Step size Adaptation Using Exponentiated Gradient Updates
Workshop on "Beyond first-order methods in ML systems" at the 37th International Conference on Machine Learning (ICML)(2020)
摘要
Optimizers like Adam and AdaGrad have been very successful in training large-scale neural networks. Yet, the performance of these methods is heavily dependent on a carefully tuned learning rate schedule. We show that in many large-scale applications, augmenting a given optimizer with an adaptive tuning method of the step-size greatly improves the performance. More precisely, we maintain a global step-size scale for the update as well as a gain factor for each coordinate. We adjust the global scale based on the alignment of the average gradient and the current gradient vectors. A similar approach is used for updating the local gain factors. This type of step-size scale tuning has been done before with gradient descent updates. In this paper, we update the step-size scale and the gain variables with exponentiated gradient updates instead. Experimentally, we show that our approach can achieve compelling accuracy on standard models without using any specially tuned learning rate schedule. We also show the effectiveness of our approach for quickly adapting to distribution shifts in the data during training.
更多查看译文
关键词
adaptation,gradient,step-size
AI 理解论文
溯源树
样例
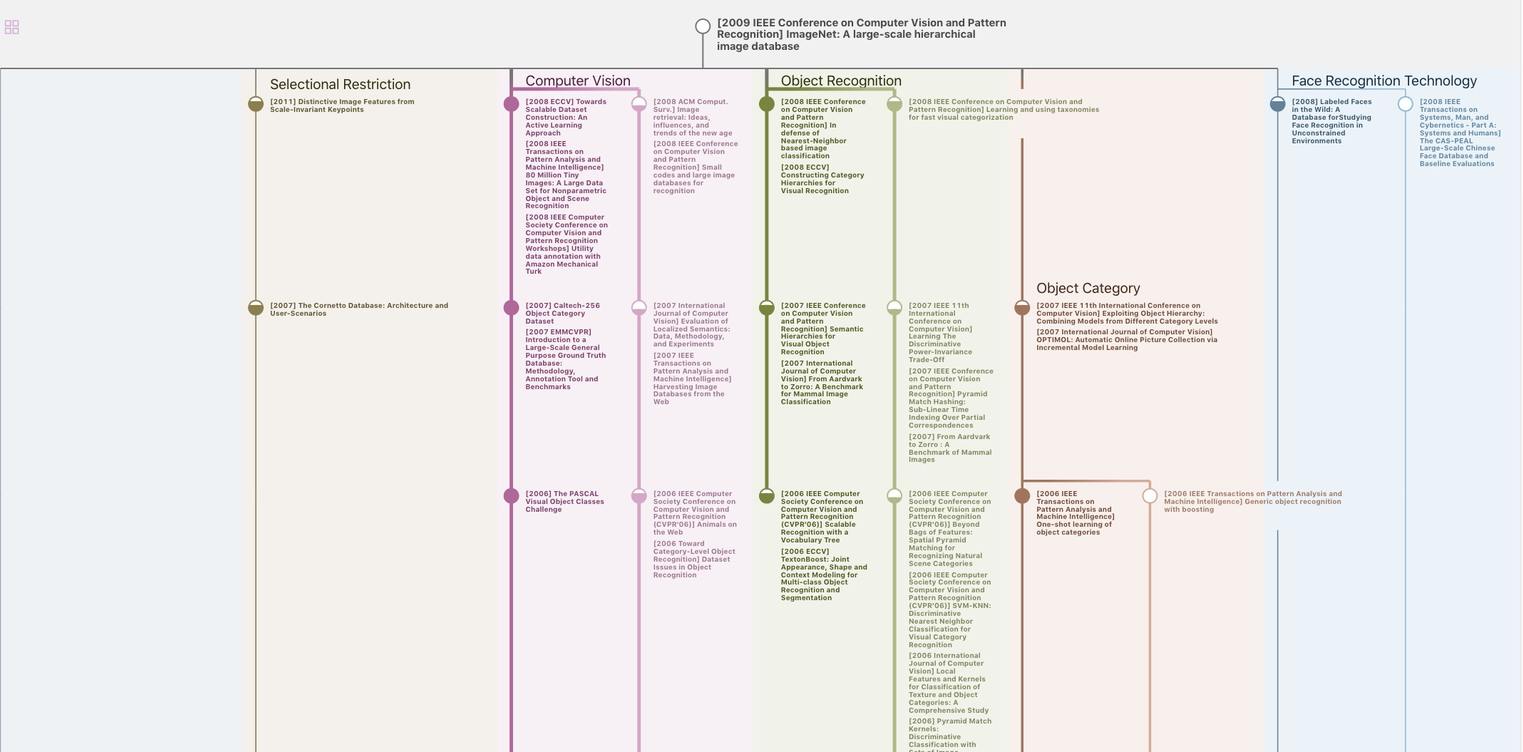
生成溯源树,研究论文发展脉络
Chat Paper
正在生成论文摘要