Automated Metadata Harmonization Using Entity Resolution and Contextual Embedding
Springer eBooks(2020)
摘要
Data curation process for Analytics and Data Science typically involves collecting data from large number of heterogenous and federated source systems with varied schema structures. To make these datasets interoperable, their metadata needs to be standardized. This process, also known as Metadata Harmonization, is predominantly a manual effort involving several hours of concentrated work that leads to reduced efficiency of ML-Ops lifecycle. This paper aims to demonstrate the automation of metadata harmonization using Machine Learning. It focuses on using entity resolution and contextual embedding methods to capture hidden relationships among data columns that help identify similarities in metadata, and thereby, help in automated mapping of columns to a standard schema. This study also addresses the automated derivation of the correct ontological structure for the target data model using ML. While prior competing approaches address manual metadata harmonization problem by proposing usage of semantic middleware, data dictionaries and matching rules this approach recommends novel usage of Machine Learning which improves efficacy of overall lifecycle.
更多查看译文
关键词
Metadata harmonization,Metadata crosswalking,Data curation,Metadata contextual embedding
AI 理解论文
溯源树
样例
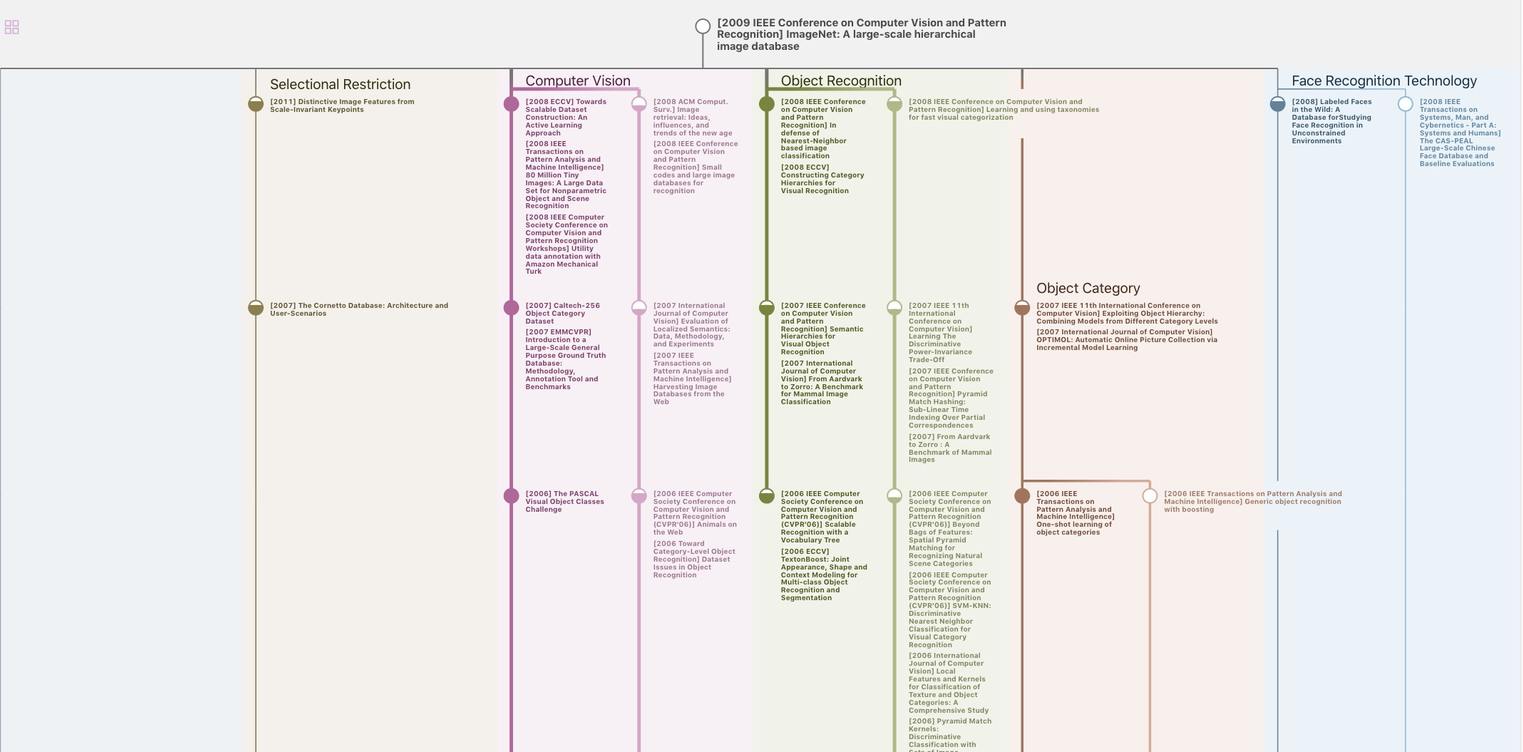
生成溯源树,研究论文发展脉络
Chat Paper
正在生成论文摘要