Short-time deep-learning based source separation for speech enhancement in reverberant environments with beamforming
arxiv(2020)
摘要
The source separation-based speech enhancement problem with multiple beamforming in reverberant indoor environments is addressed in this paper. We propose that more generic solutions should cope with time-varying dynamic scenarios with moving microphone array or sources such as those found in voice-based human-robot interaction or smart speaker applications. The effectiveness of ordinary source separation methods based on statistical models such as ICA and NMF depends on the analysis window size and cannot handle reverberation environments. To address these limitations, a short-term source separation method based on a temporal convolutional network in combination with compact bilinear pooling is presented. The proposed scheme is virtually independent of the analysis window size and does not lose effectiveness when the analysis window is shortened to 1.6s, which in turn is very interesting to tackle the source separation problem in time-varying scenarios. Also, improvements in WER as high as 80% were obtained when compared to ICA and NMF with multi-condition reverberant training and testing, and with time-varying SNR experiments to simulate a moving target speech source. Finally, the experiment with the estimation of the clean signal employing the proposed scheme and a clean trained ASR provided a WER 13% lower than the one obtained with the corrupted signal and a multi-condition trained ASR. This surprising result contradicts the widely adopted practice of using multi-condition trained ASR systems and reinforce the use of speech enhancement methods for user profiling in HRI environments.
更多查看译文
关键词
speech enhancement,reverberant environments,source separation,deep-learning deep-learning,short-time
AI 理解论文
溯源树
样例
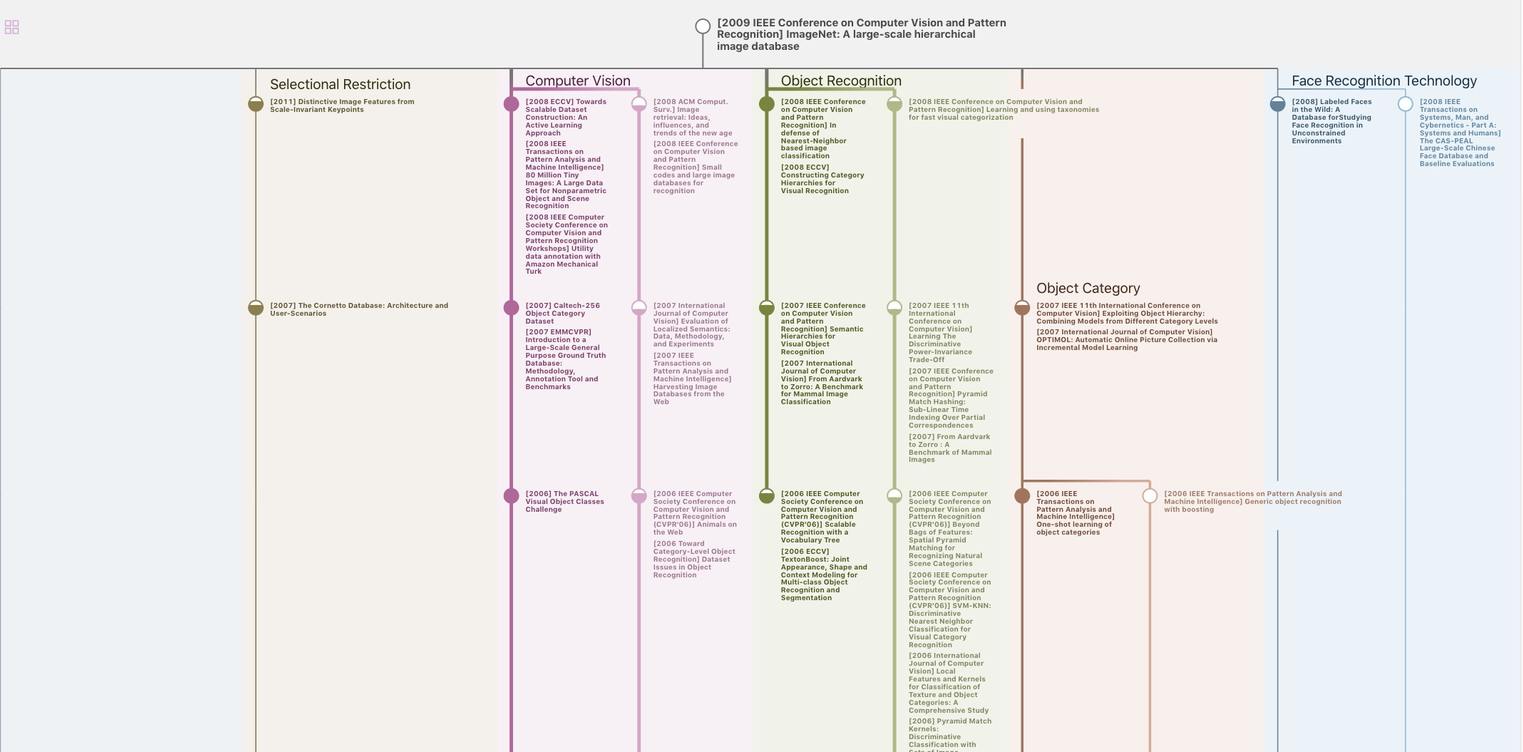
生成溯源树,研究论文发展脉络
Chat Paper
正在生成论文摘要