AQuaMuSe: Automatically Generating Datasets for Query-Based Multi-Document Summarization
arxiv(2020)
摘要
Summarization is the task of compressing source document(s) into coherent and succinct passages. This is a valuable tool to present users with concise and accurate sketch of the top ranked documents related to their queries. Query-based multi-document summarization (qMDS) addresses this pervasive need, but the research is severely limited due to lack of training and evaluation datasets as existing single-document and multi-document summarization datasets are inadequate in form and scale. We propose a scalable approach called AQuaMuSe to automatically mine qMDS examples from question answering datasets and large document corpora. Our approach is unique in the sense that it can general a dual dataset -- for extractive and abstractive summaries both. We publicly release a specific instance of an AQuaMuSe dataset with 5,519 query-based summaries, each associated with an average of 6 input documents selected from an index of 355M documents from Common Crawl. Extensive evaluation of the dataset along with baseline summarization model experiments are provided.
更多查看译文
关键词
datasets,query-based,multi-document
AI 理解论文
溯源树
样例
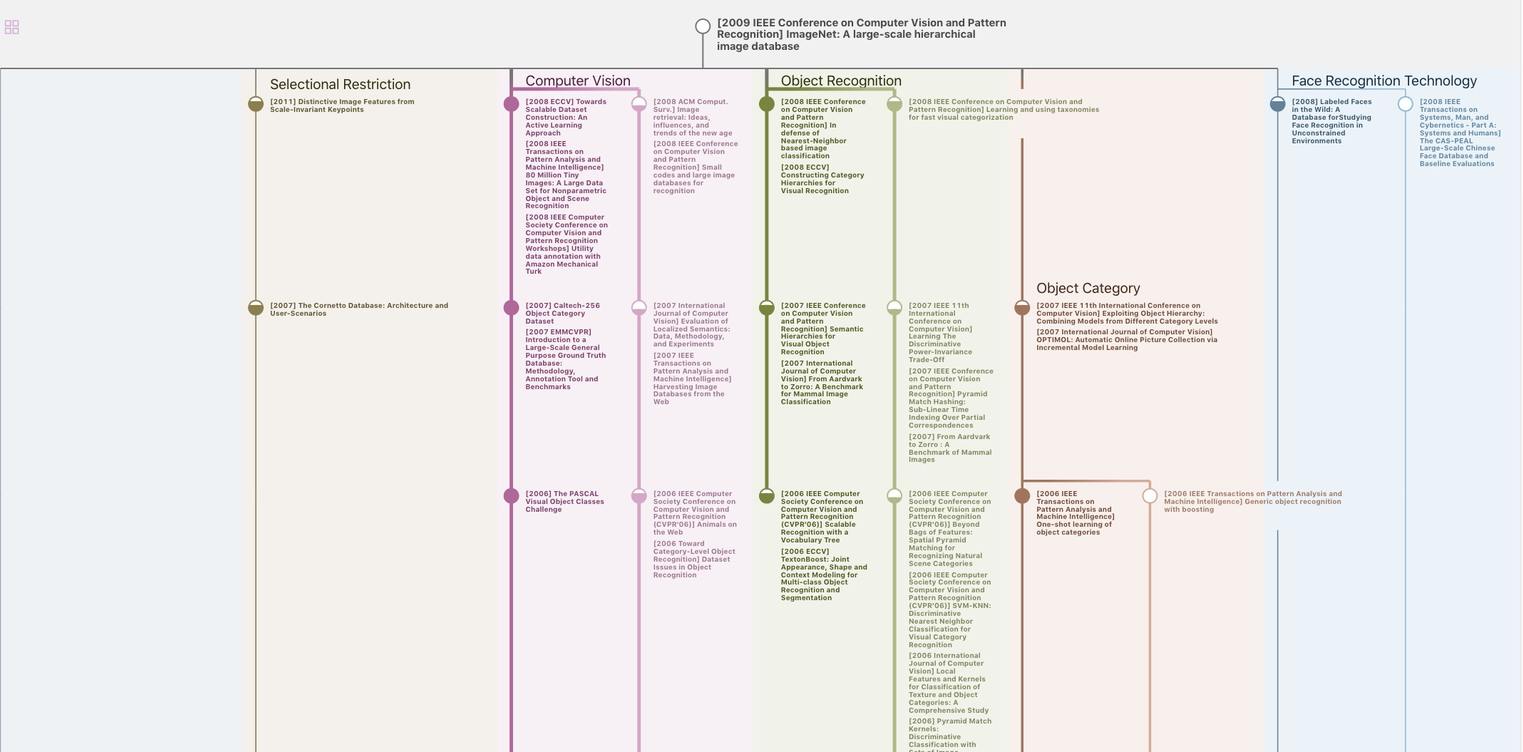
生成溯源树,研究论文发展脉络
Chat Paper
正在生成论文摘要