Are Few Shot Learning Benchmarks too Simple? Solving them without Task Supervision at Test Time
arXiv preprint arXiv:1902.08605(2019)
摘要
We argue that the widely used Omniglot and miniImageNet benchmarks are too simple because their* class semantics* do not vary across episodes, which defeats their intended purpose of evaluating few-shot classification methods. The class semantics of Omniglot is invariably" characters" and the class semantics of miniImageNet," object category". Because the class semantics are so similar, we propose a new method called Centroid Networks which can achieve surprisingly high accuracies on Omniglot and miniImageNet without using any labels at meta-evaluation time. Our results suggest that those benchmarks are not adapted for supervised few-shot classification since the supervision itself is not necessary during meta-evaluation. The Meta-Dataset, a collection of 10 datasets, was recently proposed as a harder few-shot classification benchmark. Using our method, we derive a new metric, the Class Semantics Consistency Criterion, and use it to quantify the difficulty of Meta-Dataset. Finally, under some restrictive assumptions, we show that Centroid Networks is faster and more accurate than a state-of-the-art learning-to-cluster method (Hsu et al 2018).
更多查看译文
AI 理解论文
溯源树
样例
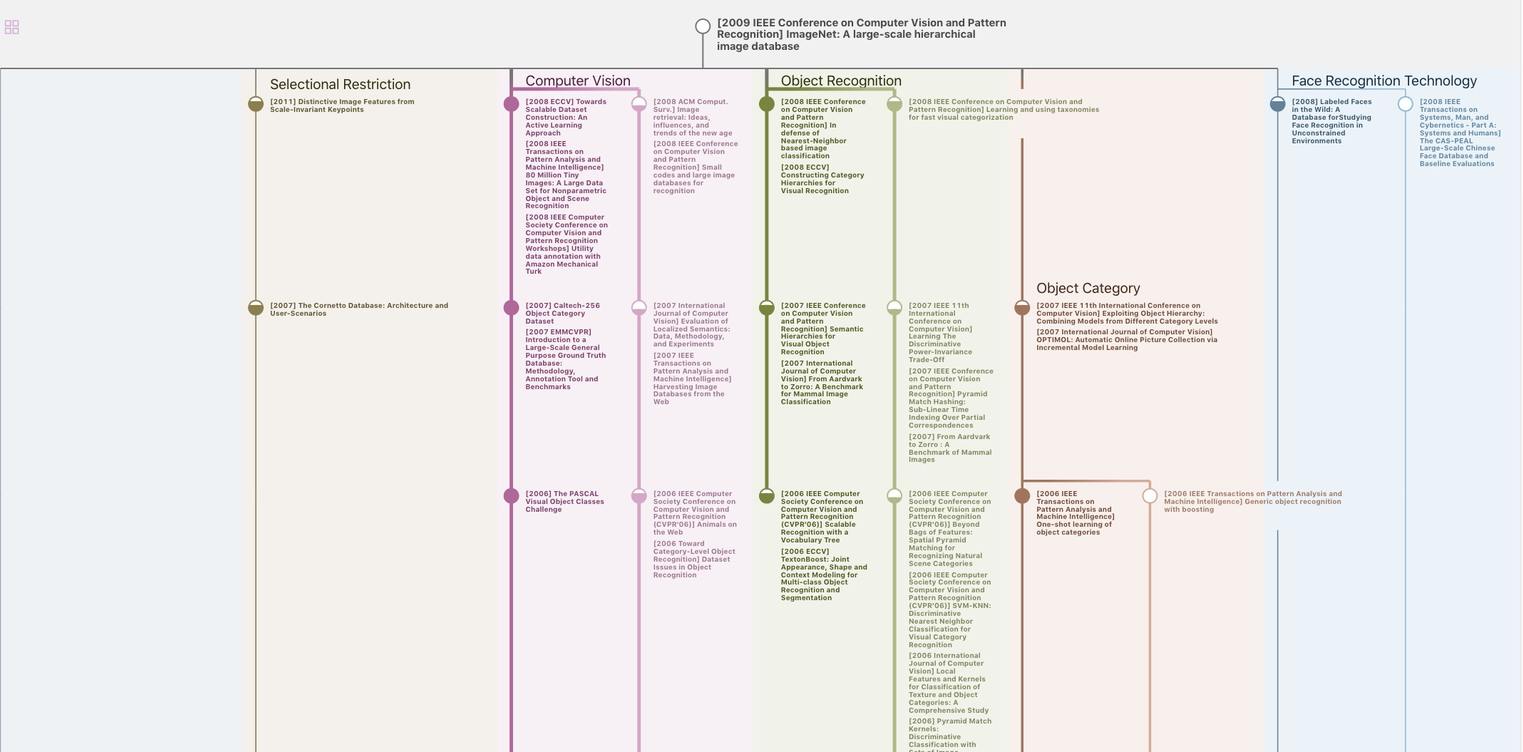
生成溯源树,研究论文发展脉络
Chat Paper
正在生成论文摘要