PROSODIC REPRESENTATION LEARNING AND CONTEXTUAL SAMPLING FOR NEURAL TEXT-TO-SPEECH
2021 IEEE INTERNATIONAL CONFERENCE ON ACOUSTICS, SPEECH AND SIGNAL PROCESSING (ICASSP 2021)(2021)
摘要
In this paper, we introduce Kathaka, a model trained with a novel two-stage training process for neural speech synthesis with contextually appropriate prosody. In Stage I, we learn a prosodic distribution at the sentence level from mel-spectrograms available during training. In Stage II, we propose a novel method to sample from this learnt prosodic distribution using the contextual information available in text. To do this, we use BERT on text, and graph-attention networks on parse trees extracted from text. We show a statistically significant relative improvement of 13.2% in naturalness over a strong baseline when compared to recordings. We also conduct an ablation study on variations of our sampling technique, and show a statistically significant improvement over the baseline in each case.
更多查看译文
关键词
TTS, prosody modelling, contextual prosody
AI 理解论文
溯源树
样例
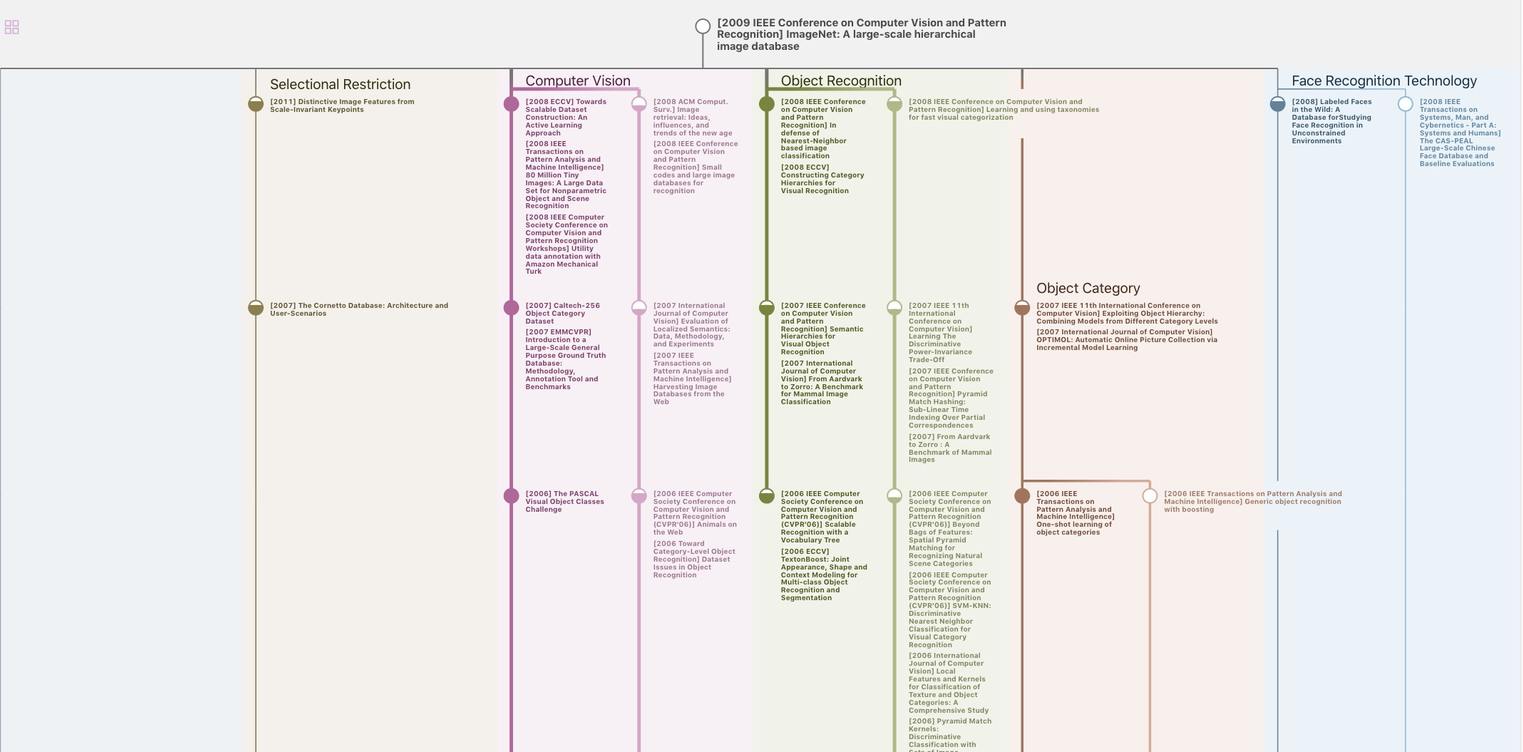
生成溯源树,研究论文发展脉络
Chat Paper
正在生成论文摘要