Inverse Learning: Solving Partially Known Models Using Inverse Optimization
arxiv(2023)
摘要
We consider the problem of learning optimal solutions of a partially known linear optimization problem and recovering its underlying cost function where a set of past decisions and the feasible set are known. We develop a new framework, denoted as Inverse Learning, that extends the inverse optimization literature to (1) learn the optimal solution of the underlying problem, (2) integrate additional information on constraints and their importance, and (3) control the balance between mimicking past behaviors and reaching new goals and rules for the learned solution. We pose inverse learning as an optimization problem that maps given (feasible and infeasible) observations to a single optimal solution with minimum perturbation, hence, not only recovering the missing cost vector but also providing an optimal solution simultaneously. The framework provides insights into an essential tradeoff in recovering linear optimization problems with regard to preserving observed behaviors and binding constraints of the known feasible set at optimality. We propose a series of mixed integer linear programming models to capture the effects of this tradeoff and validate it using a two-dimensional example. We then demonstrate the framework's applicability to a diet recommendation problem for a population of hypertension and prediabetic patients. The goal is to balance dietary constraints to achieve the necessary nutritional goals with the dietary habits of the users to encourage adherence to the diet. Results indicate that our models recommend daily food intakes that preserve the original data trends while providing a range of options to patients and providers based on the tradeoff.
更多查看译文
关键词
inverse,known models,optimization,learning
AI 理解论文
溯源树
样例
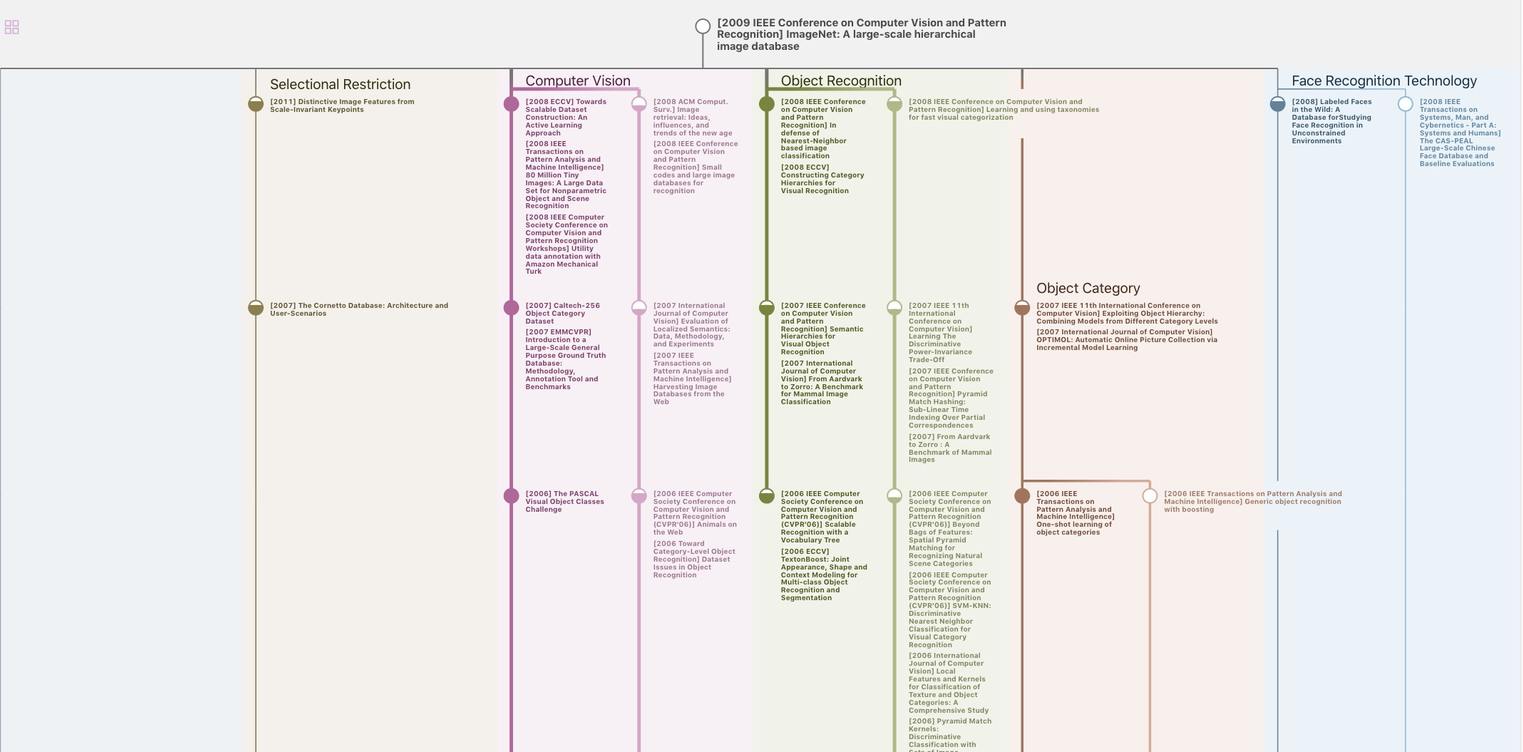
生成溯源树,研究论文发展脉络
Chat Paper
正在生成论文摘要