Quantitative ultrasound radiomics using texture derivatives in prediction of treatment response to neo-adjuvant chemotherapy for locally advanced breast cancer.
Oncotarget(2020)
摘要
BACKGROUND:To investigate quantitative ultrasound (QUS) based higher-order texture derivatives in predicting the response to neoadjuvant chemotherapy (NAC) in patients with locally advanced breast cancer (LABC).
MATERIALS AND METHODS:100 Patients with LABC were scanned before starting NAC. Five QUS parametric image-types were generated from radio-frequency data over the tumor volume. From each QUS parametric-image, 4 grey level co-occurrence matrix-based texture images were derived (20 QUS-Tex1), which were further processed to create texture derivatives (80 QUS-Tex1-Tex2). Patients were classified into responders and non-responders based on clinical/pathological responses to treatment. Three machine learning algorithms based on linear discriminant (FLD), k-nearest-neighbors (KNN), and support vector machine (SVM) were used for developing radiomic models of response prediction.
RESULTS:A KNN-model provided the best results with sensitivity, specificity, accuracy, and area under curve (AUC) of 87%, 81%, 82%, and 0.86, respectively. The most helpful features in separating the two response groups were QUS-Tex1-Tex2 features. The 5-year recurrence-free survival (RFS) calculated for KNN predicted responders and non-responders using QUS-Tex1-Tex2 model were comparable to RFS for the actual response groups.
CONCLUSIONS:We report the first study demonstrating QUS texture-derivative methods in predicting NAC responses in LABC, which leads to better results compared to using texture features alone.
更多查看译文
关键词
breast cancer,neoadjuvant chemotherapy,quantitative ultrasound,radiomics,texture derivatives
AI 理解论文
溯源树
样例
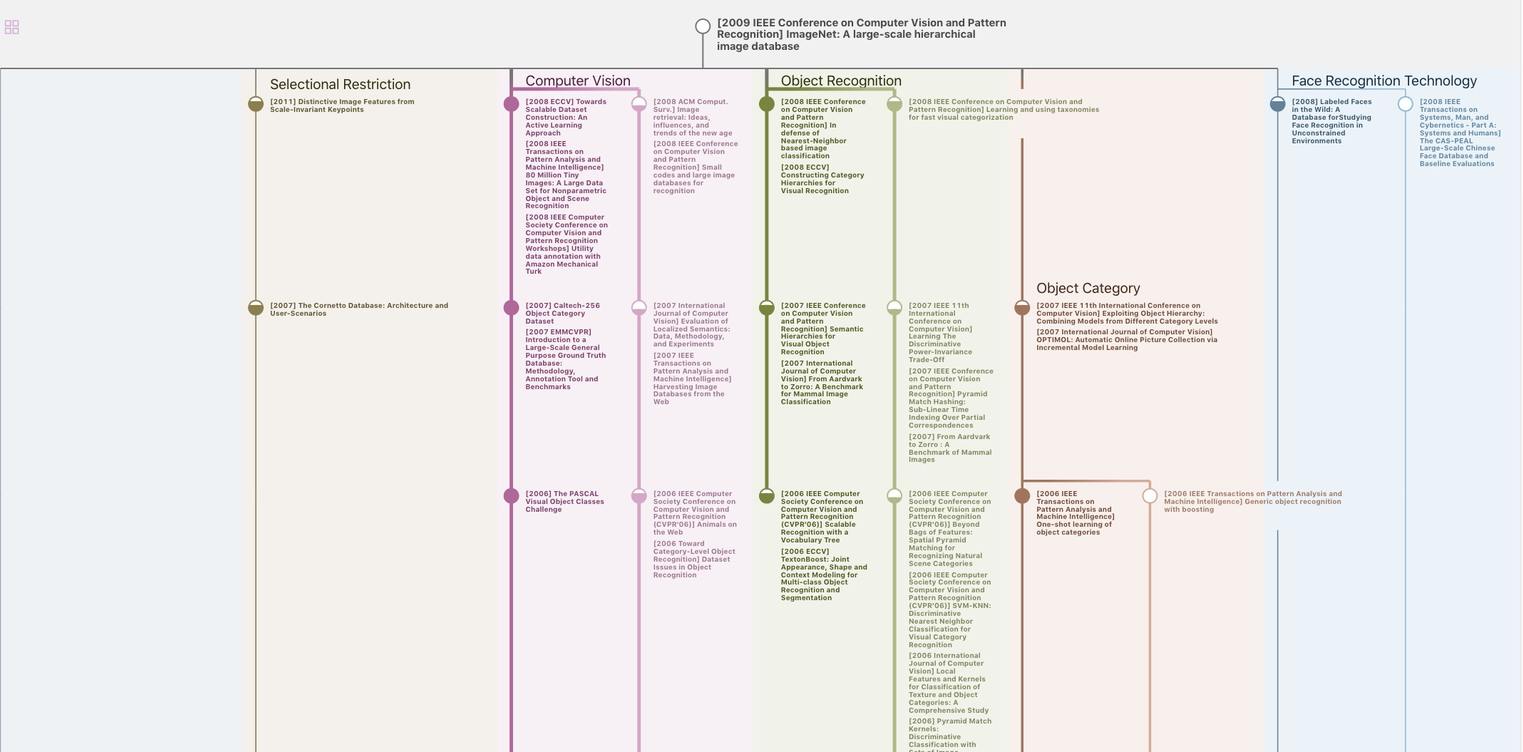
生成溯源树,研究论文发展脉络
Chat Paper
正在生成论文摘要