Machine learning many electron wave functions via backflow transformations
Phys. Rev. Lett(2019)
摘要
The goal of determining the electronic structure of molecules and materials by solving the many-body Schrödinger equation has challenged theoretical physics and chemistry over the last century and driven the development of powerful approximations and computational methods. In the three papers above [1, 2, 3], the authors show how deep learning architectures can systematically improve many-body wave functions for Quantum Monte Carlo calculations, and benchmark their accuracy on the Hubbard model [1], and for light atoms and small molecules [2, 3]. Out of several recent approaches for machine learning quantummany-body wave functions, eg [4, 5, 6, 7], I have chosen these three papers biased by the common underlying strategy taken there to explore, extend, and possibly exhaust existing structures of many-body trial wave functions based on backflow transformations employing neural networks.
更多查看译文
AI 理解论文
溯源树
样例
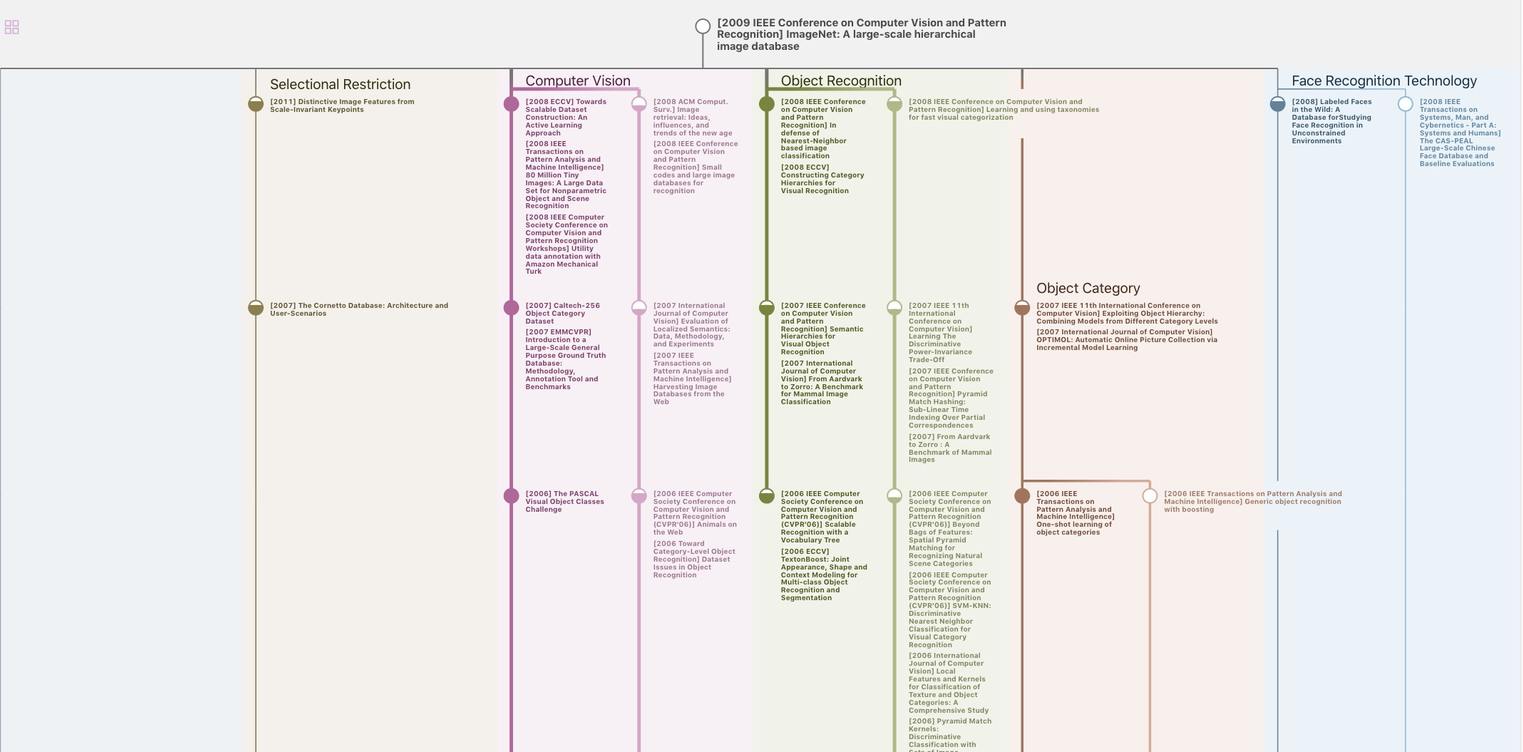
生成溯源树,研究论文发展脉络
Chat Paper
正在生成论文摘要