Kernel Fusion Method for Detecting Cancer Subtypes via Selecting Relevant Expression Data.
FRONTIERS IN GENETICS(2020)
摘要
Recently, cancer has been characterized as a heterogeneous disease composed of many different subtypes. Early diagnosis of cancer subtypes is an important study of cancer research, which can be of tremendous help to patients after treatment. In this paper, we first extract a novel dataset, which contains gene expression, miRNA expression, and isoform expression of five cancers from The Cancer Genome Atlas (TCGA). Next, to avoid the effect of noise existing in 60, 483 genes, we select a small number of genes by using LASSO that employs gene expression and survival time of patients. Then, we construct one similarity kernel for each expression data by using Chebyshev distance. And also, We used SKF to fused the three similarity matrix composed of gene, Iso, and miRNA, and finally clustered the fused similarity matrix with spectral clustering. In the experimental results, our method has betterP-value in the Cox model than other methods on 10 cancer data from Jiang Dataset and Novel Dataset. We have drawn different survival curves for different cancers and found that some genes play a key role in cancer. For breast cancer, we find out that HSPA2A, RNASE1, CLIC6, and IFITM1 are highly expressed in some specific groups. For lung cancer, we ensure that C4BPA, SESN3, and IRS1 are highly expressed in some specific groups. The code and all supporting data files are available from.
更多查看译文
关键词
cancer subtype,similarity Kernel fusion,LASSO,gene expression,miRNA expression,isform level
AI 理解论文
溯源树
样例
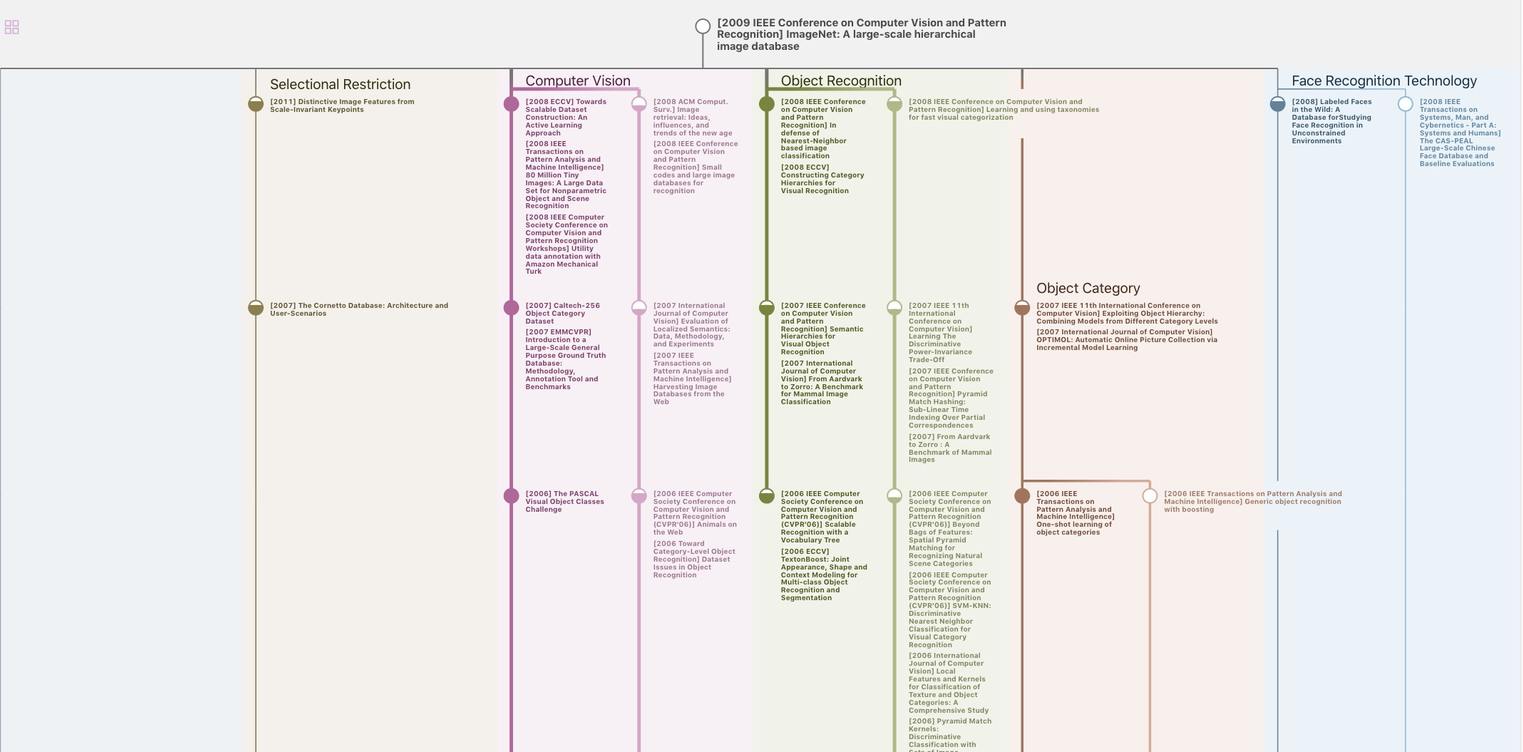
生成溯源树,研究论文发展脉络
Chat Paper
正在生成论文摘要