Neural Ordinary Differential Equations-based Explainable Deep Learning for Process Modeling
Computer Aided Chemical Engineering(2020)
摘要
Most recent advances in the machine learning domain pose the challenge of how to naturally integrate new data-driven methods with classical process models and control. We propose a process modeling framework enabling integration of data-driven algorithms through consistent topological properties and conservation of extensive quantities. Interconnections among process network units are represented through connectivity matrices and network graphs. The basic requirement is that the flow conditions can be expressed in terms of conic sector (passivity) conditions. Our formalism allows integration of fundamental conservation properties from topology with learned dynamic relations from data through sparse deep neural networks. We demonstrate in a practical example of a simple inventory control system how to integrate the basic topology of a process with a neural network ordinary differential equation model. The system specific constitutive equations are left undescribed and learned by the deep neural network using the adjoint method in combination with an adaptive ODE solver from synthetic time-series data. The resulting neural network forms a state space model for use in a model predictive control algorithm.
更多查看译文
关键词
neural ordinary differential equations,network theory,deep learning,process networks,process modelling
AI 理解论文
溯源树
样例
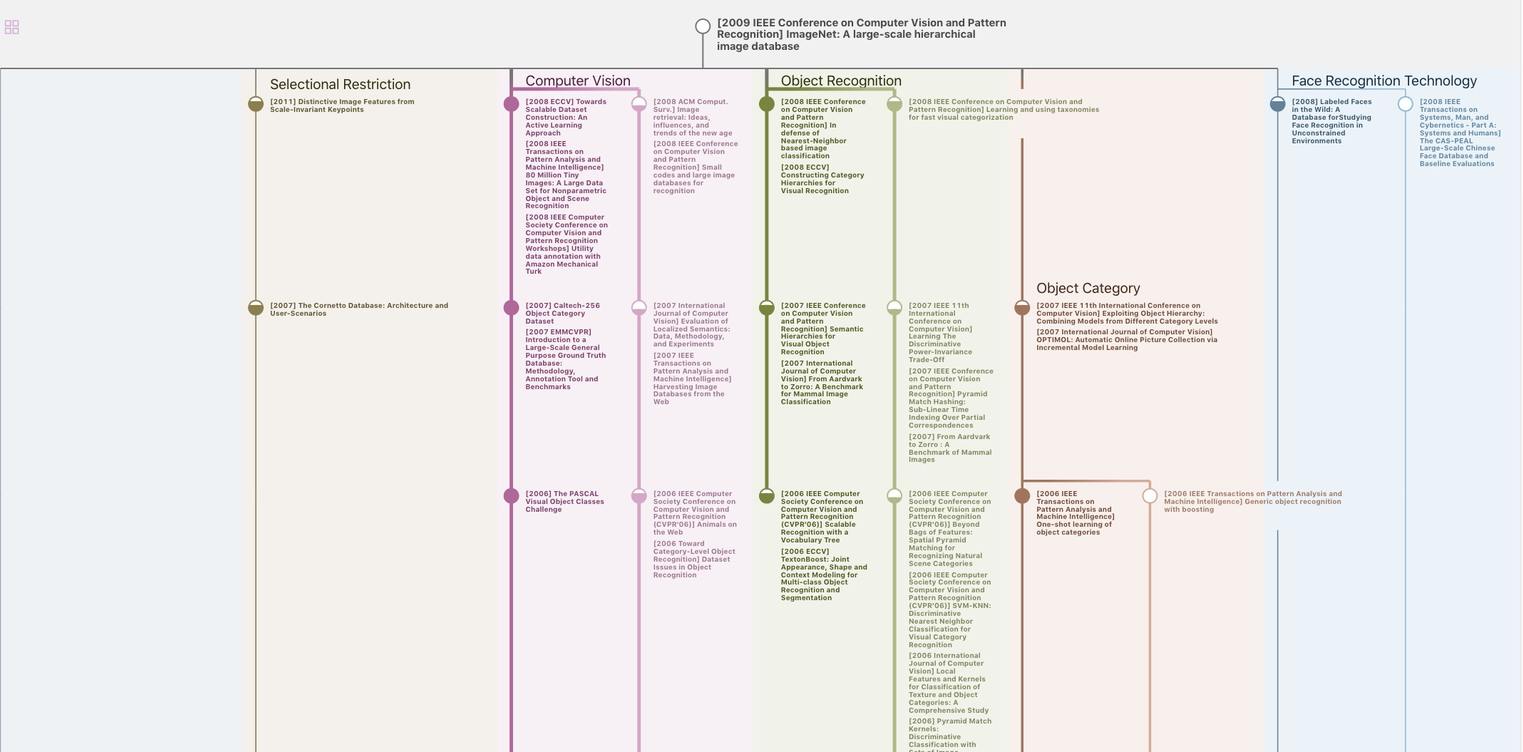
生成溯源树,研究论文发展脉络
Chat Paper
正在生成论文摘要