Mutual conditional independence and its applications to model selection in Markov networks
ANNALS OF MATHEMATICS AND ARTIFICIAL INTELLIGENCE(2020)
摘要
The fundamental concepts underlying Markov networks are the conditional independence and the set of rules called Markov properties that translate conditional independence constraints into graphs. We introduce the concept of mutual conditional independence in an independent set of a Markov network, and we prove its equivalence to the Markov properties under certain regularity conditions. This extends the notion of similarity between separation in graph and conditional independence in probability to similarity between the mutual separation in graph and the mutual conditional independence in probability. Model selection in graphical models remains a challenging task due to the large search space. We show that mutual conditional independence property can be exploited to reduce the search space. We present a new forward model selection algorithm for graphical log-linear models using mutual conditional independence. We illustrate our algorithm with a real data set example. We show that for sparse models the size of the search space can be reduced from 𝒪 (n^3) to 𝒪(n^2) using our proposed forward selection method rather than the classical forward selection method. We also envision that this property can be leveraged for model selection and inference in different types of graphical models.
更多查看译文
关键词
Markov networks,Mutual conditional independence,Graphical models,Graphical log-linear models,Forward model selection
AI 理解论文
溯源树
样例
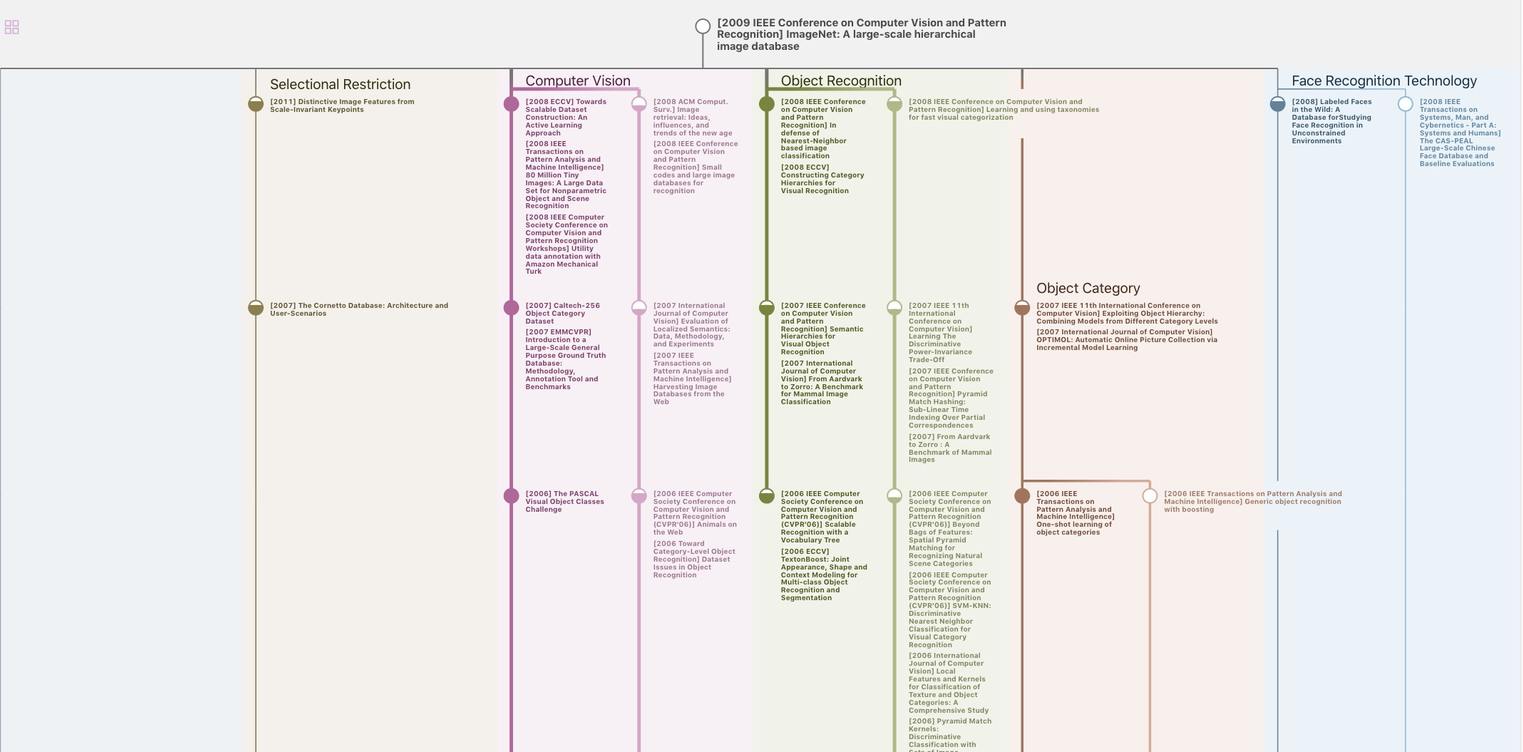
生成溯源树,研究论文发展脉络
Chat Paper
正在生成论文摘要