Perception matters: Exploring imperceptible and transferable anti-forensics for GAN-generated fake face imagery detection
PATTERN RECOGNITION LETTERS(2021)
摘要
Recently, generative adversarial networks (GANs) can generate photo-realistic fake facial images which are perceptually indistinguishable from real face photos, promoting research on fake face detection. Though fake face forensics can achieve high detection accuracy, their anti-forensic counterparts are less investigated. Here we explore more imperceptible and transferable anti-forensics for fake face imagery detection based on adversarial attacks. Since facial and background regions are often smooth, even small perturbation could cause noticeable perceptual impairment in fake face images. Therefore it makes existing transfer-based adversarial attacks ineffective as an anti-forensic method. Our perturbation analysis reveals the intuitive reason of the perceptual degradation issue when directly applying such existing attacks. We then propose a novel adversarial attack method, better suitable for image anti-forensics, in the transformed color domain by considering visual perception. Conceptually simple yet effective, the proposed method can fool both deep learning and non-deep learning based forensic detectors, achieving higher adversarial transferability and significantly improved visual quality. Specially, when adversaries consider imperceptibility as a constraint, the proposed anti-forensic method achieves the state-of-the-art attacking performances in the transfer-based black-box setting (i.e. around 30% higher attack transferability than baseline attacks). More imperceptible and more transferable , the proposed method raises new security concerns to fake face imagery detection. We have released our code for public use, and hopefully the proposed method can be further explored in related forensic applications as an anti-forensic benchmark. (c) 2021 Elsevier B.V. All rights reserved.
更多查看译文
关键词
Fake face imagery anti-forensics,Imperceptible attacks,Transferable attacks,Improved adversarial attack
AI 理解论文
溯源树
样例
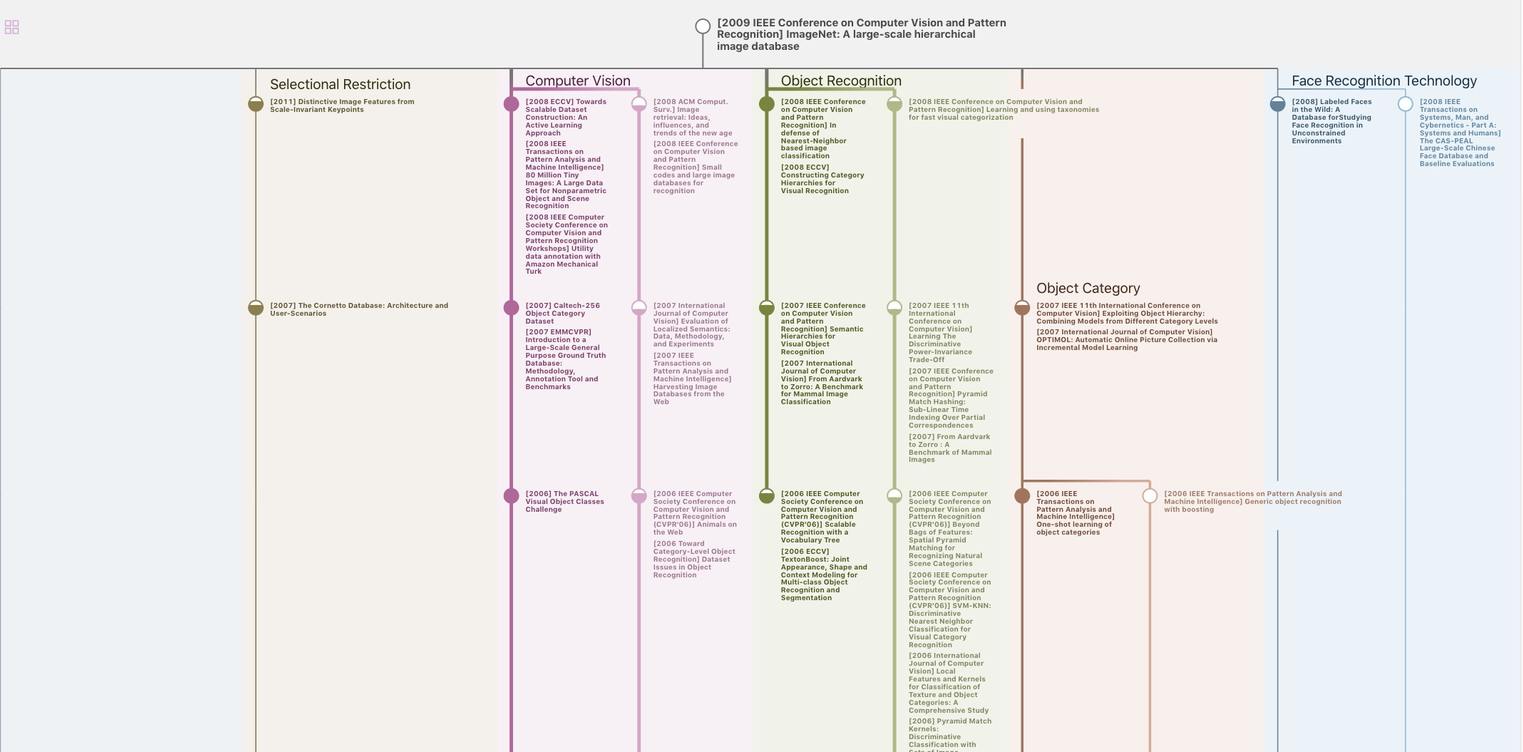
生成溯源树,研究论文发展脉络
Chat Paper
正在生成论文摘要