Stabilizing Transformer-Based Action Sequence Generation For Q-Learning
CoRR(2020)
摘要
Since the publication of the original Transformer architecture (Vaswani et al. 2017), Transformers revolutionized the field of Natural Language Processing. This, mainly due to their ability to understand timely dependencies better than competing RNN-based architectures. Surprisingly, this architecture change does not affect the field of Reinforcement Learning (RL), even though RNNs are quite popular in RL, and time dependencies are very common in RL. Recently, (Parisotto et al. 2019) conducted the first promising research of Transformers in RL. To support the findings of this work, this paper seeks to provide an additional example of a Transformer-based RL method. Specifically, the goal is a simple Transformer-based Deep Q-Learning method that is stable over several environments. Due to the unstable nature of Transformers and RL, an extensive method search was conducted to arrive at a final method that leverages developments around Transformers as well as Q-learning. The proposed method can match the performance of classic Q-learning on control environments while showing potential on some selected Atari benchmarks. Furthermore, it was critically evaluated to give additional insights into the relation between Transformers and RL.
更多查看译文
关键词
action sequence generation,transformer-based,q-learning
AI 理解论文
溯源树
样例
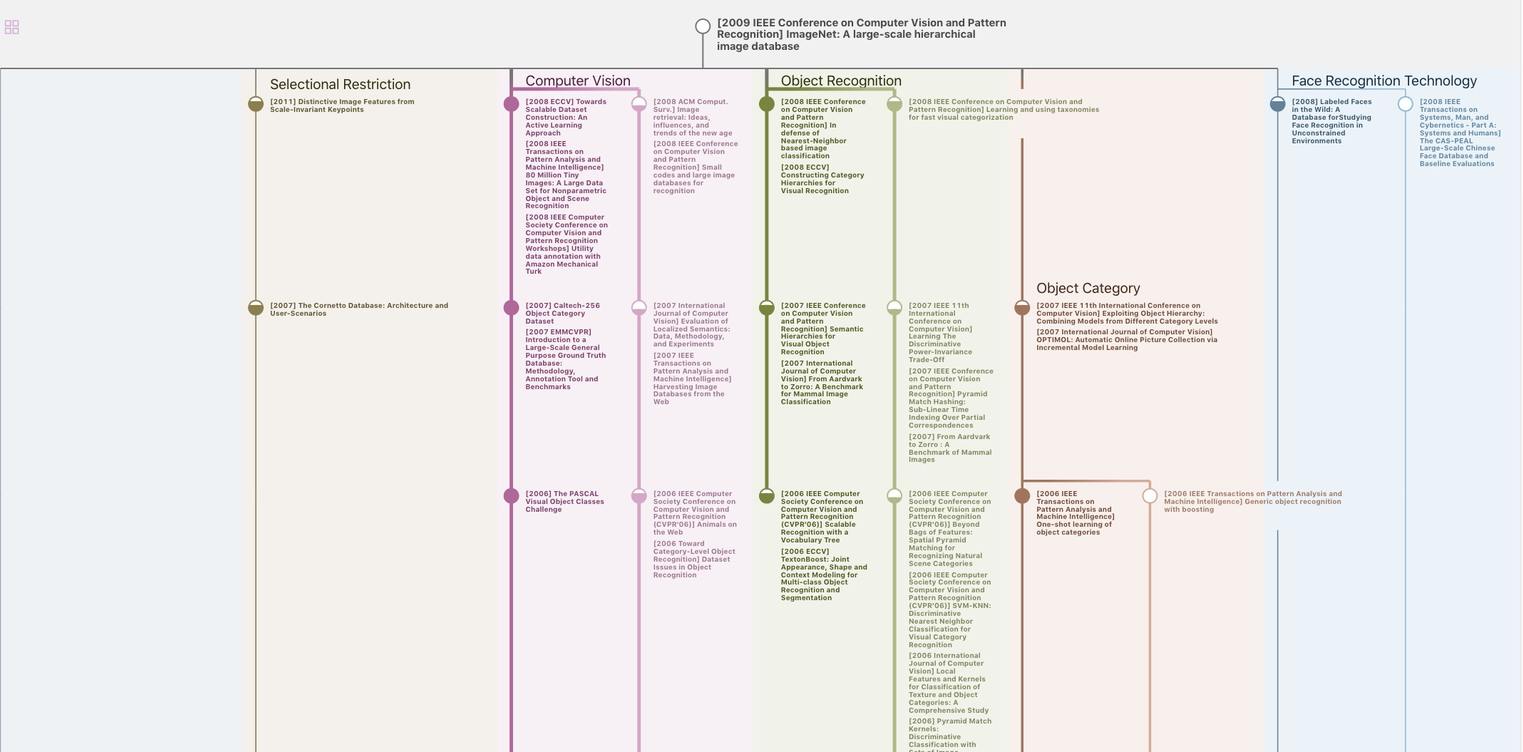
生成溯源树,研究论文发展脉络
Chat Paper
正在生成论文摘要