Improving the Taxonomy of Fossil Pollen Using Convolutional Neural Networks and Superresolution Microscopy
Proceedings of the National Academy of Sciences(2020)
摘要
Taxonomic resolution is a major challenge in palynology, largely limiting the ecological and evolutionary interpretations possible with deep-time fossil pollen data. We present an approach for fossil pollen analysis that uses optical superresolution microscopy and machine learning to create a quantitative and higher throughput workflow for producing palynological identifications and hypotheses of biological affinity. We developed three convolutional neural network (CNN) classification models: maximum projection (MPM), multislice (MSM), and fused (FM). We trained the models on the pollen of 16 genera of the legume tribe Amherstieae, and then used these models to constrain the biological classifications of 48 fossil Striatopollis specimens from the Paleocene, Eocene, and Miocene of western Africa and northern South America. All models achieved average accuracies of 83 to 90% in the classification of the extant genera, and the majority of fossil identifications (86%) showed consensus among at least two of the three models. Our fossil identifications support the paleobiogeographic hypothesis that Amherstieae originated in Paleocene Africa and dispersed to South America during the Paleocene-Eocene Thermal Maximum (56 Ma). They also raise the possibility that at least three Amherstieae genera (Crudia, Berlinia, and Anthonotha) may have diverged earlier in the Cenozoic than predicted by molecular phylogenies.
更多查看译文
关键词
Airyscan microscopy,automated classification,Detarioideae,machine learning,palynology
AI 理解论文
溯源树
样例
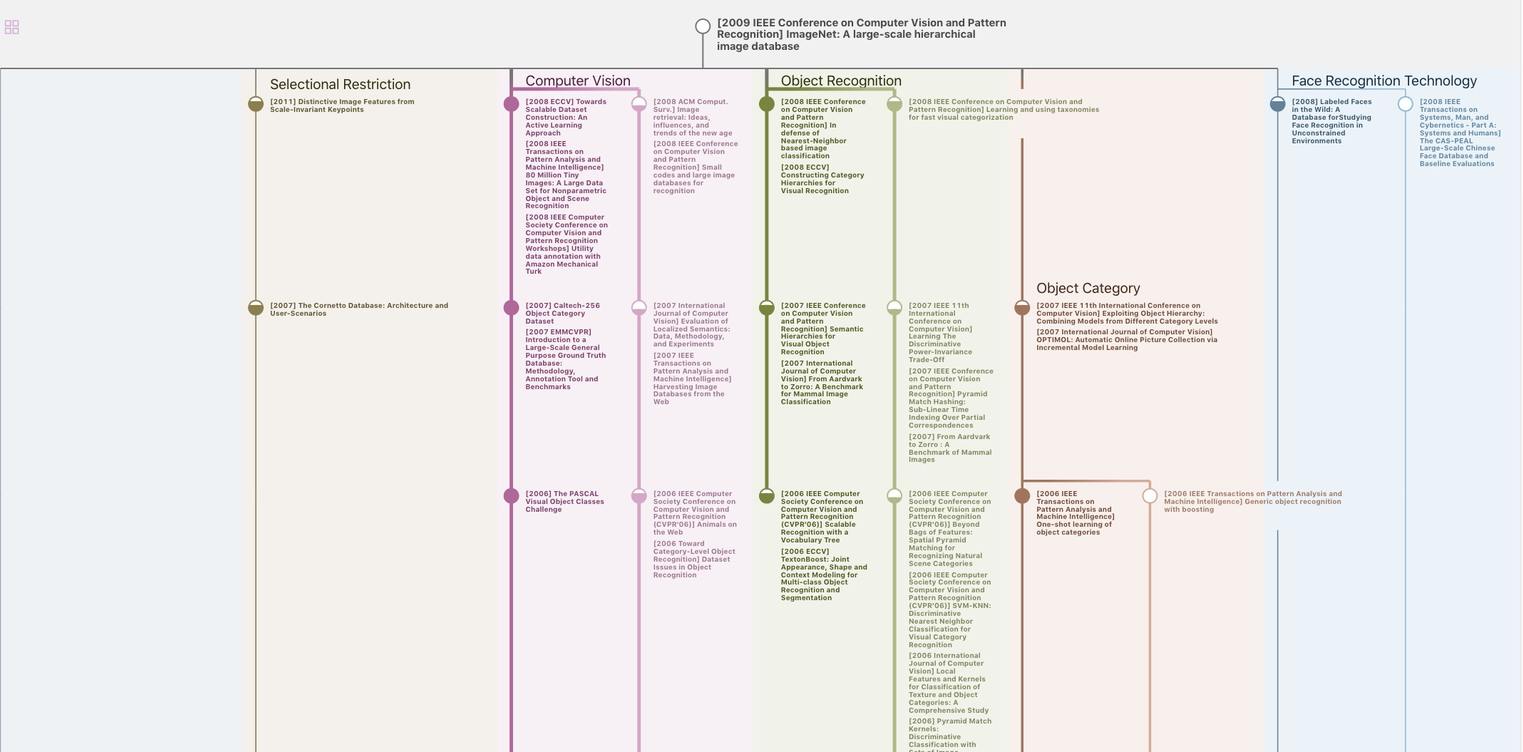
生成溯源树,研究论文发展脉络
Chat Paper
正在生成论文摘要