A Few Shot Adaptation of Visual Navigation Skills to New Observations using Meta-Learning
2021 IEEE INTERNATIONAL CONFERENCE ON ROBOTICS AND AUTOMATION (ICRA 2021)(2021)
摘要
Target-driven visual navigation is a challenging problem that requires a robot to find the goal using only visual inputs. Many researchers have demonstrated promising results using deep reinforcement learning (deep RL) on various robotic platforms, but typical end-to-end learning is known for its poor extrapolation capability to new scenarios. Therefore, learning a navigation policy for a new robot with a new sensor configuration or a new target still remains a challenging problem. In this paper, we introduce a learning algorithm that enables rapid adaptation to new sensor configurations or target objects with a few shots. We design a policy architecture with latent features between perception and inference networks and quickly adapt the perception network via meta-learning while freezing the inference network. Our experiments show that our algorithm adapts the learned navigation policy with only three shots for unseen situations with different sensor configurations or different target colors. We also analyze the proposed algorithm by investigating various hyperparameters.
更多查看译文
关键词
meta-learning,inference network,learned navigation policy,sensor configurations,target colors,shot adaptation,visual navigation skills,visual inputs,deep reinforcement learning,deep RL,robotic platforms,typical end-to-end learning,poor extrapolation capability,sensor configuration,learning algorithm,rapid adaptation,target objects,policy architecture,inference networks,perception network
AI 理解论文
溯源树
样例
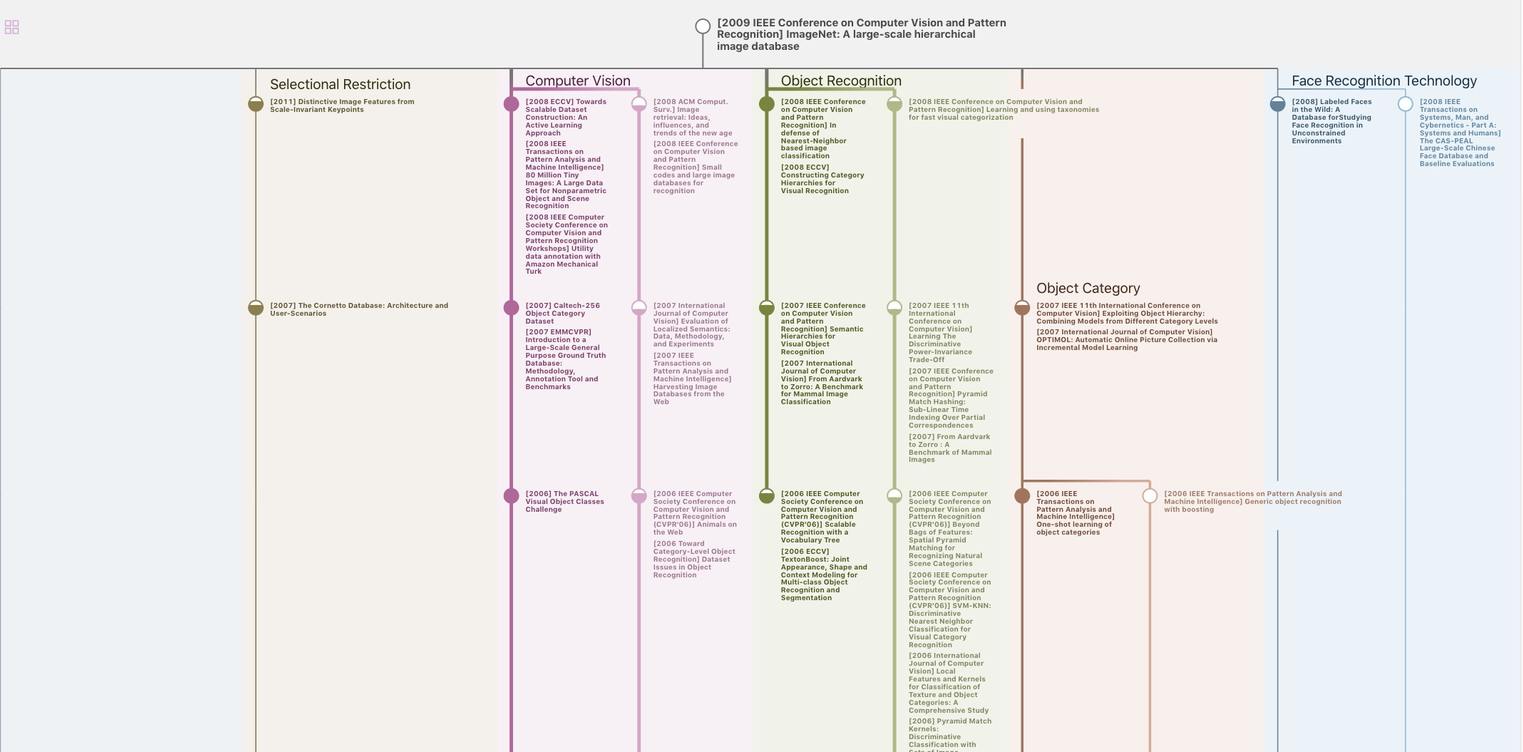
生成溯源树,研究论文发展脉络
Chat Paper
正在生成论文摘要