SMBOP: Semi-autoregressive Bottom-up Semantic Parsing
SPNLP 2021: THE 5TH WORKSHOP ON STRUCTURED PREDICTION FOR NLP(2021)
摘要
The de-facto standard decoding method for semantic parsing in recent years has been to autoregressively decode the abstract syntax tree of the target program using a top-down depth-first traversal. In this work, we propose an alternative approach: a Semi-autoregressive Bottom-up Parser (SMBOP) that constructs at decoding step t the top-K sub-trees of height <= t. Our parser enjoys several benefits compared to top-down autoregressive parsing. From an efficiency perspective, bottom-up parsing allows to decode all sub-trees of a certain height in parallel, leading to logarithmic runtime complexity rather than linear. From a modeling perspective, a bottom-up parser learns representations for meaningful semantic sub-programs at each step, rather than for semantically-vacuous partial trees. We apply SMBOP on SPIDER, a challenging zero-shot semantic parsing benchmark, and show that SMBOP leads to a 2.2x speed-up in decoding time and a similar to 5x speed-up in training time, compared to a semantic parser that uses autoregressive decoding. SMBOP obtains 71.1 denotation accuracy on SPIDER, establishing a new state-of-the-art, and 69.5 exact match, comparable to the 69.6 exact match of the autoregressive RAT-SQL+GRAPPA.
更多查看译文
关键词
semi-autoregressive
AI 理解论文
溯源树
样例
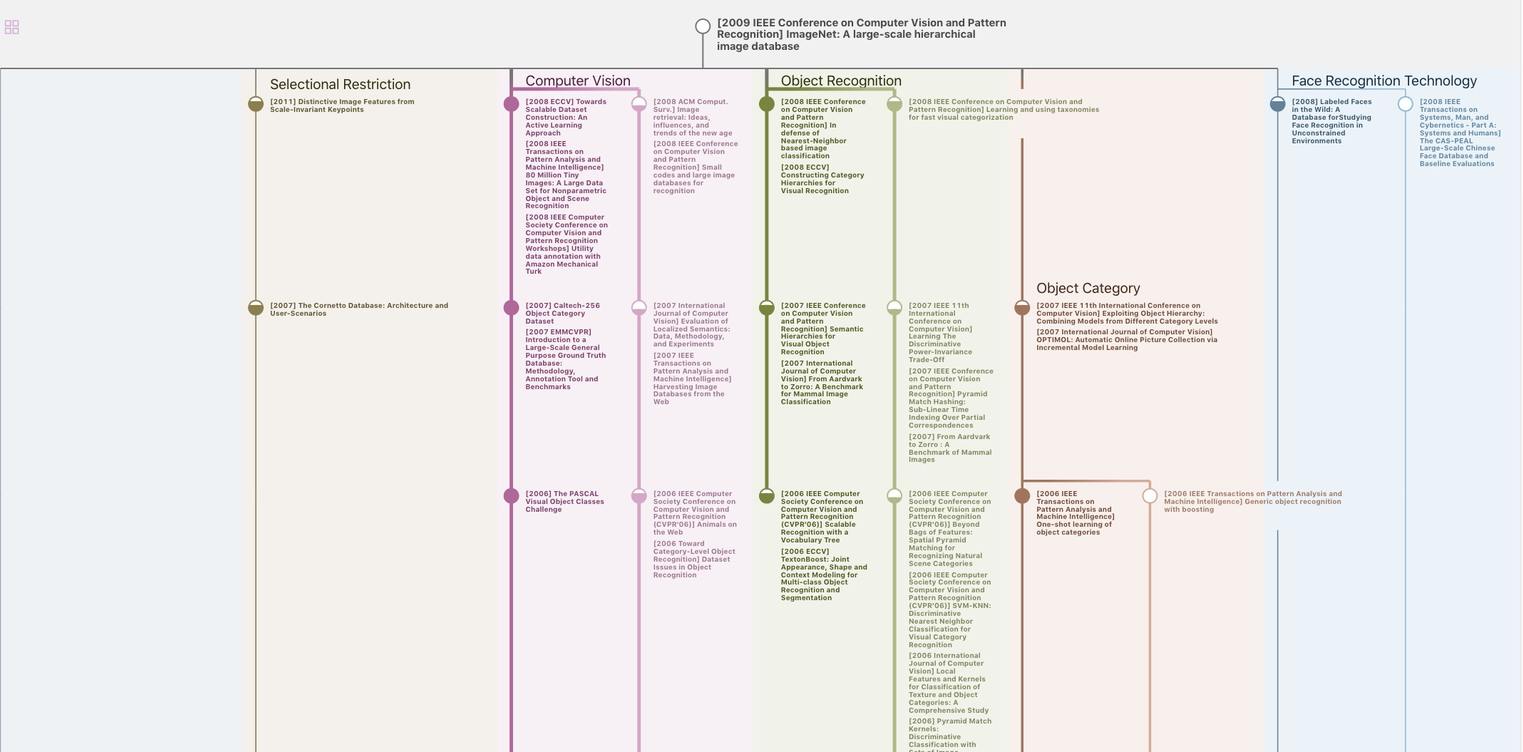
生成溯源树,研究论文发展脉络
Chat Paper
正在生成论文摘要