Improving the robustness of beach water quality modeling using an ensemble machine learning approach
The Science of the total environment(2021)
摘要
Microbial pollution of beach water can expose swimmers to harmful pathogens. Predictive modeling provides an alternative method for beach management that addresses several limitations associated with traditional culture-based methods of assessing water quality. Widely-used machine learning methods often suffer from high variability in performance from one year or beach to another. Therefore, the best machine learning method varies between beaches and years, making method selection difficult. This study proposes an ensemble machine learning approach referred to as model stacking that has a two-layered learning structure, where the outputs of five widely-used individual machine learning models (multiple linear regression, partial least square, sparse partial least square, random forest, and Bayesian network) are taken as input features for another model that produces the final prediction. Applying this approach to three beaches along eastern Lake Erie, New York, USA, we show that generally the model stacking approach was able to generate reliably good predictions compared to all of the five base models. The accuracy rankings of the stacking model consistently stayed 1st or 2nd every year, with yearly-average accuracy of 78%, 81%, and 82.3% at the three studied beaches, respectively. This study highlights the value of the model stacking approach in predicting beach water quality and solving other pressing environmental problems. (C) 2020 Elsevier B.V. All rights reserved.
更多查看译文
关键词
Water quality,Fecal indicator bacteria,E. coli,Model stacking,Machine learning model
AI 理解论文
溯源树
样例
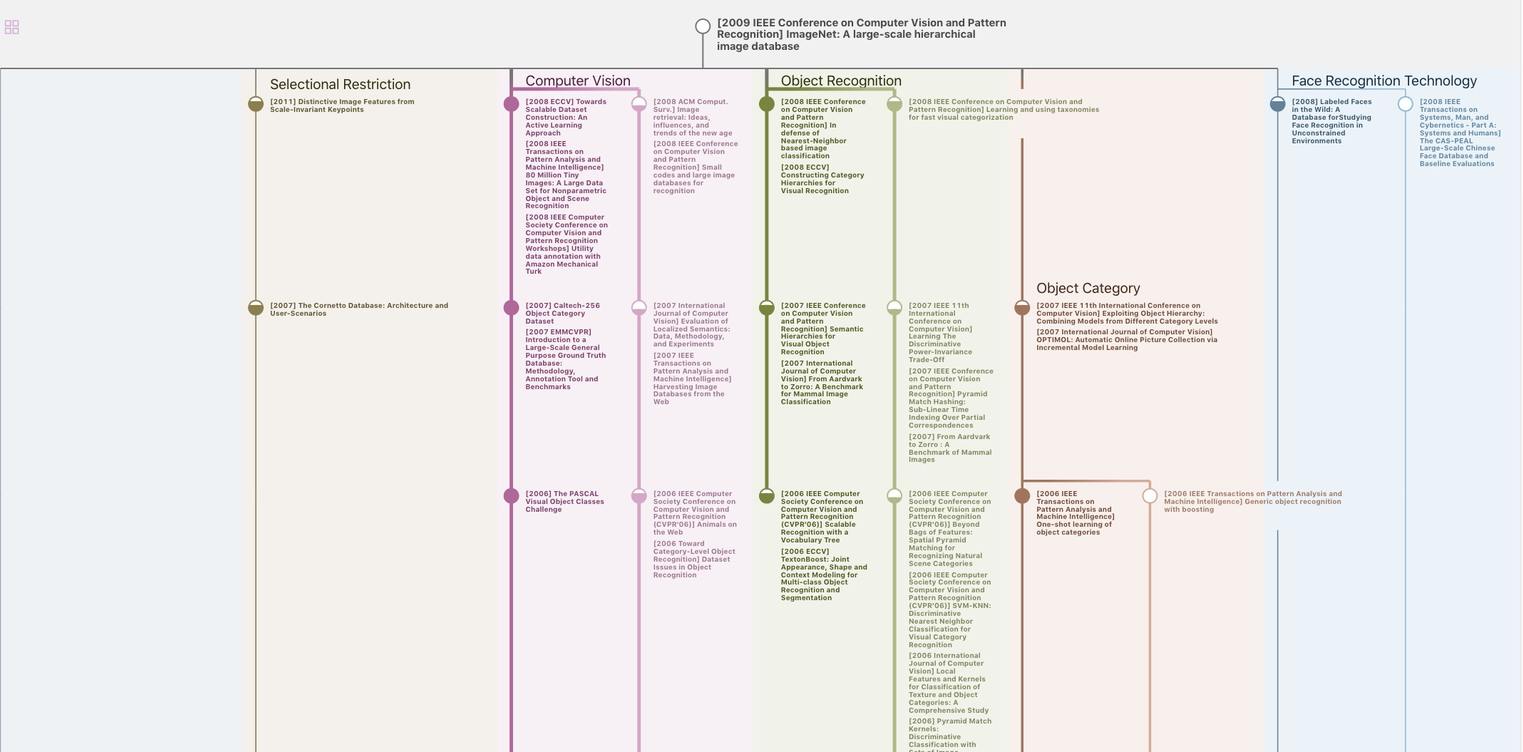
生成溯源树,研究论文发展脉络
Chat Paper
正在生成论文摘要