FIGLEARN: FILTER AND GRAPH LEARNING USING OPTIMAL TRANSPORT
2021 IEEE INTERNATIONAL CONFERENCE ON ACOUSTICS, SPEECH AND SIGNAL PROCESSING (ICASSP 2021)(2021)
摘要
In many applications, a dataset can be considered as a set of observed signals that live on an unknown underlying graph structure. Some of these signals may be seen as white noise that has been filtered on the graph topology by a graph filter. Hence, the knowledge of the filter and the graph provides valuable information about the underlying data generation process and the complex interactions that arise in the dataset. We hence introduce a novel graph signal processing framework for jointly learning the graph and its generating filter from signal observations. We cast a new optimisation problem that minimises the Wasserstein distance between the distribution of the signal observations and the filtered signal distribution model. Our proposed method outperforms state-of-the-art graph learning frameworks on synthetic data. We then apply our method to a temperature anomaly dataset, and further show how this framework can be used to infer missing values if only very little information is available.
更多查看译文
关键词
GSP, graph learning, filter learning
AI 理解论文
溯源树
样例
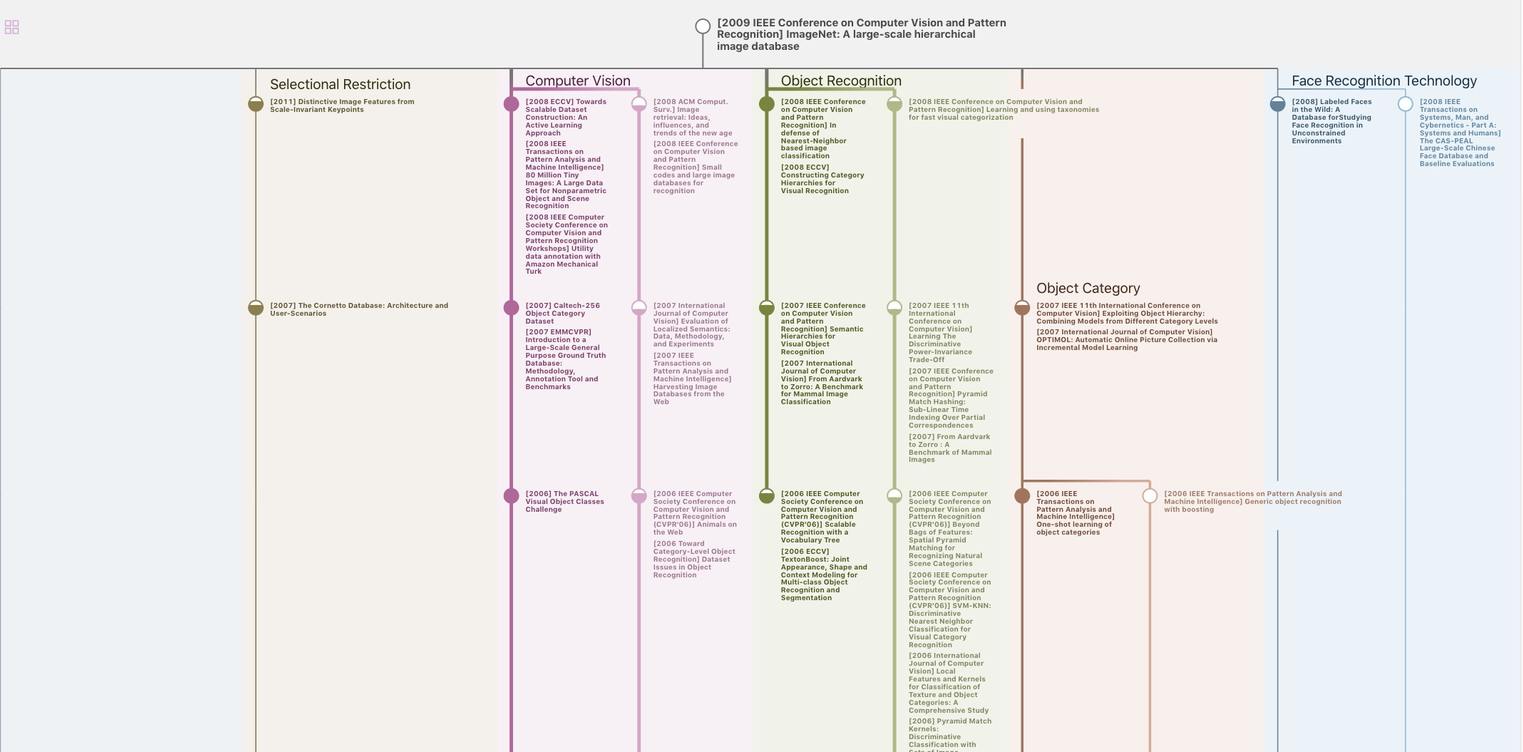
生成溯源树,研究论文发展脉络
Chat Paper
正在生成论文摘要