An Empirical-cum-Statistical Approach to Power-Performance Characterization of Concurrent GPU Kernels
arxiv(2020)
摘要
Growing deployment of power and energy efficient throughput accelerators (GPU) in data centers demands enhancement of power-performance co-optimization capabilities of GPUs. Realization of exascale computing using accelerators requires further improvements in power efficiency. With hardwired kernel concurrency enablement in accelerators, inter- and intra-workload simultaneous kernels computation predicts increased throughput at lower energy budget. To improve Performance-per-Watt metric of the architectures, a systematic empirical study of real-world throughput workloads (with concurrent kernel execution) is required. To this end, we propose a multi-kernel throughput workload generation framework that will facilitate aggressive energy and performance management of exascale data centers and will stimulate synergistic power-performance co-optimization of throughput architectures. Also, we demonstrate a multi-kernel throughput benchmark suite based on the framework that encapsulates symmetric, asymmetric and co-existing (often appears together) kernel based workloads. On average, our analysis reveals that spatial and temporal concurrency within kernel execution in throughput architectures saves energy consumption by 32%, 26% and 33% in GTX470, Tesla M2050 and Tesla K20 across 12 benchmarks. Concurrency and enhanced utilization are often correlated but do not imply significant deviation in power dissipation. Diversity analysis of proposed multi-kernels confirms characteristic variation and power-profile diversity within the suite. Besides, we explain several findings regarding power-performance co-optimization of concurrent throughput workloads.
更多查看译文
关键词
concurrent gpu kernels,empirical-cum-statistical,power-performance
AI 理解论文
溯源树
样例
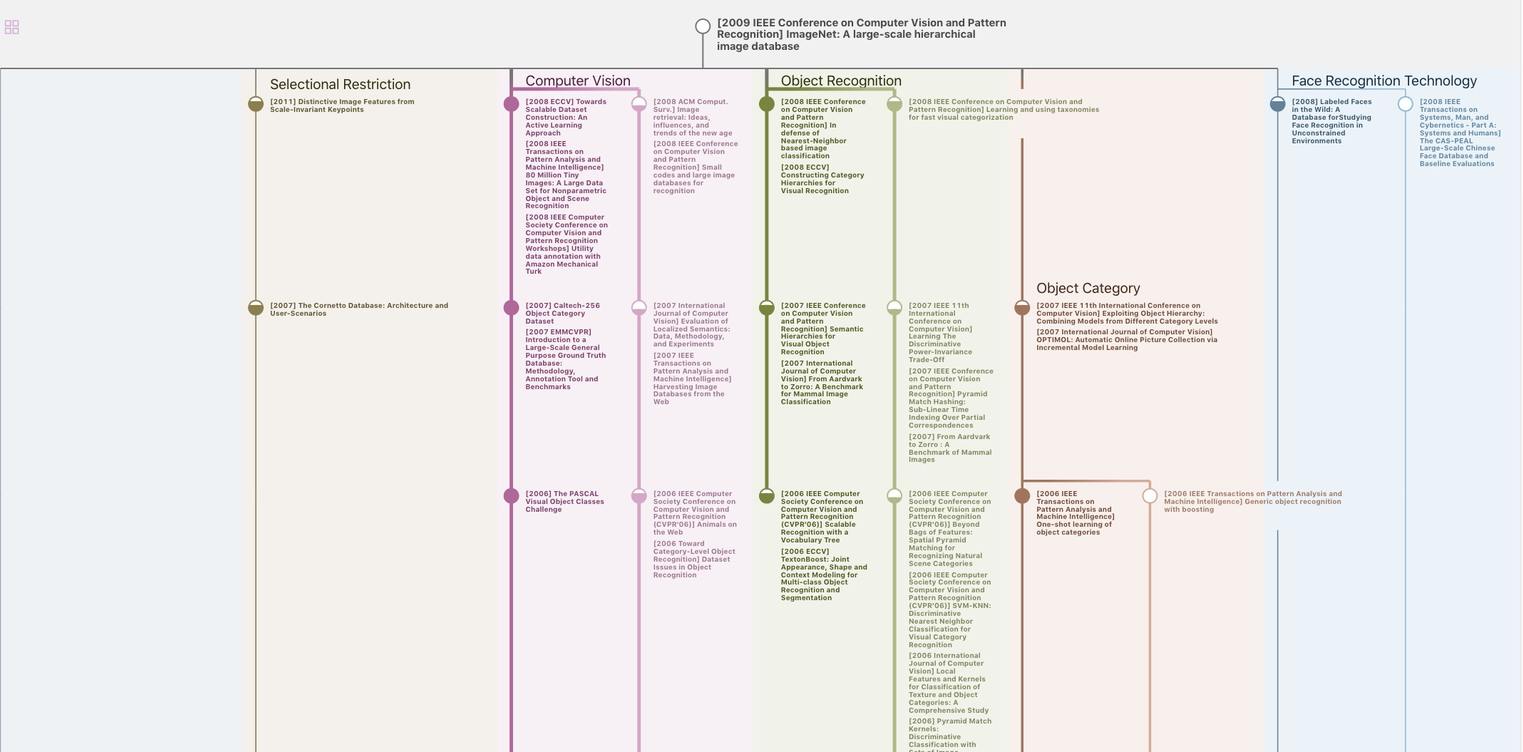
生成溯源树,研究论文发展脉络
Chat Paper
正在生成论文摘要