SEMI-SUPERVISED SPEECH RECOGNITION VIA GRAPH-BASED TEMPORAL CLASSIFICATION
2021 IEEE INTERNATIONAL CONFERENCE ON ACOUSTICS, SPEECH AND SIGNAL PROCESSING (ICASSP 2021)(2021)
摘要
Semi-supervised learning has demonstrated promising results in automatic speech recognition (ASR) by self-training using a seed ASR model with pseudo-labels generated for unlabeled data. The effectiveness of this approach largely relies on the pseudo-label accuracy, for which typically only the 1-best ASR hypothesis is used. However, alternative ASR hypotheses of an N-best list can provide more accurate labels for an unlabeled speech utterance and also reflect uncertainties of the seed ASR model. In this paper, we propose a generalized form of the connectionist temporal classification (CTC) objective that accepts a graph representation of the training labels. The newly proposed graph-based temporal classification (GTC) objective is applied for self-training with WFST-based supervision, which is generated from an N -best list of pseudo-labels. In this setup, GTC is used to learn not only a temporal alignment, similarly to CTC, but also a label alignment to obtain the optimal pseudo-label sequence from the weighted graph. Results show that this approach can effectively exploit an N -best list of pseudo-labels with associated scores, considerably outperforming standard pseudo-labeling, with ASR results approaching an oracle experiment in which the best hypotheses of the N -best lists are selected manually.
更多查看译文
关键词
graph-based temporal classification, semi-supervised ASR, pseudo-labeling, self-training, WFST
AI 理解论文
溯源树
样例
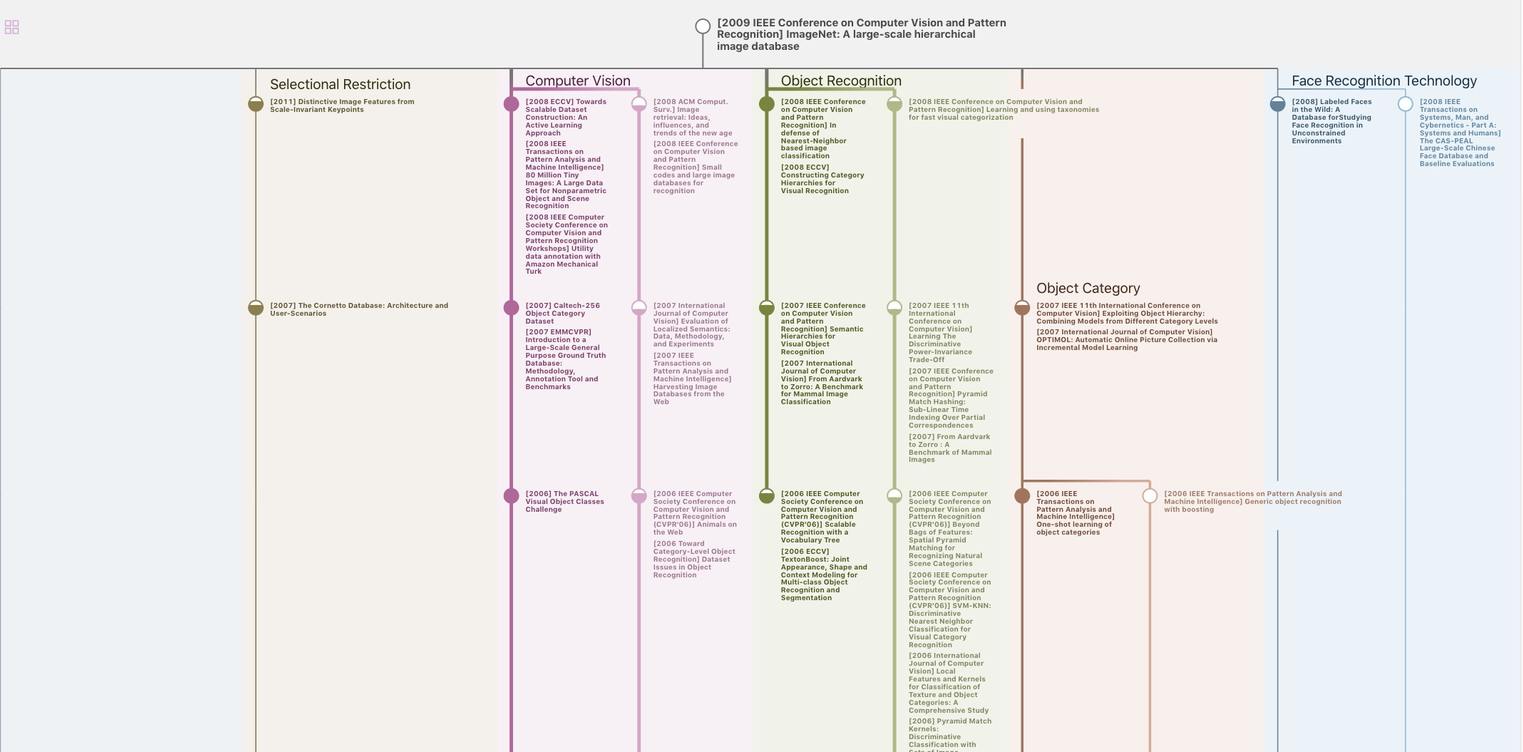
生成溯源树,研究论文发展脉络
Chat Paper
正在生成论文摘要