An Effective Sinogram Inpainting For Complementary Limited-Angle Dual-Energy Computed Tomography Imaging Using Generative Adversarial Networks
JOURNAL OF X-RAY SCIENCE AND TECHNOLOGY(2021)
摘要
Dual-energy computed tomography (DECT) provides more anatomical and functional information for image diagnosis. Presently, the popular DECT imaging systems need to scan at least full angle (i.e., 360 degrees). In this study, we propose a DECT using complementary limited-angle scan (DECT-CL) technology to reduce the radiation dose and compress the spatial distribution of the imaging system. The dual-energy total scan is 180 degrees, where the low- and high-energy scan range is the first 90 degrees and last 90 degrees, respectively. We describe this dual limited-angle problem as a complementary limited-angle problem, which is challenging to obtain high-quality images using traditional reconstruction algorithms. Furthermore, a complementary-sinogram-inpainting generative adversarial networks (CSI-GAN) with a sinogram loss is proposed to inpainting sinogram to suppress the singularity of truncated sinogram. The sinogram loss focuses on the data distribution of the generated sinogram while approaching the target sinogram. We use the simultaneous algebraic reconstruction technique namely, a total variable (SART-TV) algorithms for image reconstruction. Then, taking reconstructed CT images of pleural and cranial cavity slices as examples, we evaluate the performance of our method and numerically compare different methods based on root mean square error (RMSE), peak signal-to-noise ratio (PSNR) and structural similarity (SSIM). Compared with traditional algorithms, the proposed network shows advantages in numerical terms. Compared with Patch-GAN, the proposed network can also reduce the RMSE of the reconstruction results by an average of 40% and increase the PSNR by an average of 26%. In conclusion, both qualitative and quantitative comparison and analysis demonstrate that our proposed method achieves a good artifact suppression effect and can suitably solve the complementary limited-angle problem.
更多查看译文
关键词
Dual-energy computed tomography, complementary limited angle problem, generative adversarial networks
AI 理解论文
溯源树
样例
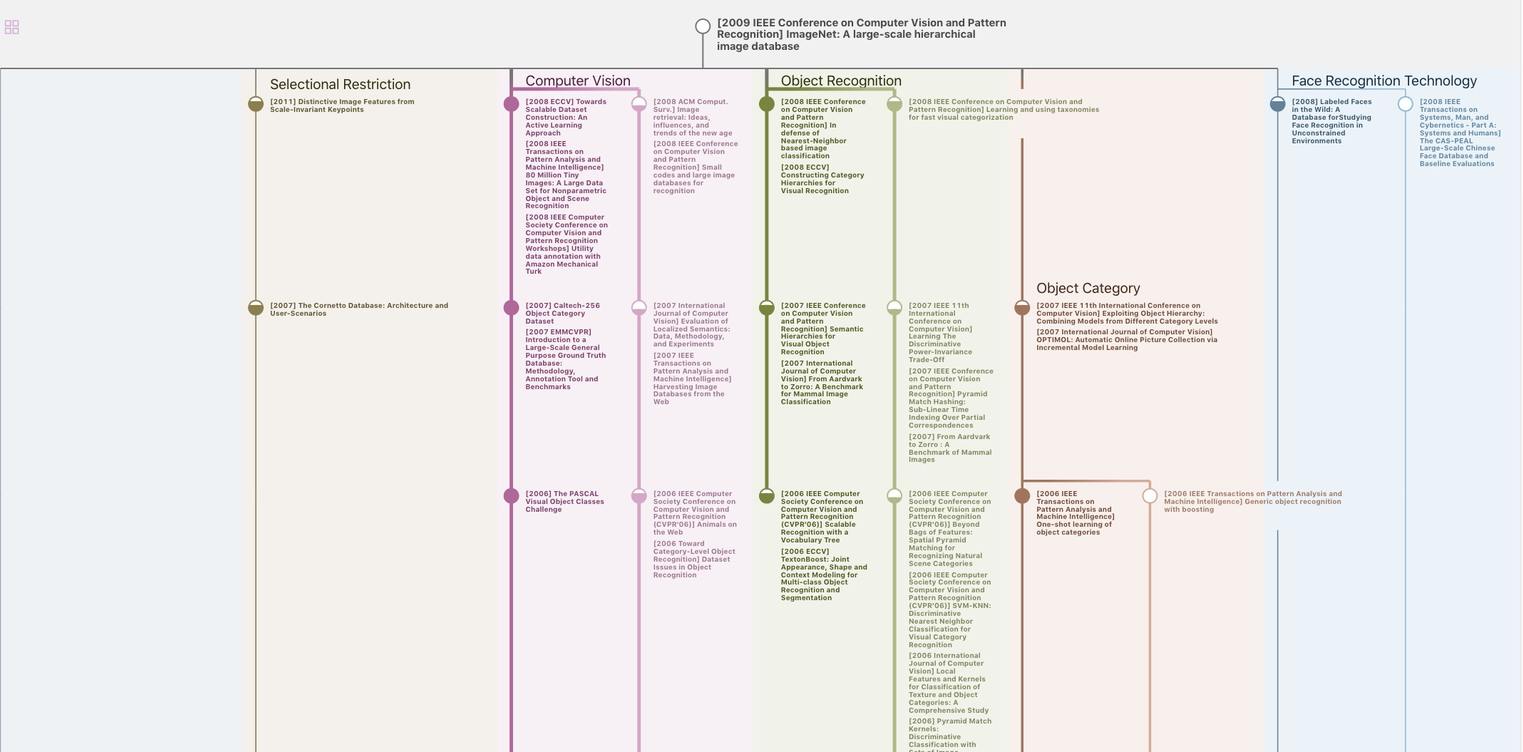
生成溯源树,研究论文发展脉络
Chat Paper
正在生成论文摘要