Machine Learning Climate Model Dynamics: Offline versus Online Performance
arxiv(2020)
摘要
Climate models are complicated software systems that approximate atmospheric and oceanic fluid mechanics at a coarse spatial resolution. Typical climate forecasts only explicitly resolve processes larger than 100 km and approximate any process occurring below this scale (e.g. thunderstorms) using so-called parametrizations. Machine learning could improve upon the accuracy of some traditional physical parametrizations by learning from so-called global cloud-resolving models. We compare the performance of two machine learning models, random forests (RF) and neural networks (NNs), at parametrizing the aggregate effect of moist physics in a 3 km resolution global simulation with an atmospheric model. The NN outperforms the RF when evaluated offline on a testing dataset. However, when the ML models are coupled to an atmospheric model run at 200 km resolution, the NN-assisted simulation crashes with 7 days, while the RF-assisted simulations remain stable. Both runs produce more accurate weather forecasts than a baseline configuration, but globally averaged climate variables drift over longer timescales.
更多查看译文
关键词
offline,climate,machine learning,online
AI 理解论文
溯源树
样例
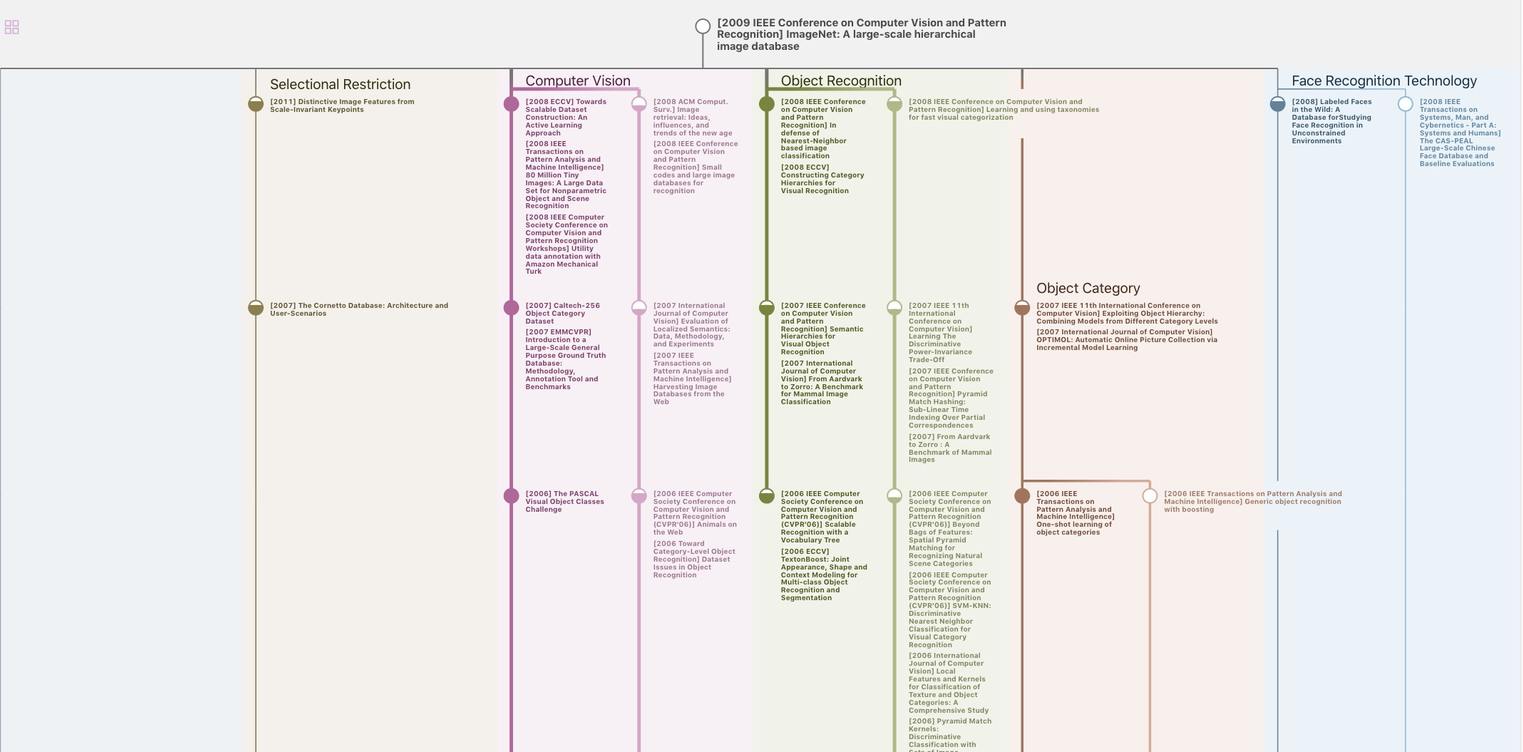
生成溯源树,研究论文发展脉络
Chat Paper
正在生成论文摘要