Fast Network Community Detection With Profile-Pseudo Likelihood Methods
arxiv(2023)
摘要
The stochastic block model is one of the most studied network models for community detection, and fitting its likelihood function on large-scale networks is known to be challenging. One prominent work that overcomes this computational challenge is the fast pseudo-likelihood approach proposed by Amini et al. for fitting stochastic block models to large sparse networks. However, this approach does not have convergence guarantee, and may not be well suited for small and medium scale networks. In this article, we propose a novel likelihood based approach that decouples row and column labels in the likelihood function, enabling a fast alternating maximization. This new method is computationally efficient, performs well for both small- and large-scale networks, and has provable convergence guarantee. We show that our method provides strongly consistent estimates of communities in a stochastic block model. We further consider extensions of our proposed method to handle networks with degree heterogeneity and bipartite properties. Supplementary materials for this article are available online.
更多查看译文
关键词
Network analysis,Profile likelihood,Pseudo likelihood,Stochastic block model,Strong consistency
AI 理解论文
溯源树
样例
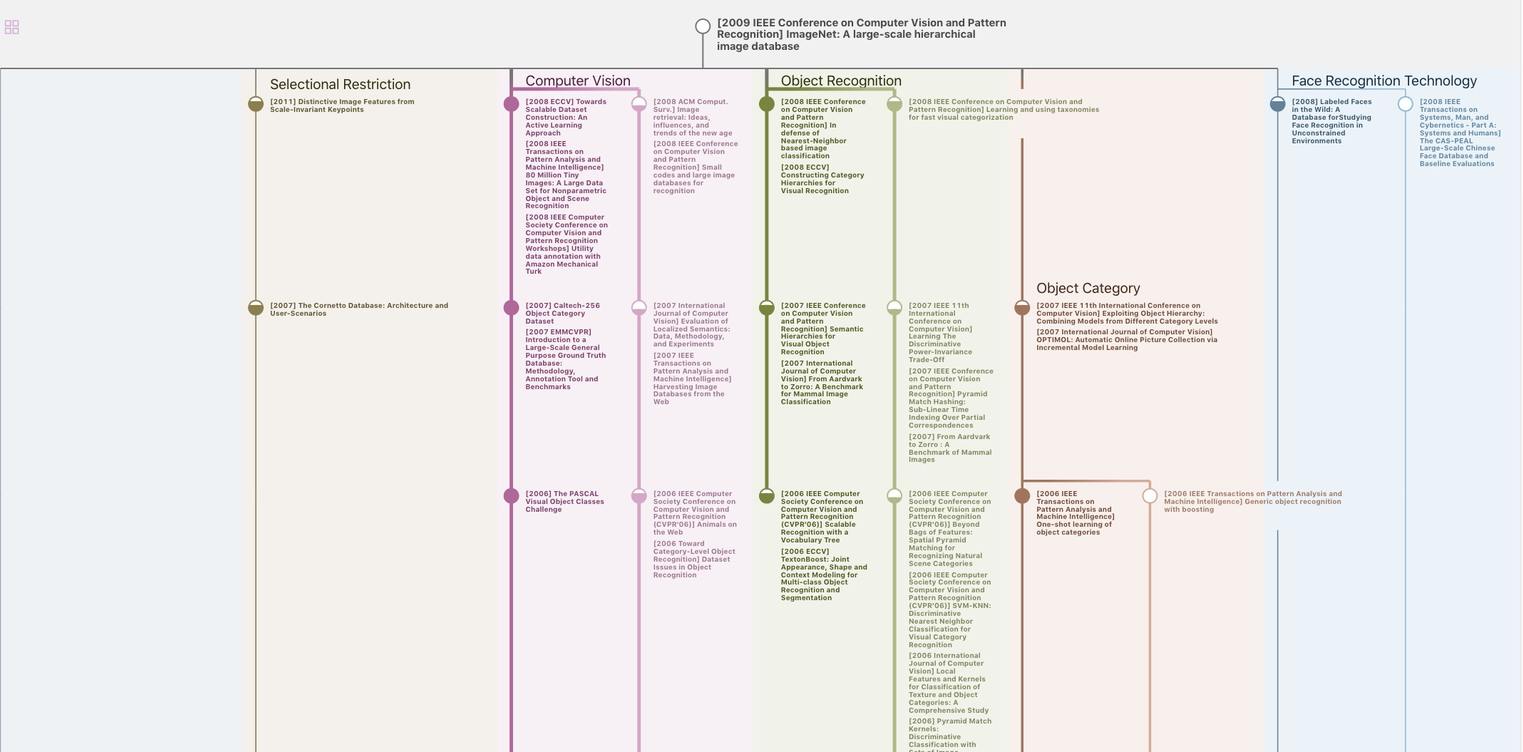
生成溯源树,研究论文发展脉络
Chat Paper
正在生成论文摘要