Machine Learning Forecasting Of Active Nematics
SOFT MATTER(2021)
摘要
Active nematics are a class of far-from-equilibrium materials characterized by local orientational order of force-generating, anisotropic constitutes. Traditional methods for predicting the dynamics of active nematics rely on hydrodynamic models, which accurately describe idealized flows and many of the steady-state properties, but do not capture certain detailed dynamics of experimental active nematics. We have developed a deep learning approach that uses a Convolutional Long-Short-Term-Memory (ConvLSTM) algorithm to automatically learn and forecast the dynamics of active nematics. We demonstrate our purely data-driven approach on experiments of 2D unconfined active nematics of extensile microtubule bundles, as well as on data from numerical simulations of active nematics.
更多查看译文
关键词
machine learning forecasting,machine learning,active
AI 理解论文
溯源树
样例
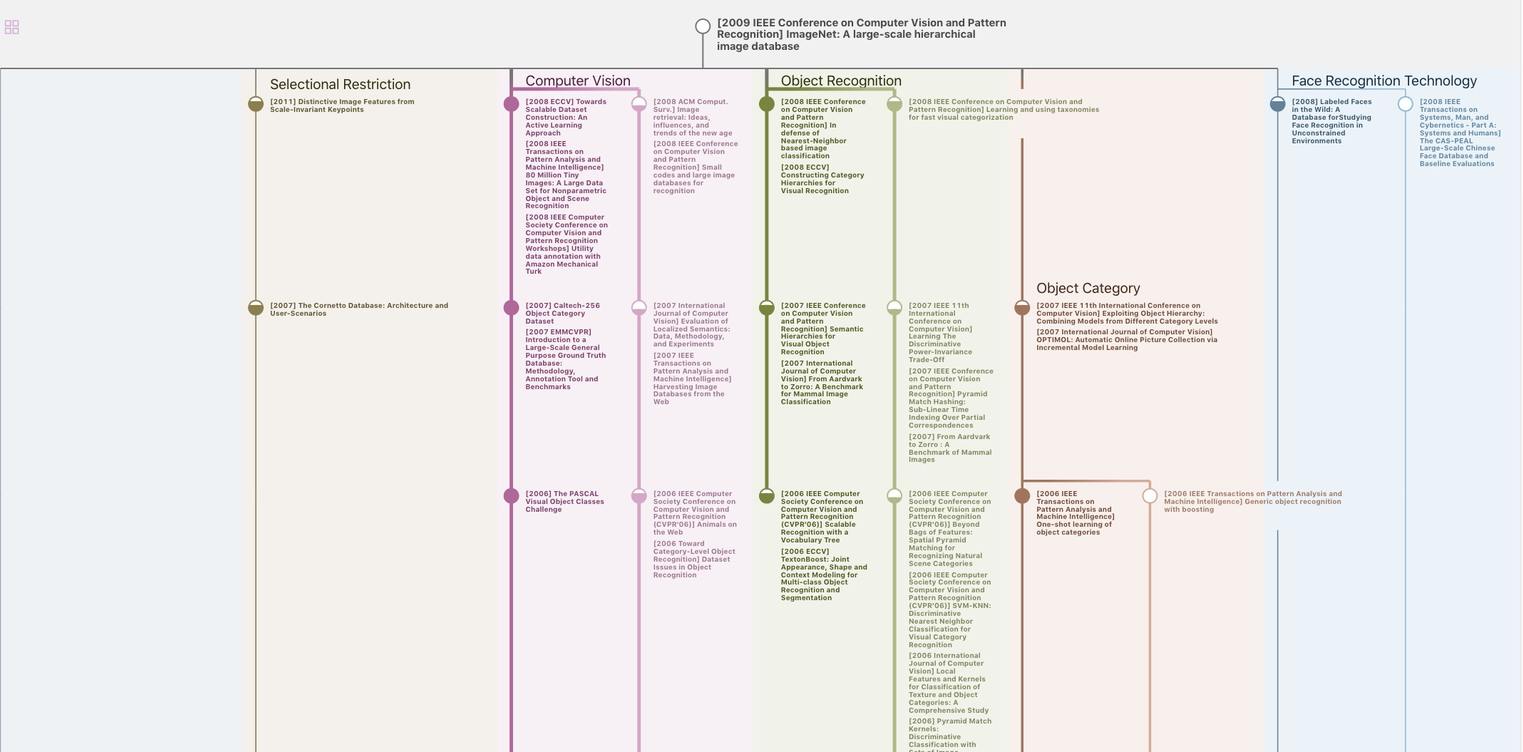
生成溯源树,研究论文发展脉络
Chat Paper
正在生成论文摘要