Prediction of culture-positive sepsis and selection of empiric antibiotics in critically ill patients with complicated intra-abdominal infections: a retrospective study
European Journal of Trauma and Emergency Surgery(2020)
摘要
Purpose To compare the mortality rates between culture-positive and culture-negative sepsis in complicated intra-abdominal infections (cIAI) and investigate the predictors of culture-positivity and their causative microorganisms. Materials and methods The medical records of 1581 adult patients who underwent emergency gastrointestinal surgery between January 2013 and December 2018 were reviewed retrospectively. A total of 239 patients with sepsis or septic shock who were admitted to an emergency department, underwent emergency surgery for cIAI, and needed postoperative intensive care unit care were included and divided into two groups according to their initial blood and peritoneal culture results. Results Among the 239 patients, 200 were culture-negative and 39 were culture-positive. The culture-positive group had higher in-hospital (35.9% vs 14.5%; P = .001) and 30-day mortality (30.8% vs 12.0%; P = .003) than the culture-negative group. Colon involvement (OR 4.211; 95% CI 1.909–9.287; P < .001) and higher Sequential Organ Failure Assessment (SOFA) score (OR 1.169; 95% CI 1.065–1.282; P = .001) were shown to be the predictors of culture-positive sepsis for cIAI. Regarding antibiotic sensitivity, 31.6% of the gram-positive bacteria were methicillin-resistant and 42.1% of the gram-negative bacteria were extended spectrum β-lactamase-producing Enterobacteriaceae. Conclusions Patients with cIAI had higher mortality rates in culture-positive sepsis than in culture-negative sepsis. High SOFA score and colon involvement were the risk factors associated with culture-positivity. The most common single species grown in the blood or peritoneal cultures was Escherichia coli , and the most common group was Gram-positive cocci.
更多查看译文
关键词
Antibiotic,Critically ill,Intra-abdominal infection
AI 理解论文
溯源树
样例
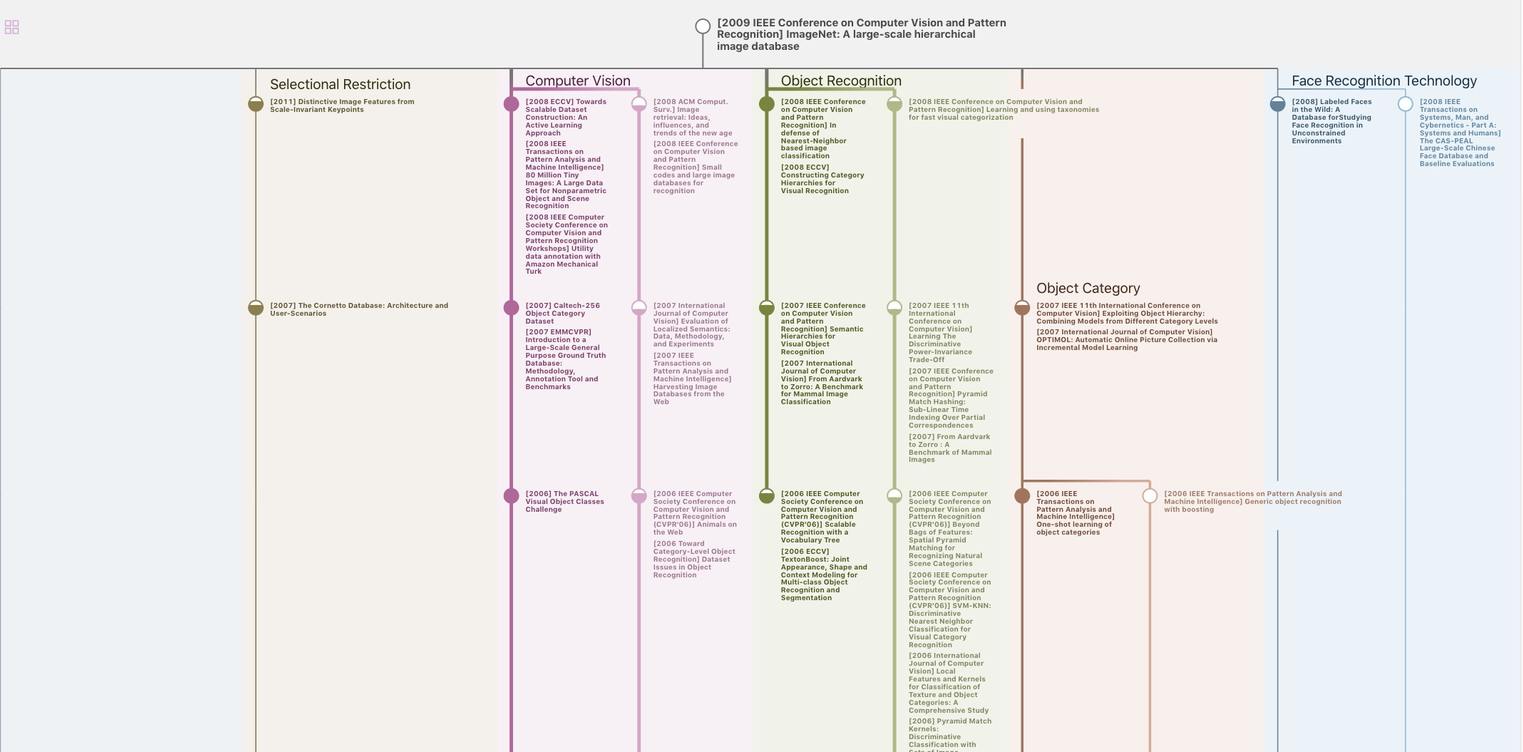
生成溯源树,研究论文发展脉络
Chat Paper
正在生成论文摘要