LEARNING CONTEXTUAL TAG EMBEDDINGS FOR CROSS-MODAL ALIGNMENT OF AUDIO AND TAGS
2021 IEEE INTERNATIONAL CONFERENCE ON ACOUSTICS, SPEECH AND SIGNAL PROCESSING (ICASSP 2021)(2021)
摘要
Self-supervised audio representation learning offers an attractive alternative for obtaining generic audio embeddings, capable to be employed into various downstream tasks. Published approaches that consider both audio and words/tags associated with audio do not employ text processing models that are capable to generalize to tags unknown during training. In this work we propose a method for learning audio representations using an audio autoencoder (AAE), a general word embeddings model (WEM), and a multi-head self-attention (MHA) mechanism. MHA attends on the output of the WEM, providing a contextualized representation of the tags associated with the audio, and we align the output of MHA with the output of the encoder of AAE using a contrastive loss. We jointly optimize AAE and MHA and we evaluate the audio representations (i.e. the output of the encoder of AAE) by utilizing them in three different downstream tasks, namely sound, music genre, and music instrument classification. Our results show that employing multi-head self-attention with multiple heads in the tag-based network can induce better learned audio representations.
更多查看译文
关键词
representation learning, multimodal contrastive learning, audio classification
AI 理解论文
溯源树
样例
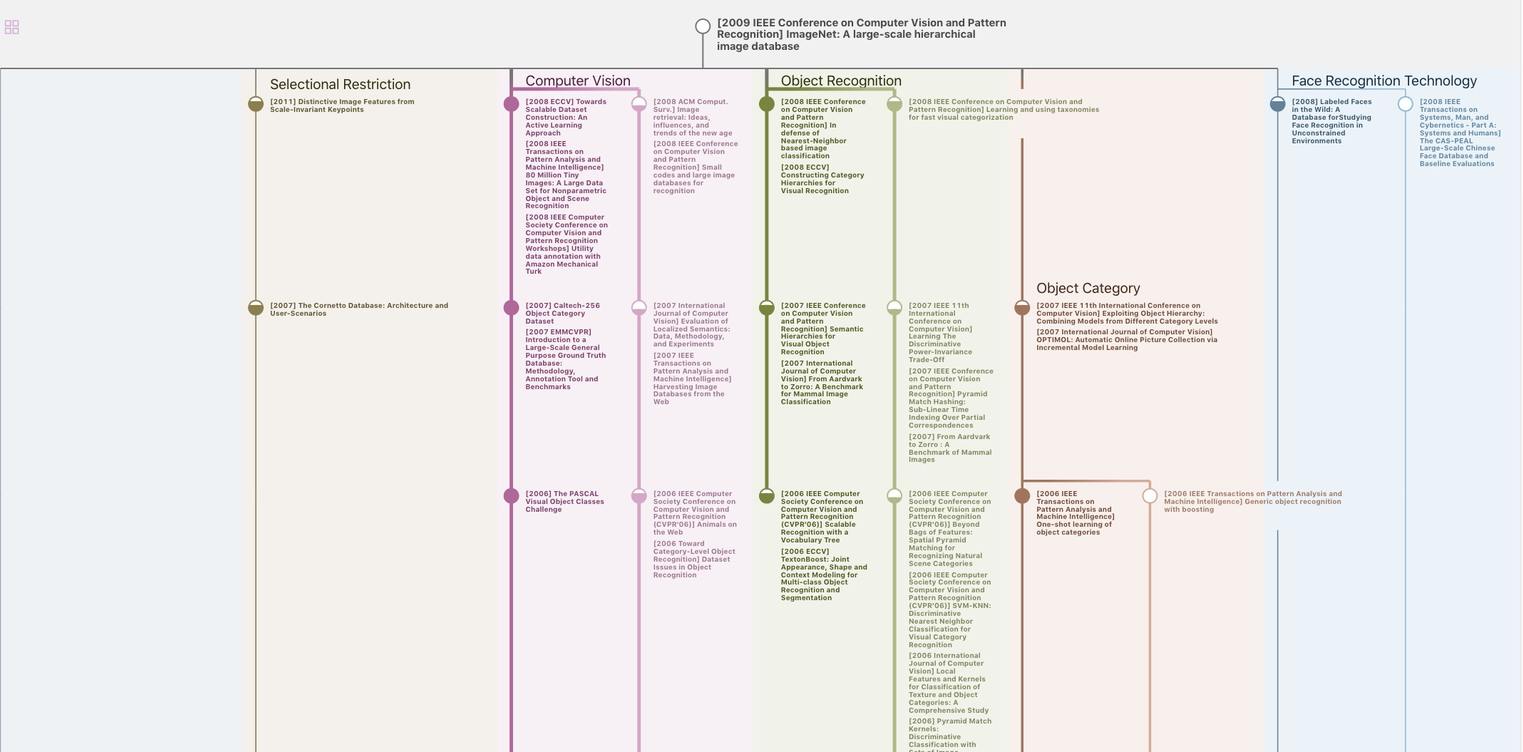
生成溯源树,研究论文发展脉络
Chat Paper
正在生成论文摘要