Unsupervised One-shot Learning of Both Specific Instances and Generalised Classes with a Hippocampal Architecture
Australasian Conference on Artificial Intelligence(2020)
摘要
Established experimental procedures for one-shot machine learning do not test the ability to learn or remember specific instances of classes, a key feature of animal intelligence. Distinguishing specific instances is necessary for many real-world tasks, such as remembering which cup belongs to you. Generalisation within classes conflicts with the ability to separate instances of classes, making it difficult to achieve both capabilities within a single architecture. We propose an extension to the standard Omniglot classification-generalisation framework that additionally tests the ability to distinguish specific instances after one exposure and introduces noise and occlusion corruption. Learning is defined as an ability to classify as well as recall training samples. Complementary Learning Systems (CLS) is a popular model of mammalian brain regions believed to play a crucial role in learning from a single exposure to a stimulus. We created an artificial neural network implementation of CLS and applied it to the extended Omniglot benchmark. Our unsupervised model demonstrates comparable performance to existing supervised ANNs on the Omniglot classification task (requiring generalisation), without the need for domain-specific inductive biases. On the extended Omniglot instance-recognition task, the same model also demonstrates significantly better performance than a baseline nearest-neighbour approach, given partial occlusion and noise.
更多查看译文
关键词
CLS, Hippocampus, One-shot, Specifics, Instances, Unsupervised, Generalization.
AI 理解论文
溯源树
样例
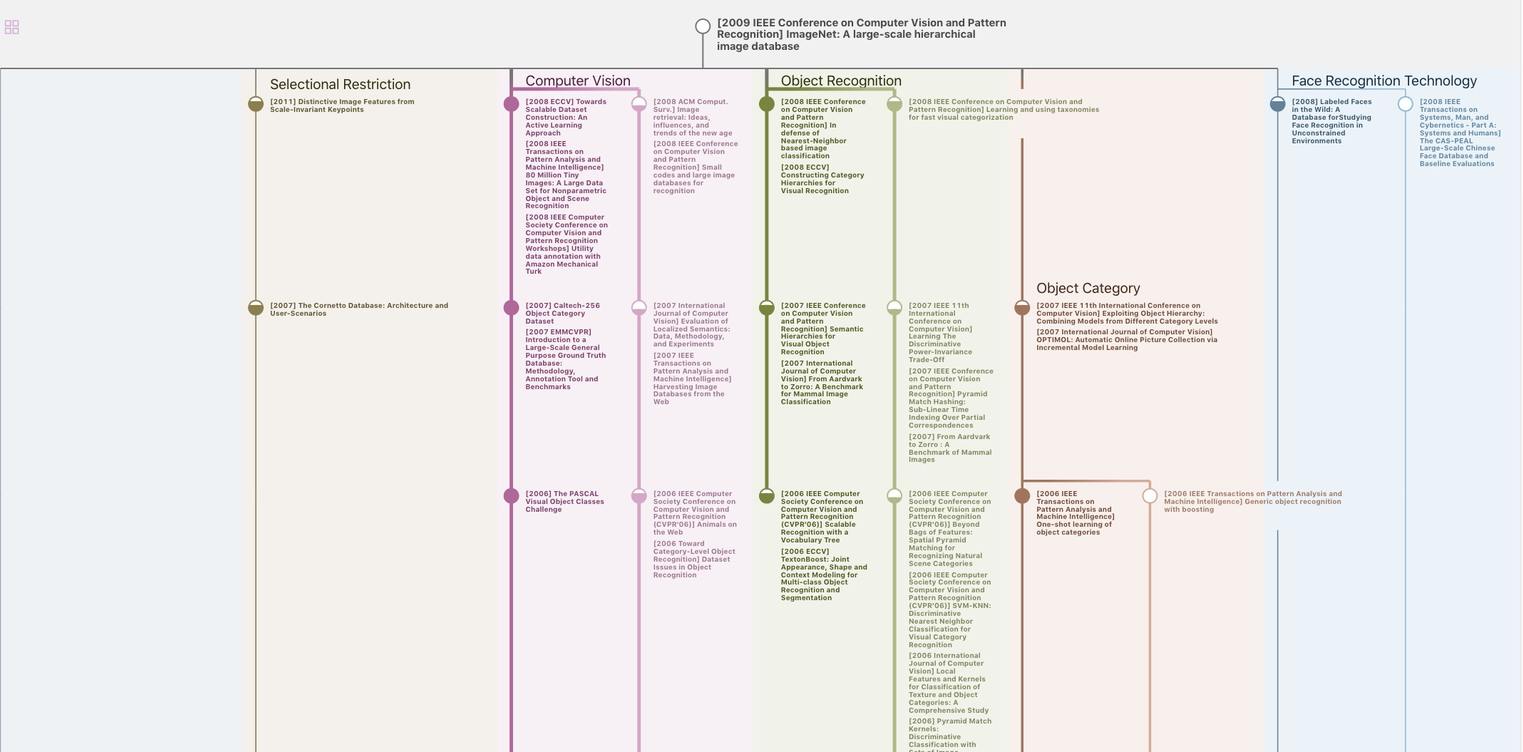
生成溯源树,研究论文发展脉络
Chat Paper
正在生成论文摘要