Introducing Various Semantic Models for Amharic: Experimentation and Evaluation with Multiple Tasks and Datasets.
Future Internet(2021)
摘要
The availability of different pre-trained semantic models enabled the quick development of machine learning components for downstream applications. Despite the availability of abundant text data for low resource languages, only a few semantic models are publicly available. Publicly available pre-trained models are usually built as a multilingual version of semantic models that can not fit well for each language due to context variations. In this work, we introduce different semantic models for Amharic. After we experiment with the existing pre-trained semantic models, we trained and fine-tuned nine new different models using a monolingual text corpus. The models are build using word2Vec embeddings, distributional thesaurus (DT), contextual embeddings, and DT embeddings obtained via network embedding algorithms. Moreover, we employ these models for different NLP tasks and investigate their impact. We find that newly trained models perform better than pre-trained multilingual models. Furthermore, models based on contextual embeddings from RoBERTA perform better than the word2Vec models.
更多查看译文
关键词
datasets,neural networks,semantic models,Amharic NLP,low-resource language,text tagging
AI 理解论文
溯源树
样例
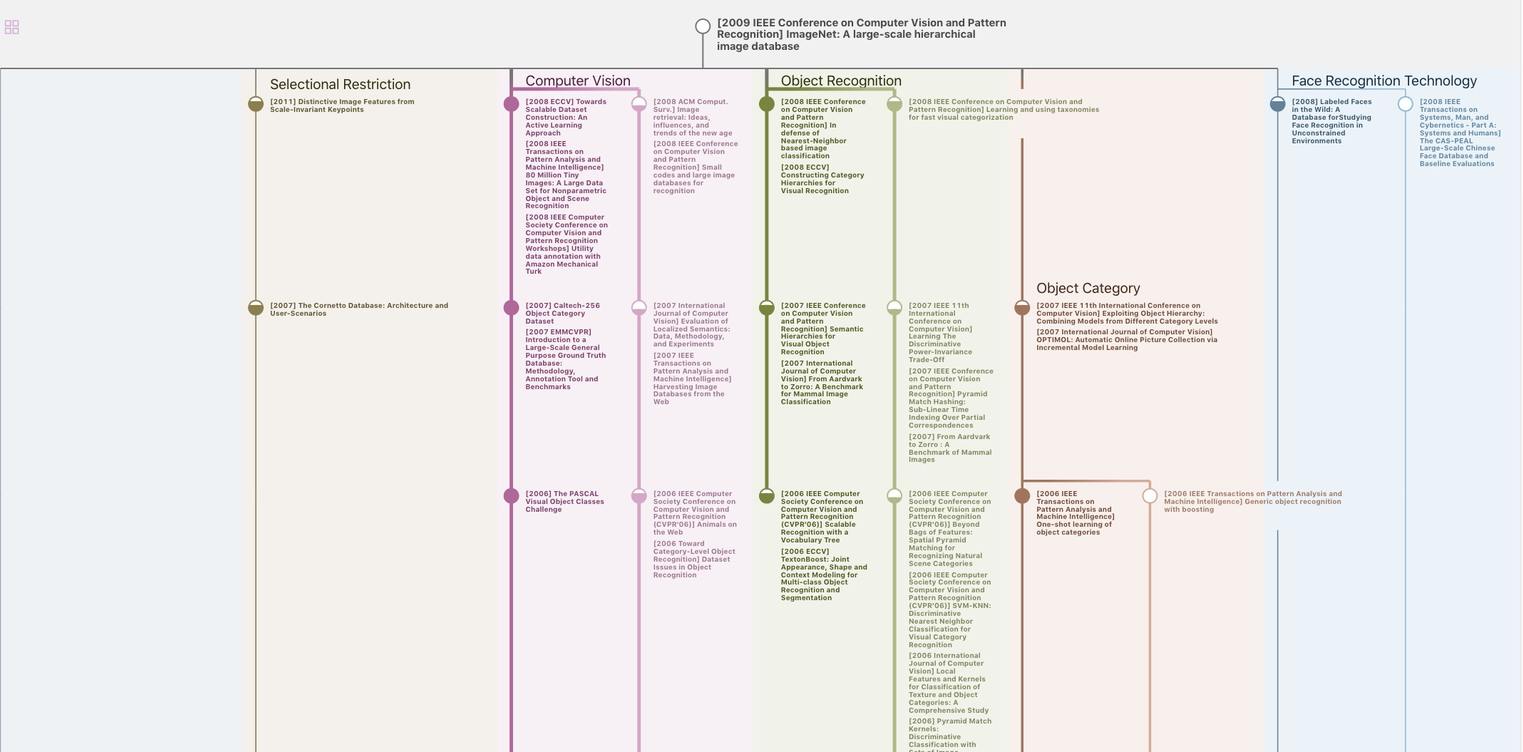
生成溯源树,研究论文发展脉络
Chat Paper
正在生成论文摘要