Low dimensional knowledge graph embeddings via hyperbolic rotations
Graph Representation Learning NeurIPS 2019 Workshop(2019)
摘要
Knowledge graphs (KGs) capture rich relationships between a large number of entities. Embeddings of these structures must preserve these relationships with high fidelity. Recently, hyperbolic embedding methods have achieved state-ofthe-art quality in graph representation learning tasks; when embedding certain graphs, they can produce parsimonious embeddings that have higher fidelity while using much fewer dimensions than their Euclidean counterparts. Mirroring work in Euclidean space, we are the first to leverage trainable hyperbolic rotations, a key notion in providing sufficiently rich representations for the complex logical patterns found in KGs. Coupled with trainable curvature, this approach yields improved embeddings in fewer dimensions. We evaluate our method, ROTATIONH, on the WN18RR link prediction task; in the low-dimensional setting, we improve on previous Euclidean-based efforts by 2.2% in mean reciprocal rank (MRR), and in the high-dimensional setting, we achieve a new state-of-the-art MRR of 49.2%, improving on existing methods by 1.1%.
更多查看译文
AI 理解论文
溯源树
样例
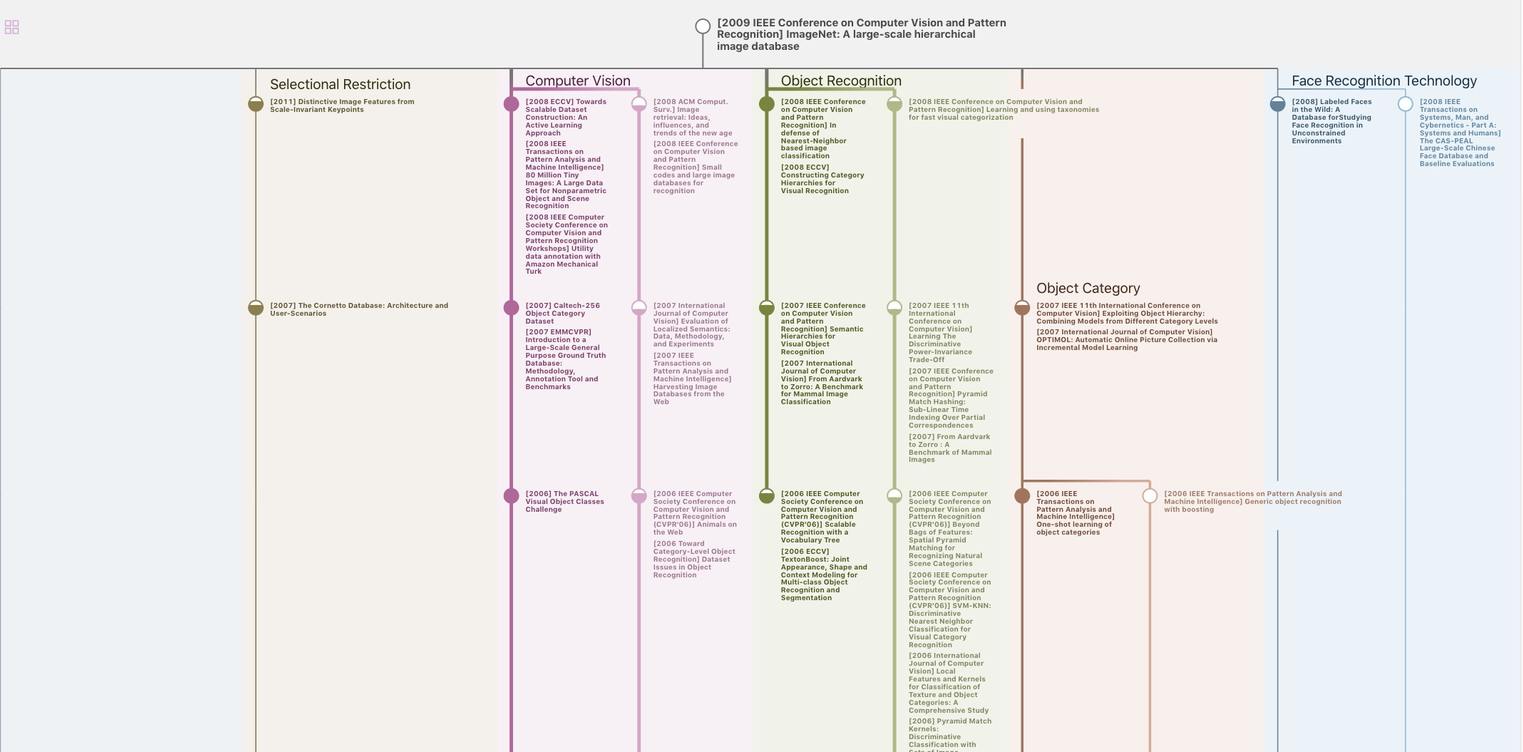
生成溯源树,研究论文发展脉络
Chat Paper
正在生成论文摘要