HDGI: An Unsupervised Graph Neural Network for Representation Learning in Heterogeneous Graph
user-5f1696ff4c775ed682f5929f(2020)
摘要
Graph representation learning is to learn universal node representations that preserve both node attributes and structural information. When a graph is heterogeneous, the problem becomes more challenging than the homogeneous graph node learning problem. Inspired by the emerging information theoretic-based learning algorithm, we propose an unsupervised graph neural network Heterogeneous Deep Graph Infomax (HDGI) for heterogeneous graph representation learning. By maximizing local-global mutual information, HDGI effectively learns high-level node representations that can be utilized in downstream graph-related tasks. Experiment results show that HDGI remarkably outperforms stateof-the-art unsupervised graph representation learning methods on both classification and clustering tasks. By feeding the learned representations into a parametric model, we even achieve comparable performance in node classification tasks when comparing with supervised end-to-end GNN models. A full version of this paper can be accessed in (Ren et al. 2019).
更多查看译文
AI 理解论文
溯源树
样例
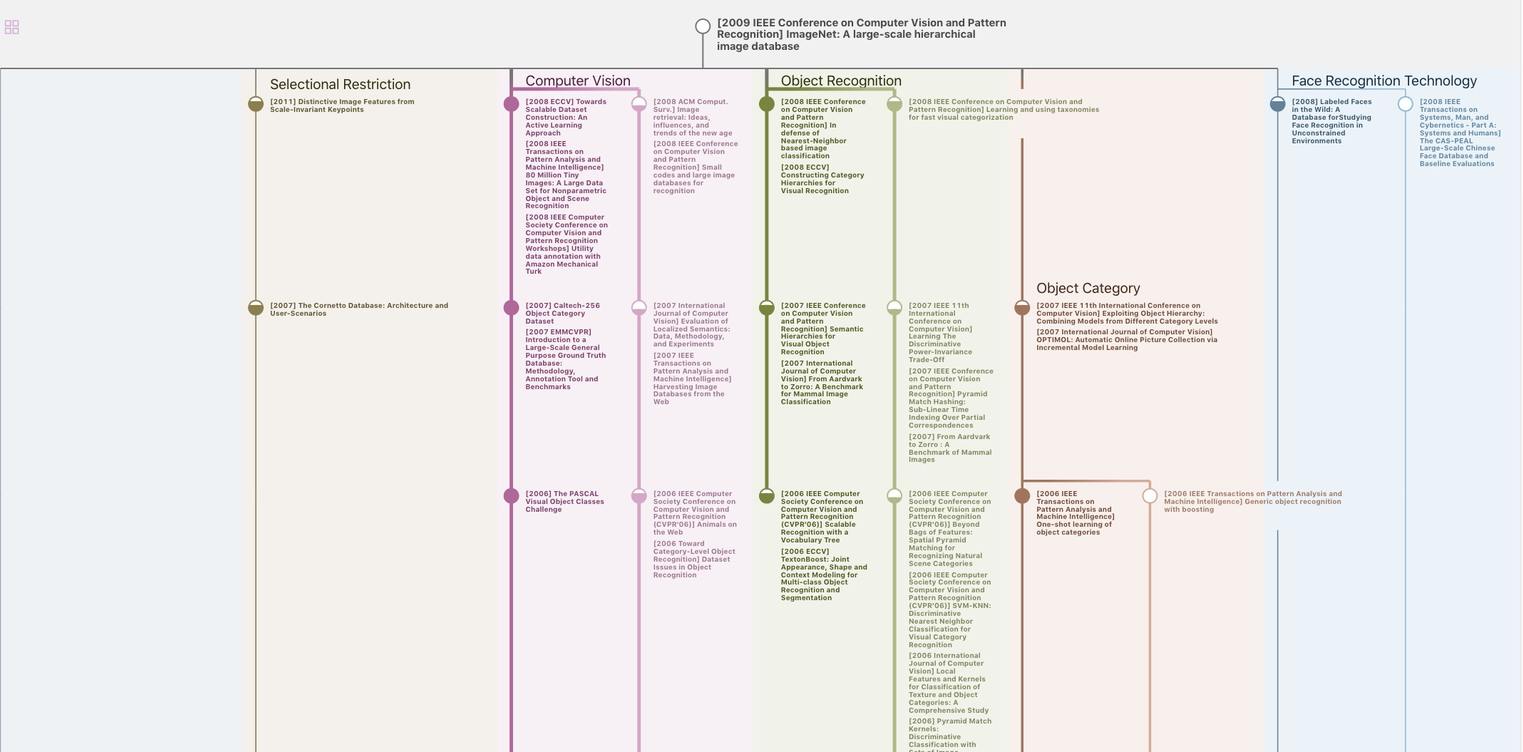
生成溯源树,研究论文发展脉络
Chat Paper
正在生成论文摘要