STReSSD - Sim-To-Real from Sound for Stochastic Dynamics.
CoRL(2020)
摘要
Sound is an information-rich medium that captures dynamic physical events. This work presents STReSSD, a framework that uses sound to bridge the simulation-to-reality gap for stochastic dynamics, demonstrated for the canonical case of a bouncing ball. A physically-motivated noise model is presented to capture stochastic behavior of the balls upon collision with the environment. A likelihood-free Bayesian inference framework is used to infer the parameters of the noise model, as well as a material property called the coefficient of restitution, from audio observations. The same inference framework and the calibrated stochastic simulator are then used to learn a probabilistic model of ball dynamics. The predictive capabilities of the dynamics model are tested in two robotic experiments. First, open-loop predictions anticipate probabilistic success of bouncing a ball into a cup. The second experiment integrates audio perception with a robotic arm to track and deflect a bouncing ball in real-time. We envision that this work is a step towards integrating audio-based inference for dynamic robotic tasks. Experimental results can be viewed at https://youtu.be/b7pOrgZrArk.
更多查看译文
关键词
stochastic dynamics,sound,sim-to-real
AI 理解论文
溯源树
样例
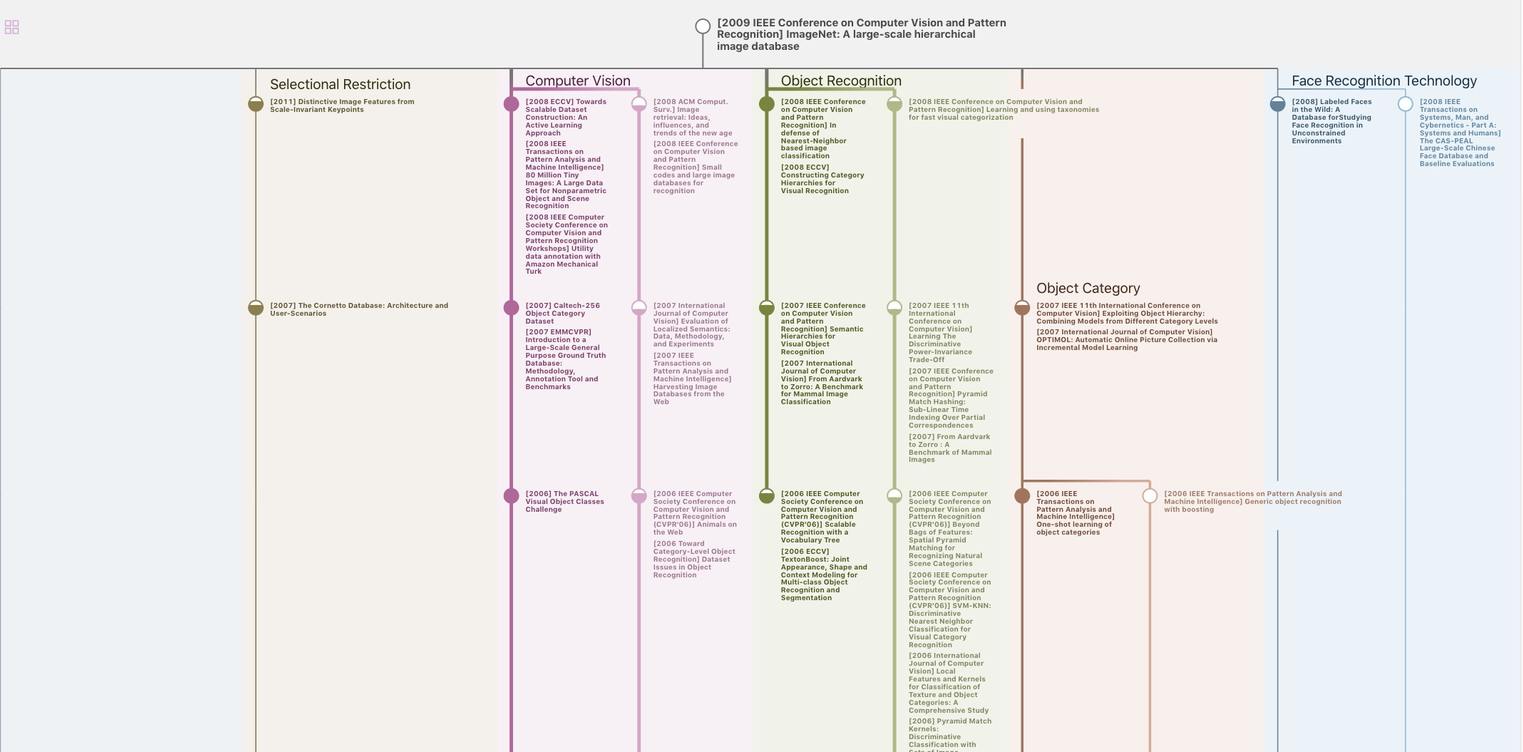
生成溯源树,研究论文发展脉络
Chat Paper
正在生成论文摘要