The Teaching Dimension Of Kernel Perceptron
24TH INTERNATIONAL CONFERENCE ON ARTIFICIAL INTELLIGENCE AND STATISTICS (AISTATS)(2021)
摘要
Algorithmic machine teaching has been studied under the linear setting where exact teaching is possible. However, little is known for teaching nonlinear learners. Here, we establish the sample complexity of teaching, aka teaching dimension, for kernelized perceptrons for different families of feature maps. As a warm-up, we show that the teaching complexity is Theta(d) for the exact teaching of linear perceptrons in R-d, and Theta(d(k)) for kernel perceptron with a polynomial kernel of order k. Furthermore, under certain smooth assumptions on the data distribution, we establish a rigorous bound on the complexity for approximately teaching a Gaussian kernel perceptron. We provide numerical examples of the optimal (approximate) teaching set under several canonical settings for linear, polynomial and Gaussian kernel perceptrons.
更多查看译文
关键词
kernel perceptron,teaching dimension
AI 理解论文
溯源树
样例
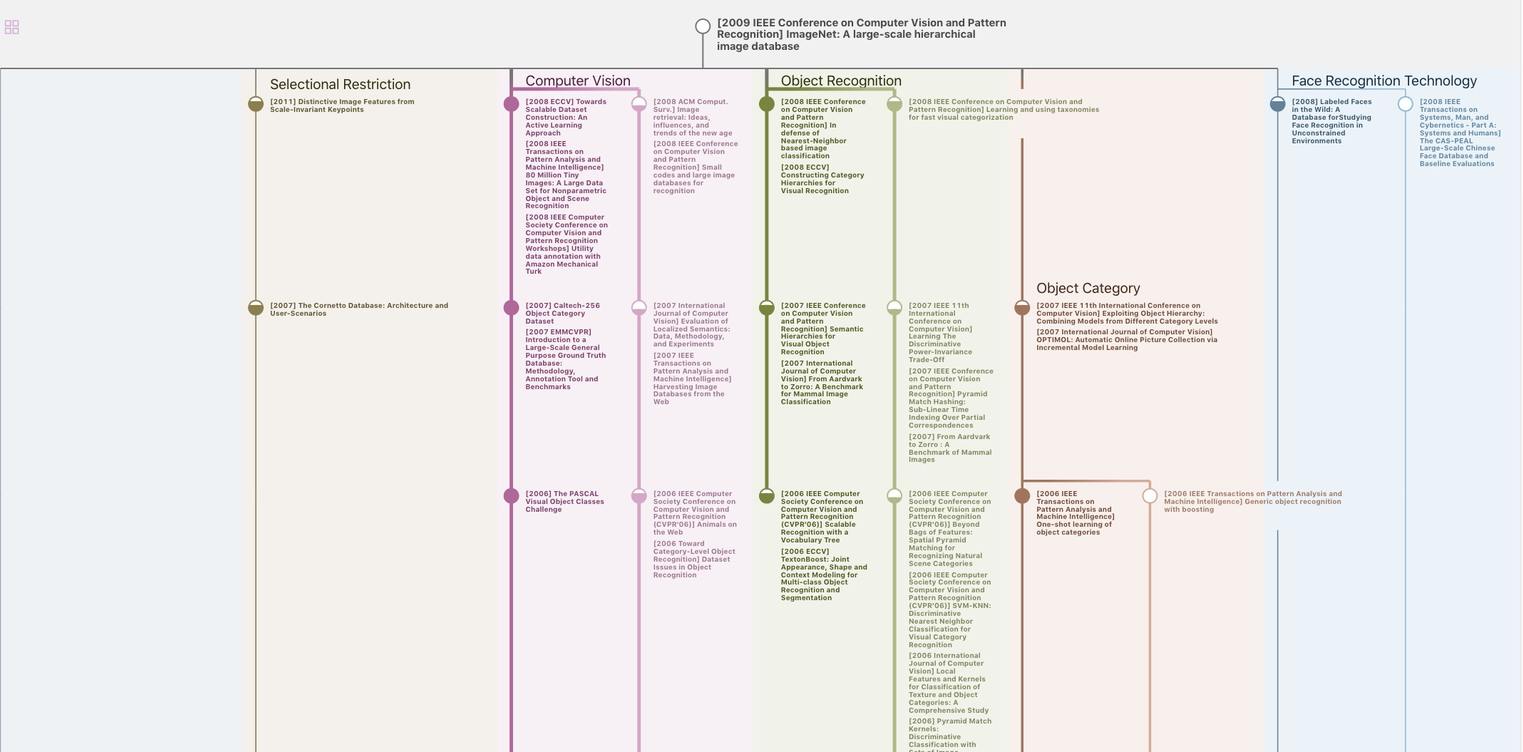
生成溯源树,研究论文发展脉络
Chat Paper
正在生成论文摘要