Leveraging an Efficient and Semantic Location Embedding to Seek New Ports of Bike Share Services
2020 IEEE INTERNATIONAL CONFERENCE ON BIG DATA (BIG DATA)(2020)
摘要
For short distance traveling in crowded urban areas, bike share services are becoming popular owing to the flexibility and convenience. To expand the service coverage, one of the key tasks is to seek new service ports, which requires to well understand the underlying features of the existing service ports. In this paper, we propose a new model, named for Efficient and Semantic Location Embedding (ESLE), which carries both geospatial and semantic information of the geo-locations. To generate ESLE, we first train a multi-label model with a deep Convolutional Neural Network (CNN) by feeding the static map-tile images and then extract location embedding vectors from the model. Compared to most recent relevant literature, ESLE is not only much cheaper in computation, but also easier to interpret via a systematic semantic analysis. Finally, we apply ESLE to seek new service ports for NTT DOCOMO's bike share services operated in Japan. The initial results demonstrate the effectiveness of ESLE, and provide a few insights that might be difficult to discover by using the conventional approaches.
更多查看译文
关键词
geo-locations,ESLE,deep convolutional neural network,systematic semantic analysis,crowded urban areas,service coverage,service ports,geospatial information,semantic information,NTT DOCOMO bike share services,efficiency and semantic location embedding,deep CNN,Japan,static map-tile images,location embedding vector extraction
AI 理解论文
溯源树
样例
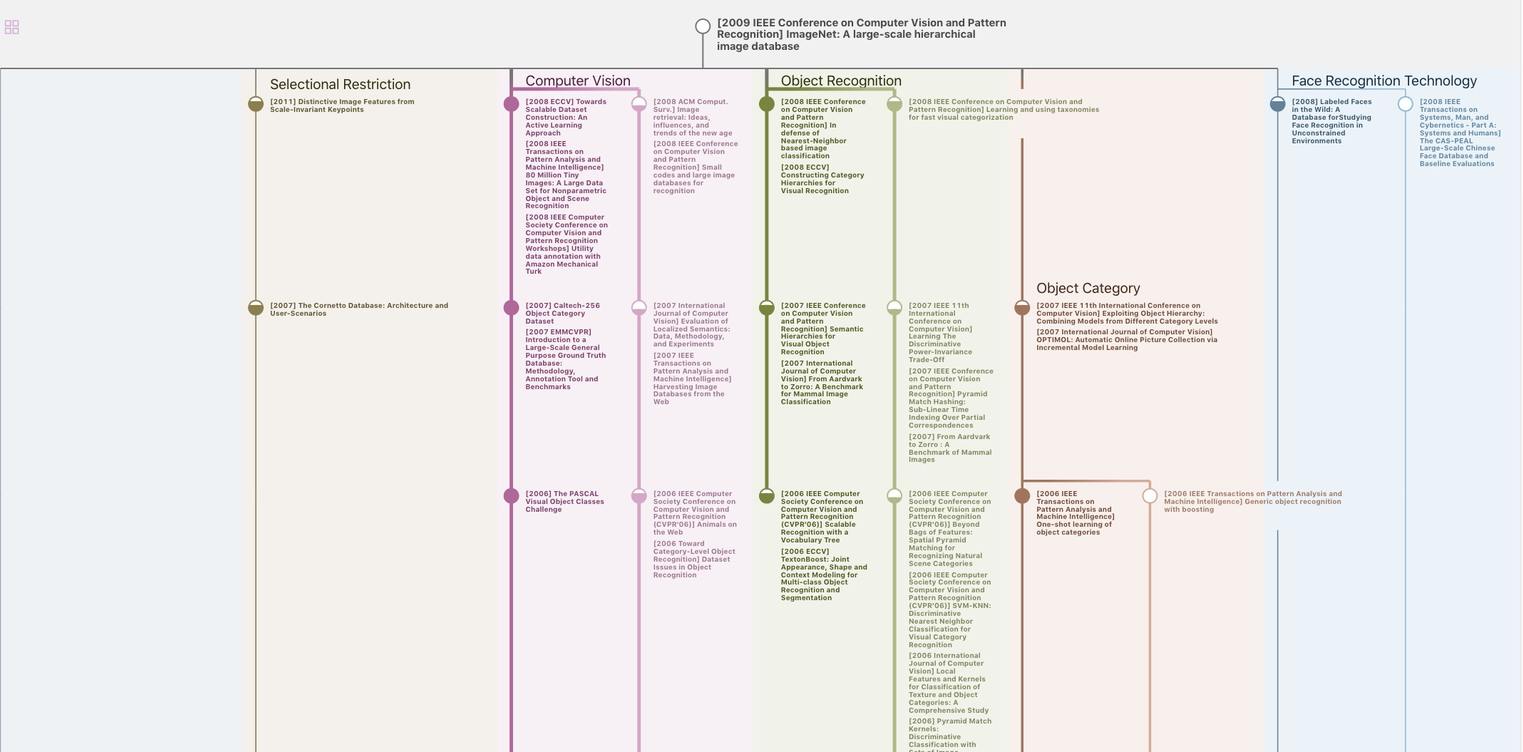
生成溯源树,研究论文发展脉络
Chat Paper
正在生成论文摘要