Deep Pairwise Hashing for Cold-Start Recommendation
IEEE Transactions on Knowledge and Data Engineering(2022)
摘要
Recommendation efficiency and data sparsity problems have been regarded as two challenges of improving performance for online recommendation. Most of the previous related work focus on improving recommendation accuracy instead of efficiency. In this paper, we propose a Deep Pairwise Hashing (DPH) to map users and items to binary vectors in Hamming space, where a user's preference for an item can be efficiently calculated by Hamming distance, which significantly improves the efficiency of online recommendation. To alleviate data sparsity and cold-start problems, the user-item interactive information and item content information are unified to learn effective representations of items and users. Specifically, we first pre-train robust item representation from item content data by a Denoising Auto-encoder instead of other deterministic deep learning frameworks; then we finetune the entire framework by adding a pairwise loss objective with discrete constraints; moreover, DPH aims to minimize a pairwise ranking loss that is consistent with the ultimate goal of recommendation. Finally, we adopt the alternating optimization method to optimize the proposed model with discrete constraints. Extensive experiments on three different datasets show that DPH can significantly advance the state-of-the-art frameworks regarding data sparsity and item cold-start recommendation.
更多查看译文
关键词
Recommender system,denosing auto-encoder,hash code,cold-start
AI 理解论文
溯源树
样例
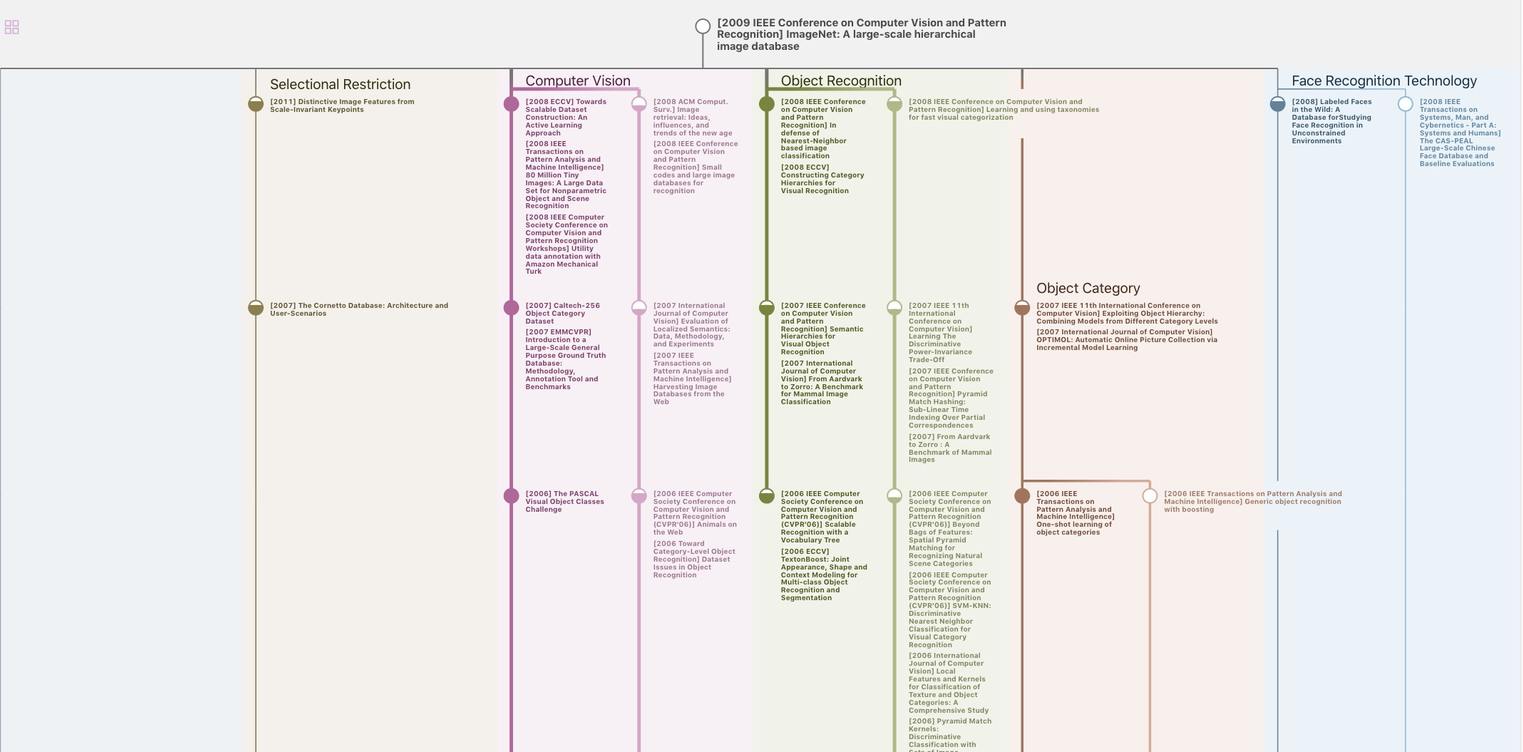
生成溯源树,研究论文发展脉络
Chat Paper
正在生成论文摘要