On the diminishing return of labeling clinical reports
ClinicalNLP@EMNLP(2020)
摘要
Ample evidence suggests that better machine learning models may be steadily obtained by training on increasingly larger datasets on natural language processing (NLP) problems from non-medical domains. Whether the same holds true for medical NLP has by far not been thoroughly investigated. This work shows that this is indeed not always the case. We reveal the somehow counter-intuitive observation that performant medical NLP models may be obtained with small amount of labeled data, quite the opposite to the common belief, most likely due to the domain specificity of the problem. We show quantitatively the effect of training data size on a fixed test set composed of two of the largest public chest x-ray radiology report datasets on the task of abnormality classification. The trained models not only make use of the training data efficiently, but also outperform the current state-of-the-art rule-based systems by a significant margin.
更多查看译文
关键词
labeling,clinical,reports
AI 理解论文
溯源树
样例
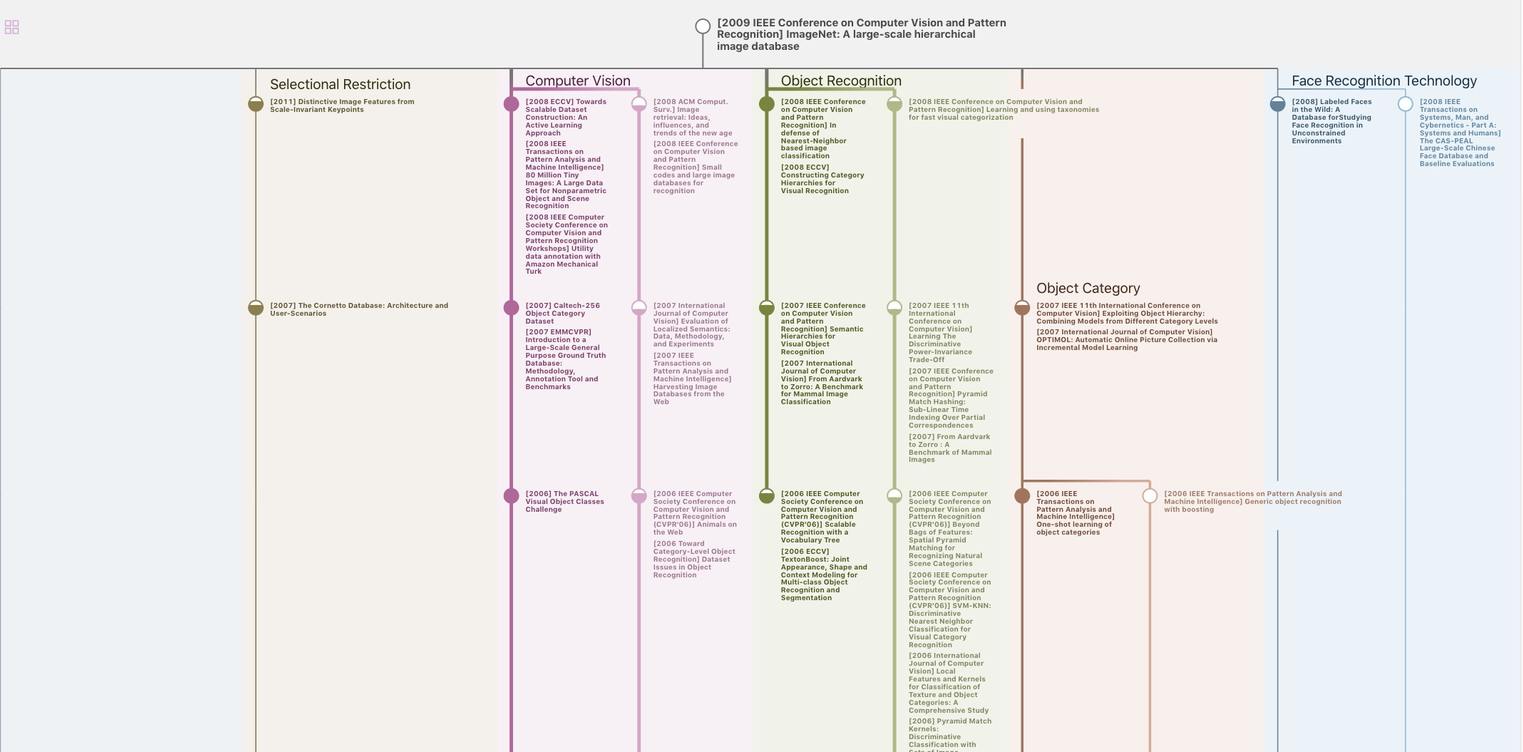
生成溯源树,研究论文发展脉络
Chat Paper
正在生成论文摘要