Scalable unsupervised multi-criteria trajectory segmentation and driving preference mining
SIGSPATIAL '20: 28th International Conference on Advances in Geographic Information Systems Seattle Washington November, 2020(2020)
摘要
We present analysis techniques for large trajectory data sets that aim to provide a semantic understanding of trajectories reaching beyond them being point sequences in time and space. The presented techniques use a driving preference model w.r.t. road segment traversal costs, e.g., travel time and distance, to analyze and explain trajectories.
In particular, we present trajectory mining techniques that can (a) find interesting points within a trajectory indicating, e.g., a via-point, and (b) recover the driving preferences of a driver based on their chosen trajectory. We evaluate our techniques on the tasks of via-point identification and personalized routing using a data set of more than 1 million vehicle trajectories collected throughout Denmark during a 3-year period. Our techniques can be implemented efficiently and are highly parallelizable, allowing them to scale to millions or billions of trajectories.
更多查看译文
关键词
driving preference mining,trajectory,segmentation,multi-criteria
AI 理解论文
溯源树
样例
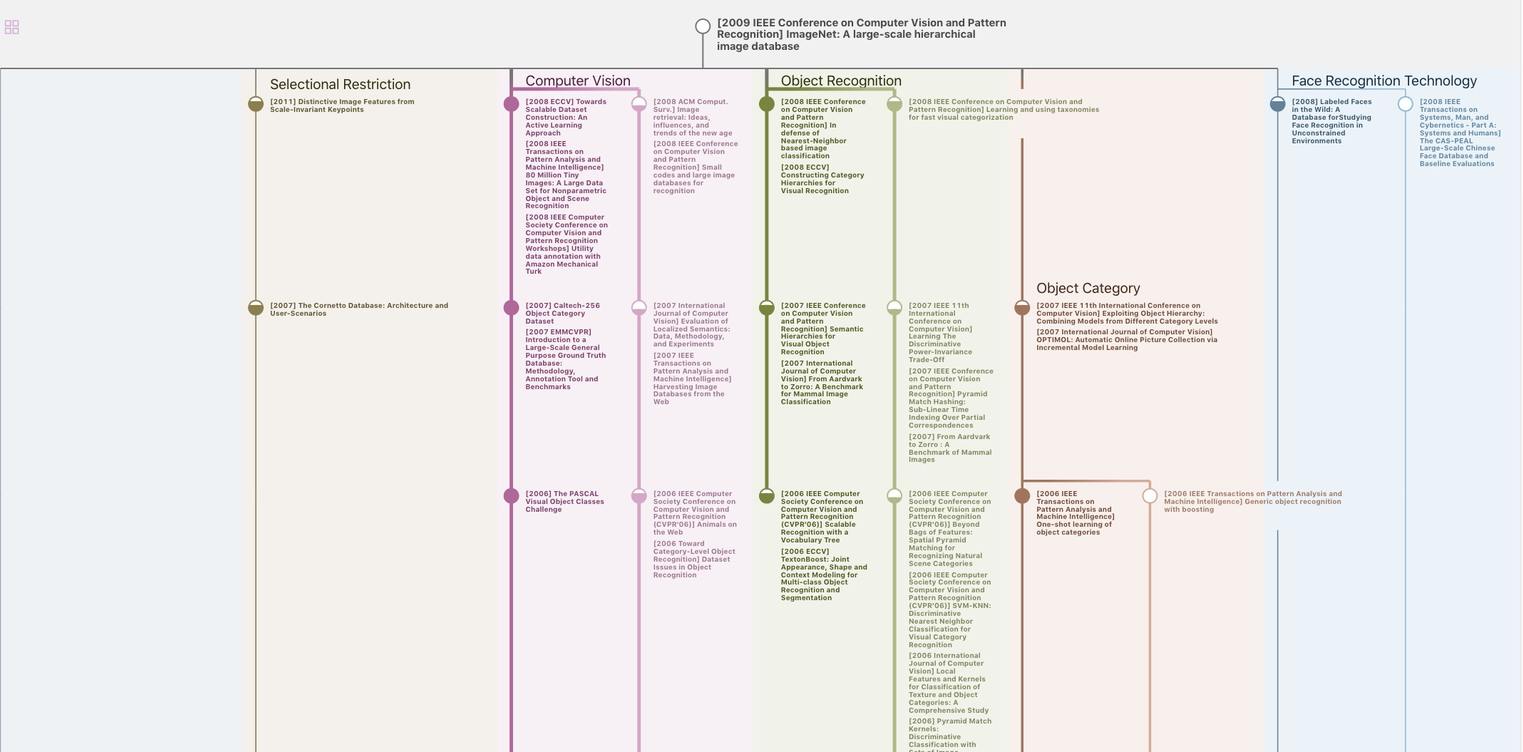
生成溯源树,研究论文发展脉络
Chat Paper
正在生成论文摘要