BYTECOVER: COVER SONG IDENTIFICATION VIA MULTI-LOSS TRAINING
2021 IEEE INTERNATIONAL CONFERENCE ON ACOUSTICS, SPEECH AND SIGNAL PROCESSING (ICASSP 2021)(2021)
摘要
We present in this paper ByteCover, which is a new feature learning method for cover song identification (CSI). ByteCover is built based on the classical ResNet model, and two major improvements are designed to further enhance the capability of the model for CSI. In the first improvement, we introduce the integration of instance normalization (IN) and batch normalization (BN) to build IBN blocks, which are major components of our ResNet-IBN model. With the help of the IBN blocks, our CSI model can learn features that are invariant to the changes of musical attributes such as key, tempo, timbre and genre, while preserving the version information. In the second improvement, we employ the BN-Neck method to allow a multi-loss training and encourage our method to jointly optimize a classification loss and a triplet loss, and by this means, the inter-class discrimination and intra-class compactness of cover songs, can be ensured at the same time. A set of experiments demonstrated the effectiveness and efficiency of ByteCover on multiple datasets, and in the Da-TACOS dataset, ByteCover outperformed the best competitive system by 18.0%.
更多查看译文
关键词
Cover song identification, instance-batch normalization (IBN), BNNeck, classification loss, triplet loss
AI 理解论文
溯源树
样例
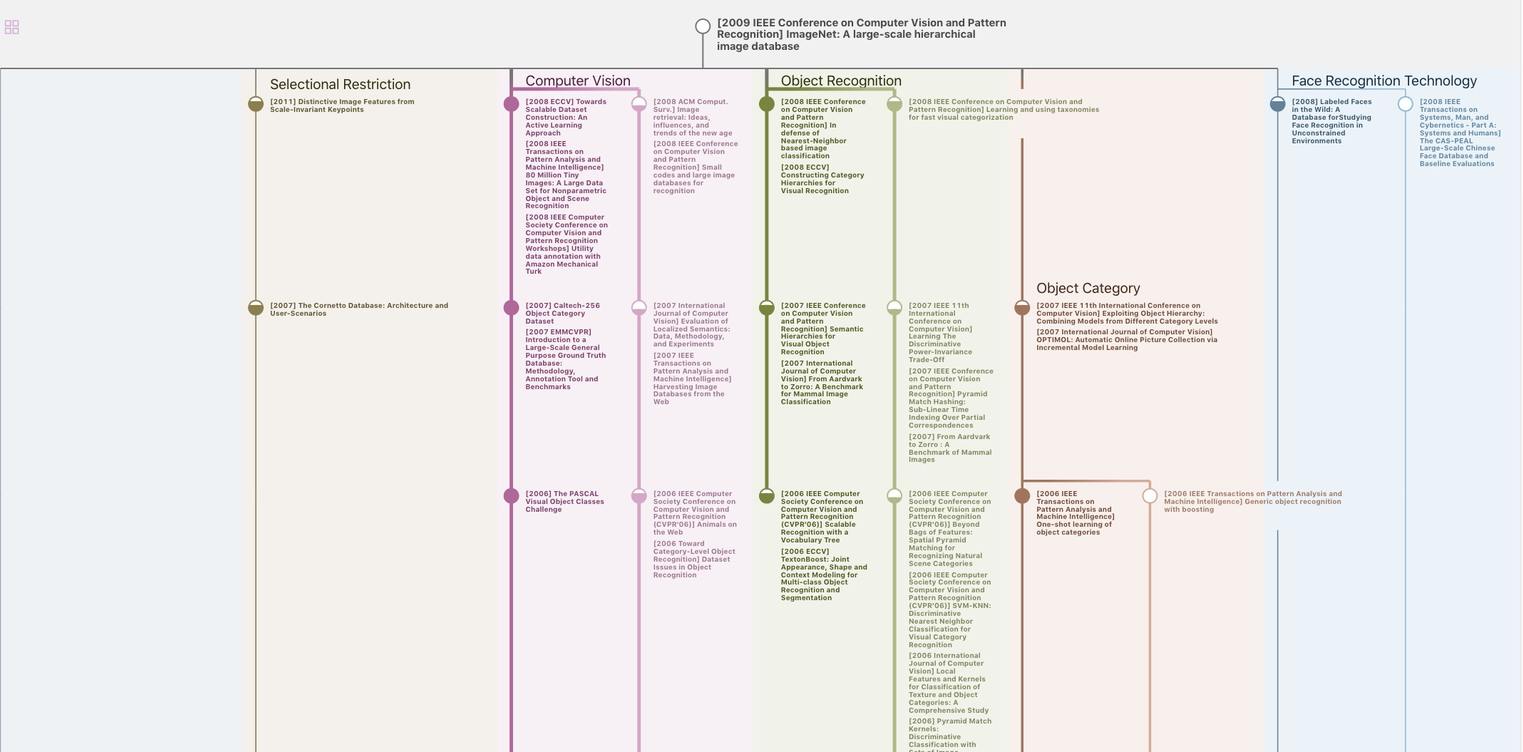
生成溯源树,研究论文发展脉络
Chat Paper
正在生成论文摘要