Unsupervised Deep Persistent Monocular Visual Odometry and Depth Estimation in Extreme Environments
2021 IEEE/RSJ INTERNATIONAL CONFERENCE ON INTELLIGENT ROBOTS AND SYSTEMS (IROS)(2021)
摘要
In recent years, unsupervised deep learning approaches have received significant attention to estimating the depth and visual odometry (VO) from unlabelled monocular image sequences. However, their performance is limited in challenging environments due to perceptual degradation, occlusions, and rapid motions. Moreover, the existing unsupervised methods suffer from the lack of scale-consistency constraints across frames, which causes that the VO estimators fail to provide persistent trajectories over long sequences. In this study, we propose an unsupervised monocular deep VO framework that predicts a six-degrees-of-freedom pose camera motion and depth map of the scene from unlabelled RGB image sequences. We provide detailed quantitative and qualitative evaluations of the proposed framework on a) a challenging dataset collected during the DARPA Subterranean challenge(1); and b) the benchmark KITTI and Cityscapes datasets. The proposed approach significantly outperforms state-of-the-art unsupervised deep VO and depth prediction methods under perceptually degraded conditions providing better results for both pose estimation and depth recovery. Furthermore, it achieves state-of-the-art results in most of the VO and depth metrics on benchmark datasets. The presented approach is part of the solution used by the COSTAR team participating in the DARPA Subterranean Challenge.
更多查看译文
关键词
unsupervised monocular deep VO framework,depth map,unlabelled RGB image sequences,depth prediction methods,depth recovery,DARPA Subterranean Challenge,unsupervised deep persistent monocular visual odometry,extreme environments,unsupervised deep learning approaches,unlabelled monocular image sequences,perceptual degradation,scale-consistency constraints,six-degrees-of-freedom pose camera motion,Cityscapes datasets,benchmark KITTI datasets,pose estimation
AI 理解论文
溯源树
样例
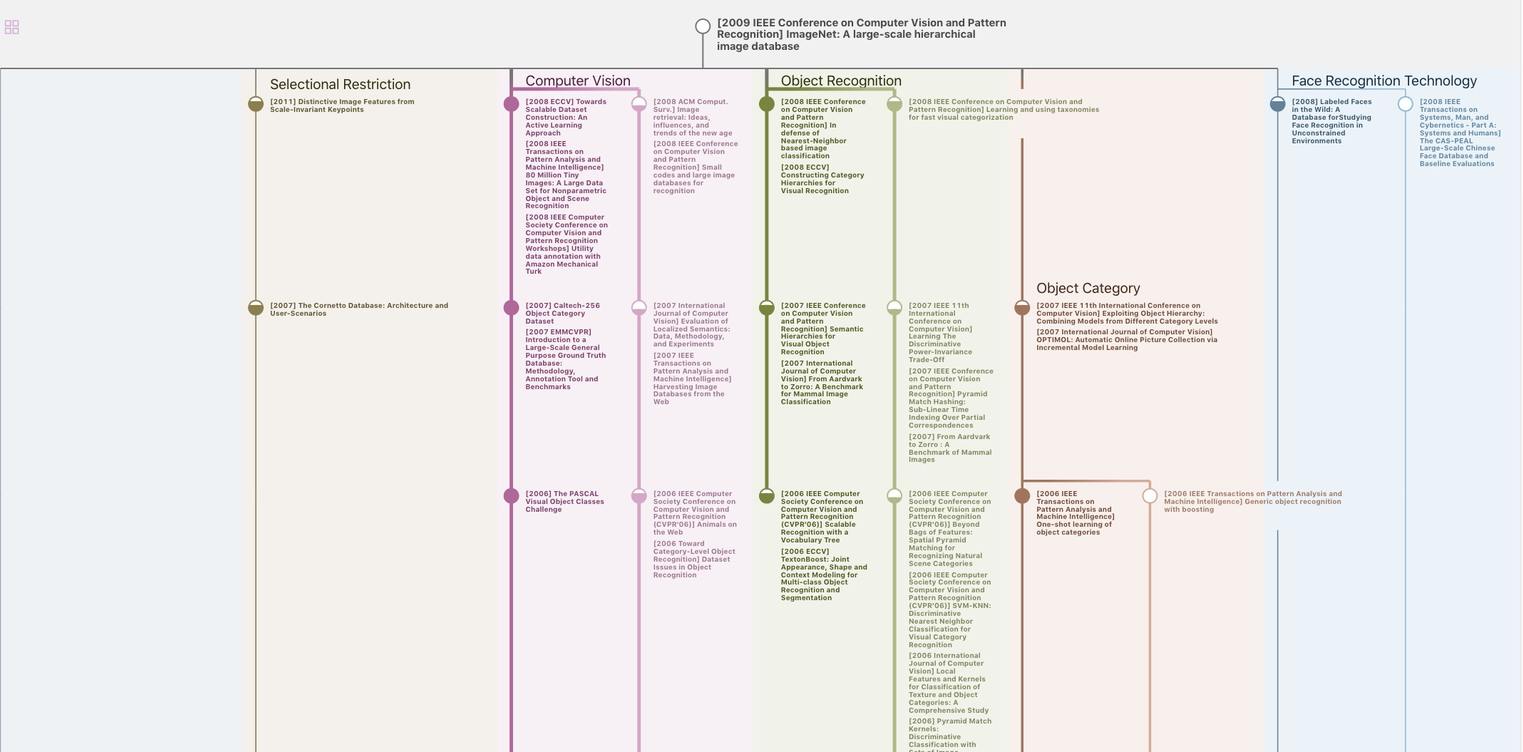
生成溯源树,研究论文发展脉络
Chat Paper
正在生成论文摘要