper
正在生成论文摘要