Network Analysis as a Grand Unifier in Biomedical Data Science
ANNUAL REVIEW OF BIOMEDICAL DATA SCIENCE, VOL 1(2018)
摘要
Biomedical data scientists study many types of networks, ranging from those formed by neurons to those created by molecular interactions. People often criticize these networks as uninterpretable diagrams termed hairballs; however, here we show that molecular biological networks can be interpreted in several straightforward ways. First, we can break down a network into smaller components, focusing on individual pathways and modules. Second, we can compute global statistics describing the network as a whole. Third, we can compare networks. These comparisons can be within the same context (e.g., between two gene regulatory networks) or cross-disciplinary (e.g., between regulatory networks and governmental hierarchies). The latter comparisons can transfer a formalism, such as that for Markov chains, from one context to another or relate our intuitions in a familiar setting (e.g., social networks) to the relatively unfamiliar molecular context. Finally, key aspects of molecular networks are dynamics and evolution, i.e., how they evolve over time and how genetic variants affect them. By studying the relationships between variants in networks, we can begin to interpret many common diseases, such as cancer and heart disease.
更多查看译文
关键词
network analysis,molecular interaction,systems biology,cross-disciplinary research,network medicine
AI 理解论文
溯源树
样例
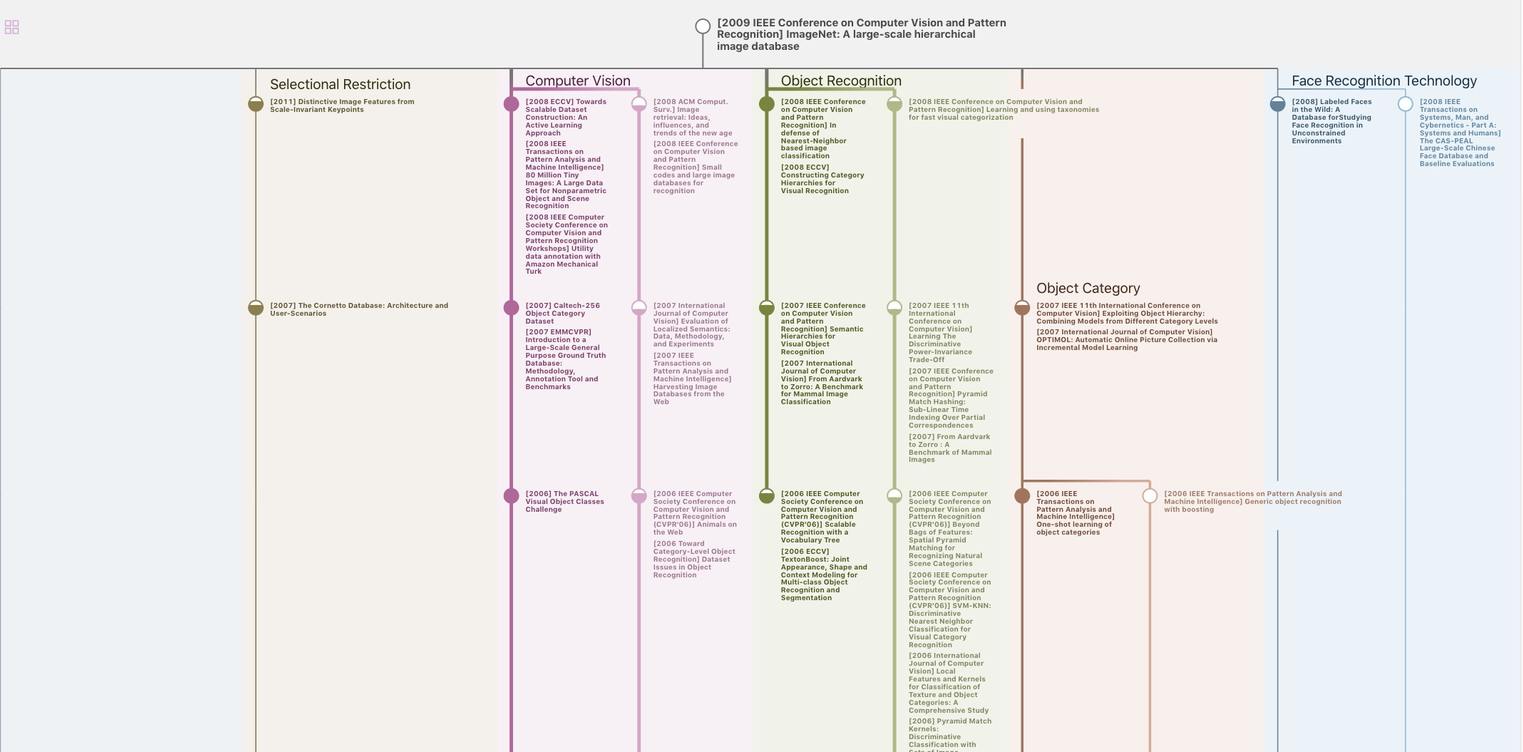
生成溯源树,研究论文发展脉络
Chat Paper
正在生成论文摘要