Preclinical validation of therapeutic targets predicted by tensor factorization on heterogeneous graphs
SCIENTIFIC REPORTS(2020)
摘要
Incorrect drug target identification is a major obstacle in drug discovery. Only 15% of drugs advance from Phase II to approval, with ineffective targets accounting for over 50% of these failures 1 – 3 . Advances in data fusion and computational modeling have independently progressed towards addressing this issue. Here, we capitalize on both these approaches with Rosalind, a comprehensive gene prioritization method that combines heterogeneous knowledge graph construction with relational inference via tensor factorization to accurately predict disease-gene links. Rosalind demonstrates an increase in performance of 18%-50% over five comparable state-of-the-art algorithms. On historical data, Rosalind prospectively identifies 1 in 4 therapeutic relationships eventually proven true. Beyond efficacy, Rosalind is able to accurately predict clinical trial successes (75% recall at rank 200) and distinguish likely failures (74% recall at rank 200). Lastly, Rosalind predictions were experimentally tested in a patient-derived in-vitro assay for Rheumatoid arthritis (RA), which yielded 5 promising genes, one of which is unexplored in RA.
更多查看译文
关键词
Data integration,Machine learning,Target identification,Science,Humanities and Social Sciences,multidisciplinary
AI 理解论文
溯源树
样例
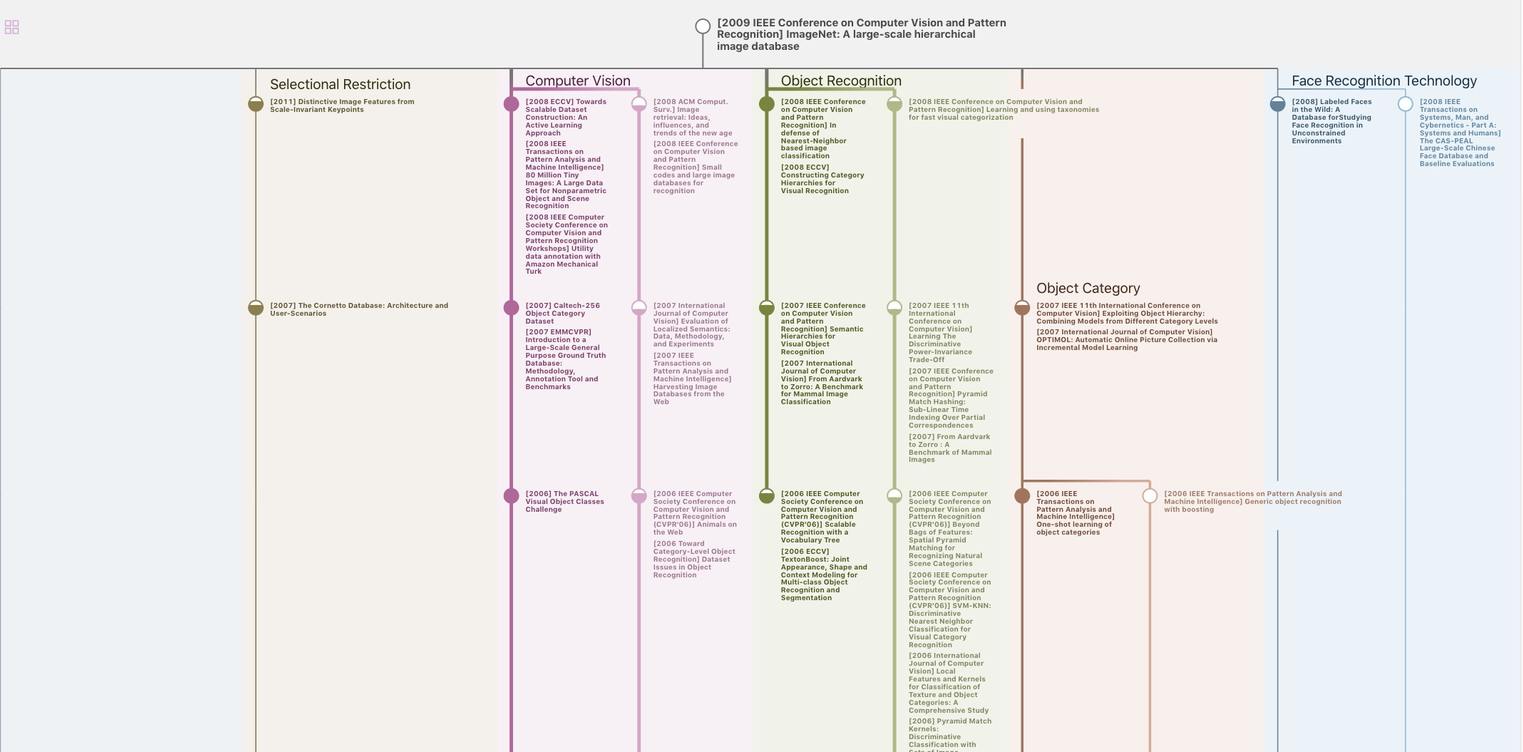
生成溯源树,研究论文发展脉络
Chat Paper
正在生成论文摘要