Learning from Non-Binary Constituency Trees via Tensor Decomposition
COLING(2020)
摘要
Processing sentence constituency trees in binarised form is a common and popular approach in literature. However, constituency trees are non-binary by nature. The binarisation procedure changes deeply the structure, furthering constituents that instead are close. In this work, we introduce a new approach to deal with non-binary constituency trees which leverages tensor-based models. In particular, we show how a powerful composition function based on the canonical tensor decomposition can exploit such a rich structure. A key point of our approach is the weight sharing constraint imposed on the factor matrices, which allows limiting the number of model parameters. Finally, we introduce a Tree-LSTM model which takes advantage of this composition function and we experimentally assess its performance on different NLP tasks.
更多查看译文
关键词
tensor,decomposition,learning,non-binary
AI 理解论文
溯源树
样例
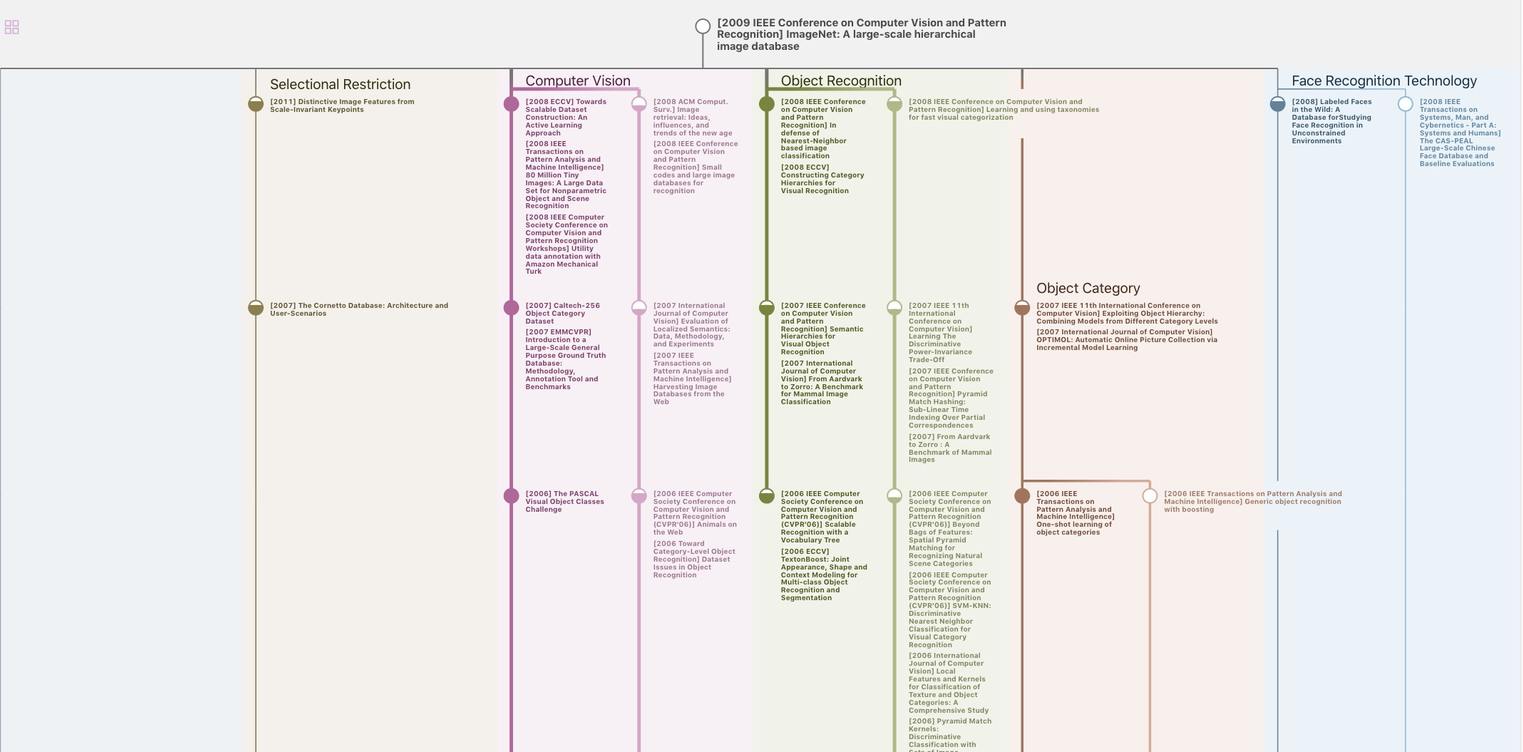
生成溯源树,研究论文发展脉络
Chat Paper
正在生成论文摘要