DEVELOPMENT OF A NOVEL TRANSLATIONAL IN SILICO INDICATION DISCOVERY FRAMEWORK: EXEMPLIFIED BY THE CLINICAL COMPOUND CENERIMOD
ANNALS OF THE RHEUMATIC DISEASES(2020)
摘要
Background: To explore the full therapeutic spectrum of a drug it is crucial to consider its potential effectiveness in all diseases. Serendipitous clinical observations have often shown that approved drugs and those in development to be efficacious in indications different to those originally tested for. Traditional approaches to match a drug candidate with possible indications are mostly based on matching drug mechanistic knowledge with disease pathophysiology. Proof-of-concept trials or elaborate pre-clinical studies in animal models do not allow for a broad assessment due to high costs and slow progress. Gene expression changes in patients or animal models represent a good proxy to comprehensively assess both disease and drug effects. Furthermore, this data type can be integrated with a plethora of publicly available data. Objectives: Generation of a novel in silico framework to support the selection and expansion of potential indications which associate with a compound or approved drug. The framework was exemplified by the clinical compound cenerimod, a potent, selective, and orally active sphingosine-1-phosphate receptor 1 modulator (Piali et al., 2017). Methods: A total of ~13’000 public patient gene expression datasets from ~140 diseases were evaluated against cenerimod gene expression data generated in mouse disease models. To improve comparability of studies across platforms and species, computer algorithms (neural networks) were trained and employed to reduce noise within the data sets and improve signal. The predicted response to cenerimod for individual patients was contrasted against clinical patient characteristics. Results: The neural network algorithm efficiently reduced experimental noise and improved sensitivity in the gene expression data. The results predicted cenerimod to be efficacious in several auto-immune diseases foremost SLE. Additionally, focused analysis on individual patients rather than disease cohorts revealed potential determinants predictive of maximal clinical response, with the highest predicted clinical response for cenerimod in patients with severe inflammatory endotype and/or high SLE Disease Activity Index (SLEDAI). Conclusion: Combining preclinical compound data with the wealth of public disease gene expression data, provides great potential to support indication selection. The novel in silico framework identified SLE as a prime potential indication for cenerimod and supported the cenerimod phase 2b clinical trial in patients with SLE (CARE study, NCT03742037). References: [1]Piali, L., Birker-Robaczewska, M., Lescop, C., Froidevaux, S., Schmitz, N., Morrison, K., … Nayler, O. (2017). Cenerimod, a novel selective S1P1 receptor modulator with unique signaling properties. Pharmacology Research \u0026 Perspectives, 5(6), 1–12. https://doi.org/10.1002/prp2.370 Disclosure of Interests: Dominik Hartl Shareholder of: Idorsia shares, Employee of: Idorsia employee, Marcel Keller Shareholder of: Idorsia options/shares, Employee of: Idorsia employee, Axel Klenk Shareholder of: Idorsia option/shares, Employee of: Idorsia employee, Mark Murphy Shareholder of: Idorsia shares and stock options, Employee of: Idorsia employee, Marianne Martinic Shareholder of: Idorsia options/shares, Employee of: Idorsia employee, Gabin Pierlot Shareholder of: Idorsia options/shares, Employee of: Idorsia employee, Peter Groenen Shareholder of: Idorsia options/shares, Employee of: Idorsia employee, Daniel Strasser Shareholder of: Idorsia options/shares, Employee of: Idorsia employee
更多查看译文
AI 理解论文
溯源树
样例
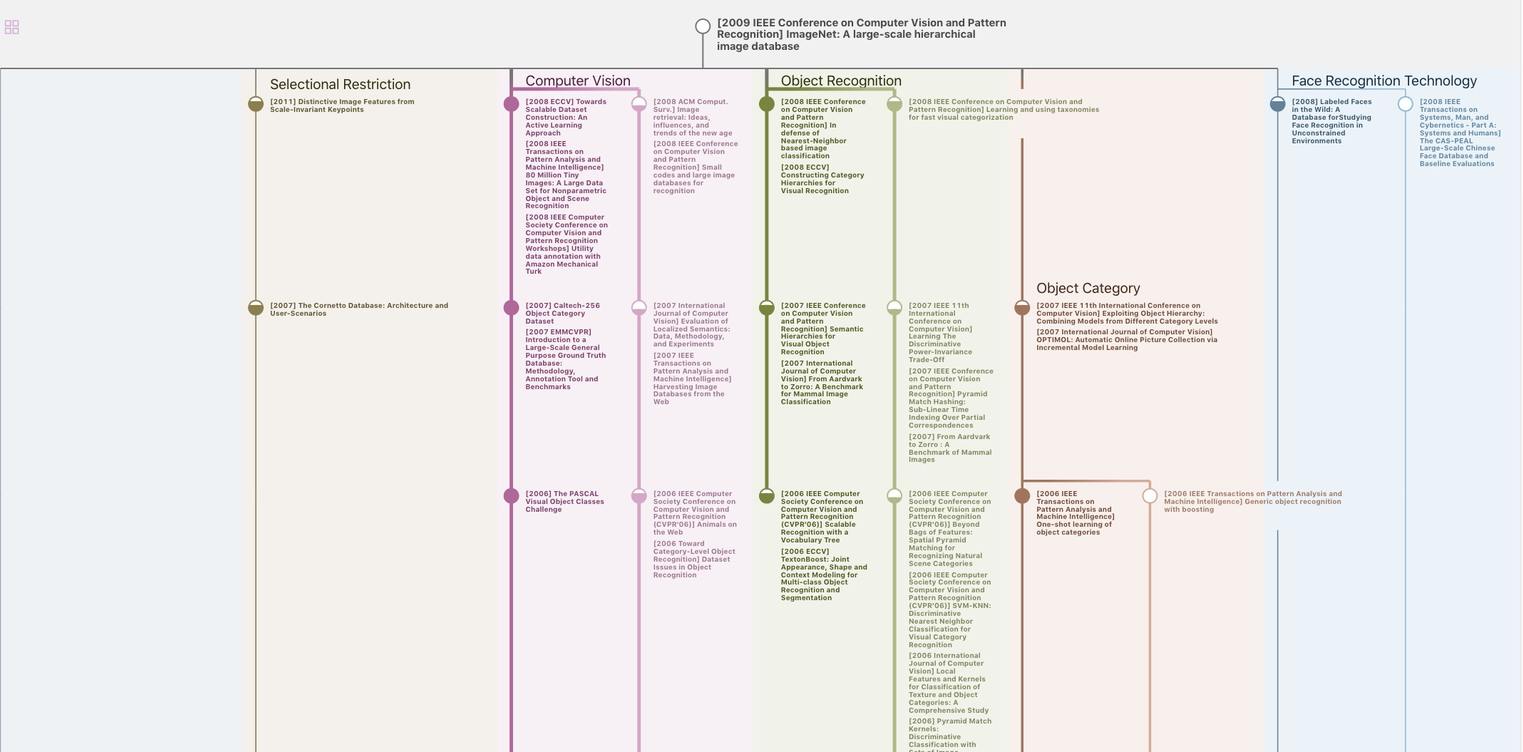
生成溯源树,研究论文发展脉络
Chat Paper
正在生成论文摘要