Machine Learning Regularized Solution of the Lippmann-Schwinger Equation
arxiv(2020)
摘要
Solution of the discretized Lippmann-Schwinger equation in the spatial frequency domain involves the inversion of a linear operator specified by the scattering potential. To regularize this inevitably ill-conditioned problem, we propose a machine learning approach: a recurrent neural network with long short-term memory (LSTM) and with the null space projection of the Lippmann-Schwinger kernel on the recurrence path. The learning method is trained using examples of typical scattering potentials and their corresponding scattered fields. We test the proposed method in two cases: electromagnetic scattering by dielectric objects, and electron scattering by multiple screened Coulomb potentials. In both cases the solutions to test examples, disjoint from the training set, were obtained with fewer iterations and were accurate compared to linear solvers. We also observed surprising generalization ability: in the electromagnetic case, an LSTM trained with random arrangements of dielectric spheres was able to obtain the correct solutions for general topologically similar objects, such as polygons. This suggests that the LSTM successfully incorporates the physics of scattering into the inversion algorithm.
更多查看译文
关键词
machine learning,lippmann-schwinger
AI 理解论文
溯源树
样例
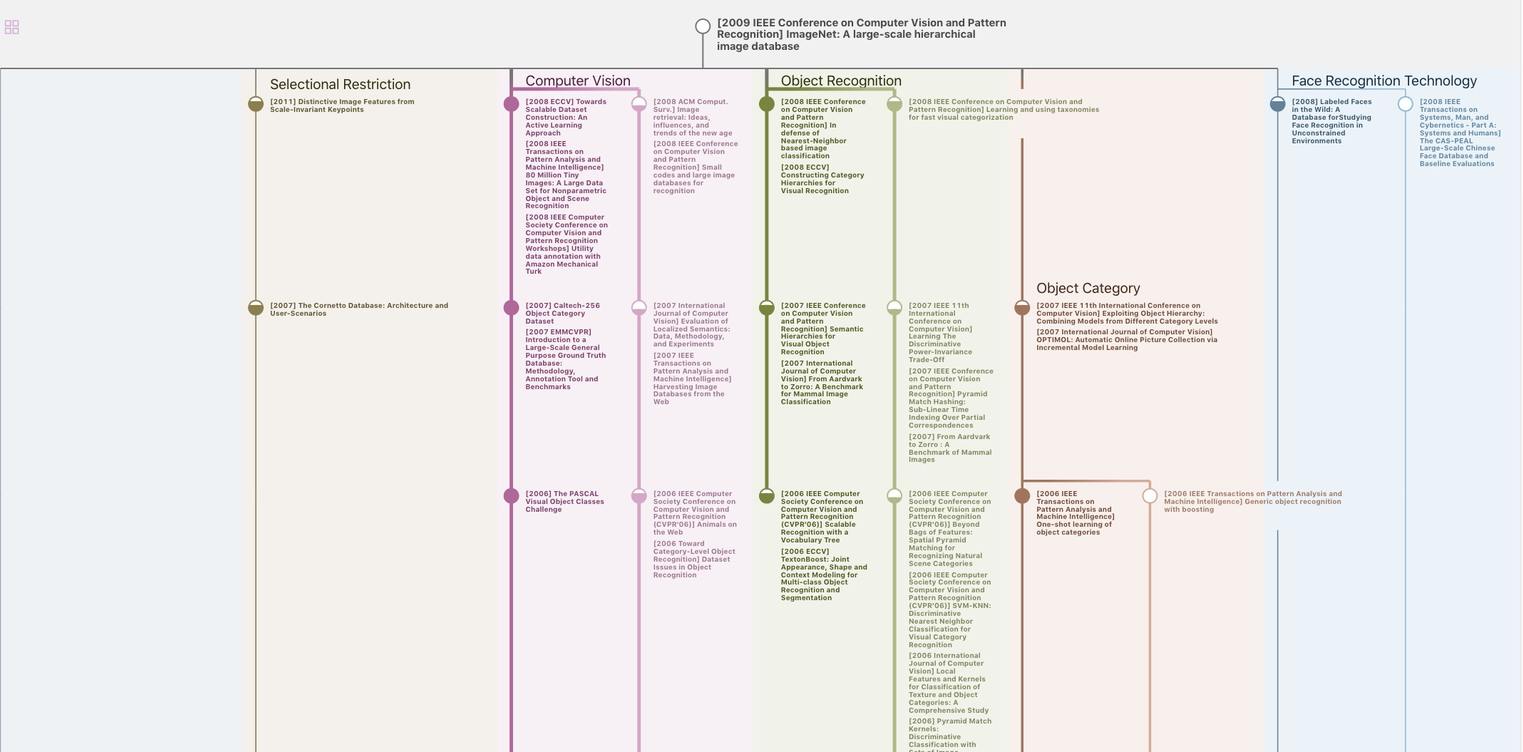
生成溯源树,研究论文发展脉络
Chat Paper
正在生成论文摘要