A flexible class of dependence-aware multi-label loss functions
Machine Learning(2022)
摘要
The idea to exploit label dependencies for better prediction is at the core of methods for multi-label classification (MLC), and performance improvements are normally explained in this way. Surprisingly, however, there is no established methodology that allows to analyze the dependence-awareness of MLC algorithms. With that goal in mind, we introduce a class of loss functions that are able to capture the important aspect of label dependence. To this end, we leverage the mathematical framework of non-additive measures and integrals. Roughly speaking, a non-additive measure allows for modeling the importance of correct predictions of label subsets (instead of single labels), and thereby their impact on the overall evaluation, in a flexible way. The well-known Hamming and subset 0/1 losses are rather extreme special cases of this function class, which give full importance to single label sets or the entire label set, respectively. We present concrete instantiations of this class, which appear to be especially appealing from a modeling perspective. The assessment of multi-label classifiers in terms of these losses is illustrated in an empirical study, clearly showing their aptness at capturing label dependencies. Finally, while not being the main goal of this study, we also show some preliminary results on the minimization of this parametrized family of losses.
更多查看译文
关键词
Multi-label classification, Loss function, Non-additive measures, Analysis, Label dependence
AI 理解论文
溯源树
样例
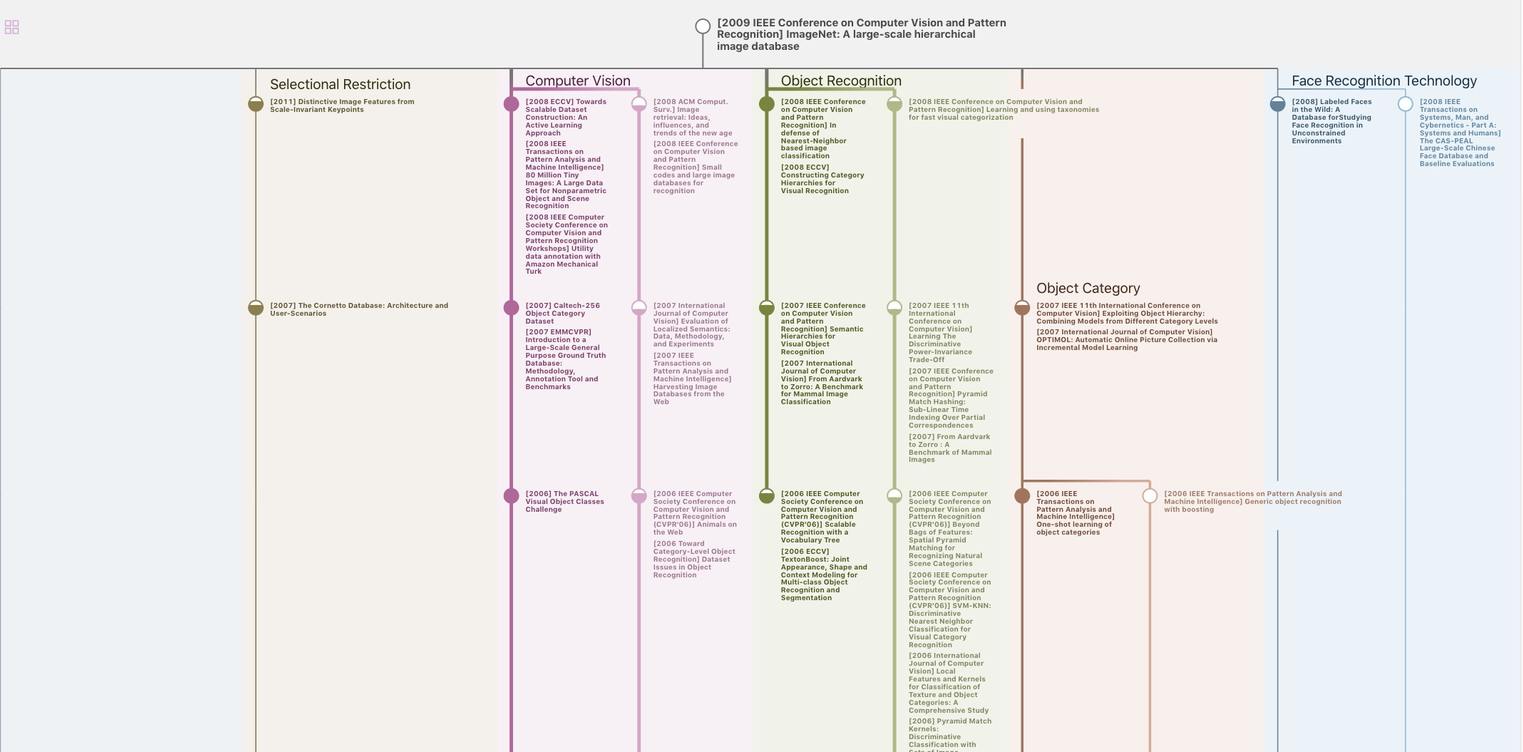
生成溯源树,研究论文发展脉络
Chat Paper
正在生成论文摘要