Machine Learning aided 3D-position reconstruction in large LaCl$_{3}$ crystals
arxiv(2020)
摘要
We investigate five different models to reconstruct the 3D $\gamma$-ray hit coordinates in five large \lacls monolithic crystals optically coupled to pixelated silicon photomultipliers. These scintillators have a base surface of 50 $\times$ 50 mm$^2$ and five different thicknesses, from 10 mm to 30 mm. Four of these models are analytical prescriptions and one is based on a Convolutional Neural Network. Average resolutions close to 1-2mm fwhm are obtained in the transverse crystal plane for crystal thicknesses between 10 mm and 20 mm using analytical models. For thicker crystals average resolutions of about 3-5~mm fwhm are obtained. Depth of interaction resolutions between 1mm and 4 mm are achieved depending on the distance of the interaction point to the photosensor surface. We propose a Machine Learning algorithm to correct for linearity distortions and pin-cushion effects. The latter allows one to keep a large field of view of about 70-80\% of the crystal surface, regardless of crystal thickness. This work is aimed at optimizing the performance of the so-called Total Energy Detector with Compton imaging capability (i-TED) for time-of-flight neutron capture cross-section measurements.
更多查看译文
关键词
Gamma-ray,Position sensitive detectors,Monolithic crystals,Compton imaging,Machine Learning,Convolutional Neural Networks,Total Energy Detector,Neutron capture cross-section
AI 理解论文
溯源树
样例
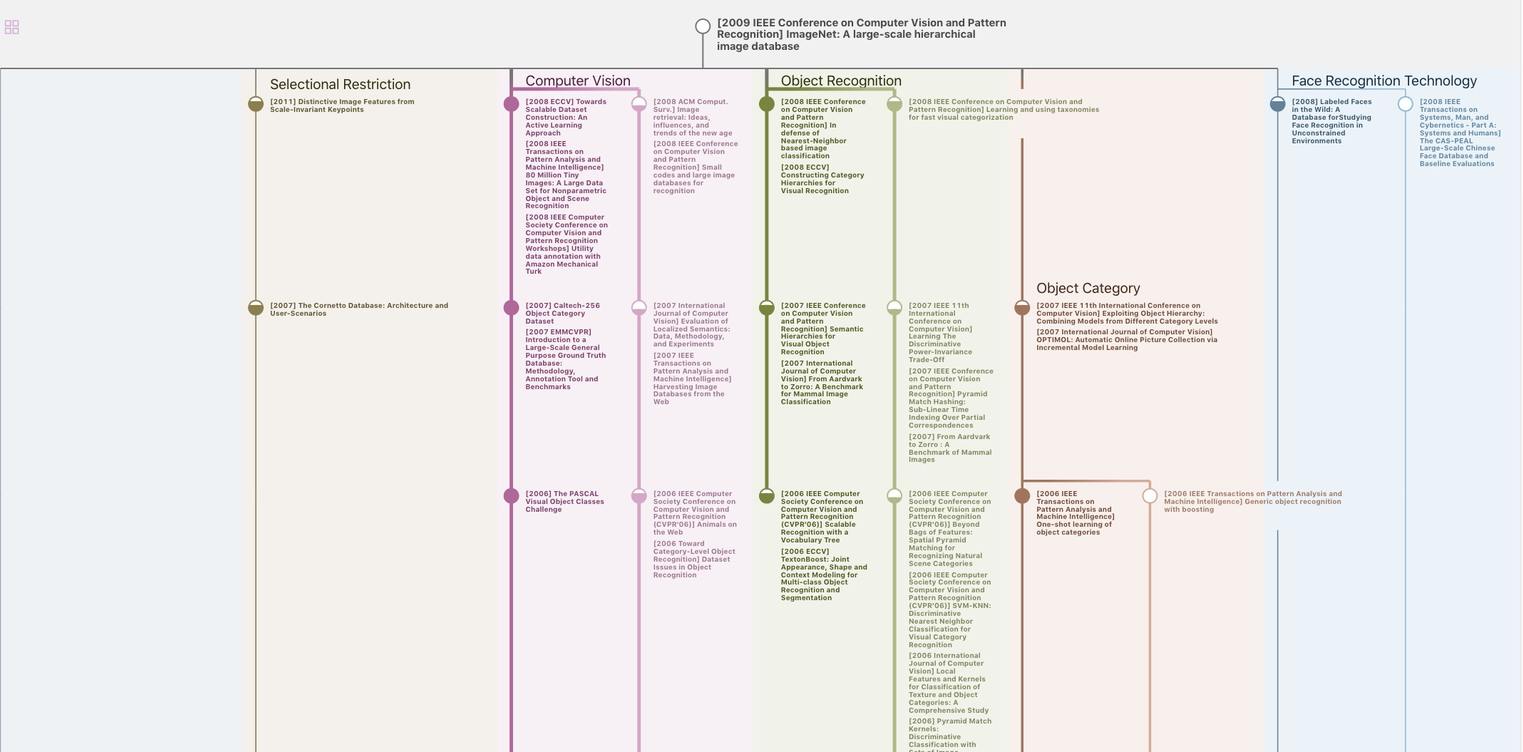
生成溯源树,研究论文发展脉络
Chat Paper
正在生成论文摘要