Is She Truly Enjoying the Conversation?: Analysis of Physiological Signals toward Adaptive Dialogue Systems
ICMI '20: INTERNATIONAL CONFERENCE ON MULTIMODAL INTERACTION Virtual Event Netherlands October, 2020(2020)
摘要
In human-agent interactions, it is necessary for the systems to identify the current emotional state of the user to adapt their dialogue strategies. Nevertheless, this task is challenging because the current emotional states are not always expressed in a natural setting and change dynamically. Recent accumulated evidence has indicated the usefulness of physiological modalities to realize emotion recognition. However, the contribution of the time series physiological signals in human-agent interaction during a dialogue has not been extensively investigated. This paper presents a machine learning model based on physiological signals to estimate a user's sentiment at every exchange during a dialogue. Using a wearable sensing device, the time series physiological data including the electrodermal activity (EDA) and heart rate in addition to acoustic and visual information during a dialogue were collected. The sentiment labels were annotated by the participants themselves and by external human coders for each exchange consisting of a pair of system and participant utterances. The experimental results showed that a multimodal deep neural network (DNN) model combined with the EDA and visual features achieved an accuracy of 63.2%. In general, this task is challenging, as indicated by the accuracy of 63.0% attained by the external coders. The analysis of the sentiment estimation results for each individual indicated that the human coders often wrongly estimated the negative sentiment labels, and in this case, the performance of the DNN model was higher than that of the human coders. These results indicate that physiological signals can help in detecting the implicit aspects of negative sentiments, which are acoustically/visually indistinguishable.
更多查看译文
AI 理解论文
溯源树
样例
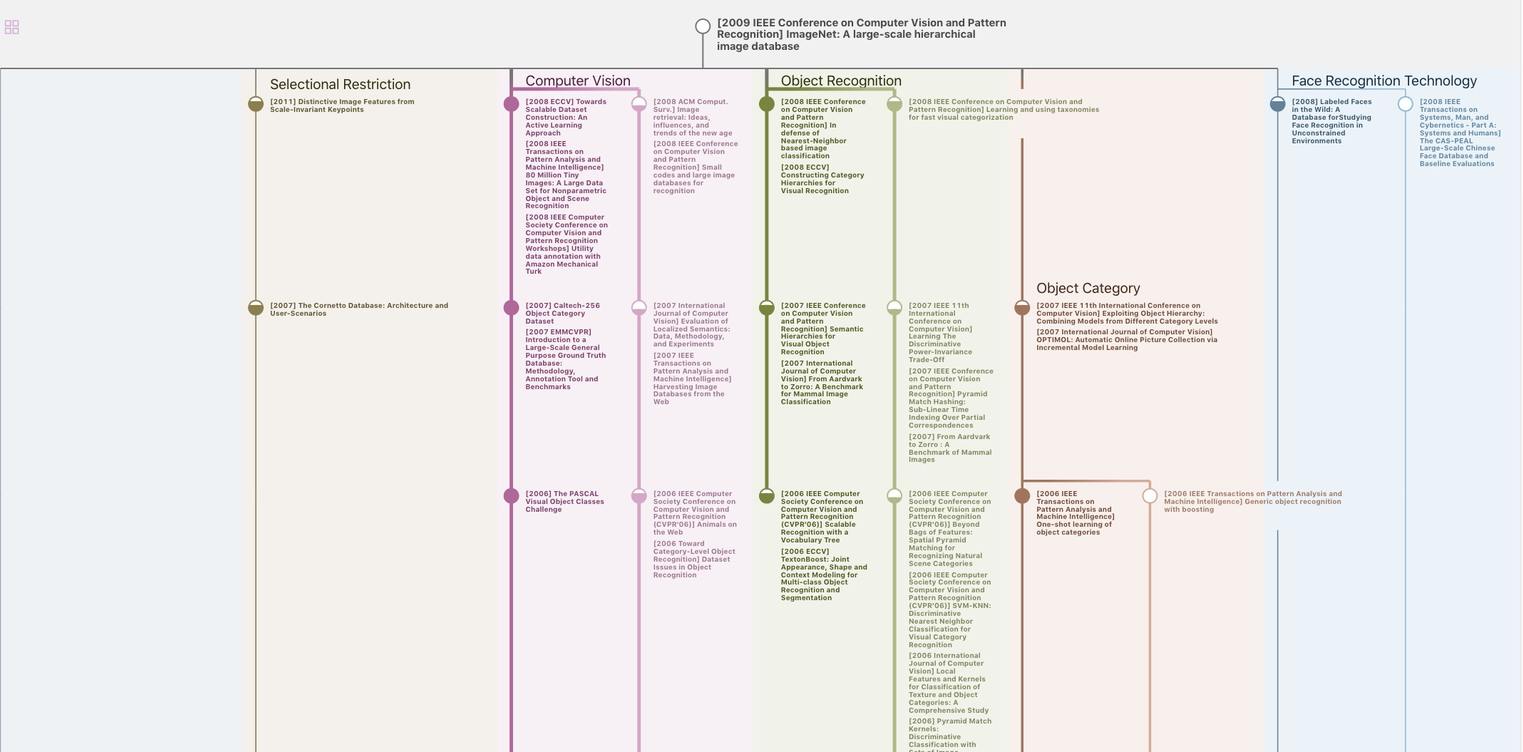
生成溯源树,研究论文发展脉络
Chat Paper
正在生成论文摘要