Lcollision: Fast Generation Of Collision-Free Human Poses Using Learned Non-Penetration Constraints
THIRTY-FIFTH AAAI CONFERENCE ON ARTIFICIAL INTELLIGENCE, THIRTY-THIRD CONFERENCE ON INNOVATIVE APPLICATIONS OF ARTIFICIAL INTELLIGENCE AND THE ELEVENTH SYMPOSIUM ON EDUCATIONAL ADVANCES IN ARTIFICIAL INTELLIGENCE(2021)
摘要
We present LCollision, a learning-based method that synthesizes collision-free 3D human poses. At the crux of our approach is a novel deep architecture that simultaneously decodes new human poses from the latent space and predicts colliding body parts. These two components of our architecture are used as the objective function and surrogate hard constraints in a constrained optimization for collision-free human pose generation. A novel aspect of our approach is the use of a bilevel autoencoder that decomposes whole-body collisions into groups of collisions between localized body parts. By solving the constrained optimizations, we show that a significant amount of collision artifacts can be resolved. Furthermore, in a large test set of 2.5 x 10(6) randomized poses from SCAPE, our architecture achieves a collision-prediction accuracy of 94.1% with 80x speedup over exact collision detection algorithms. To the best of our knowledge, LCollision is the first approach that accelerates collision detection and resolves penetrations using a neural network.
更多查看译文
AI 理解论文
溯源树
样例
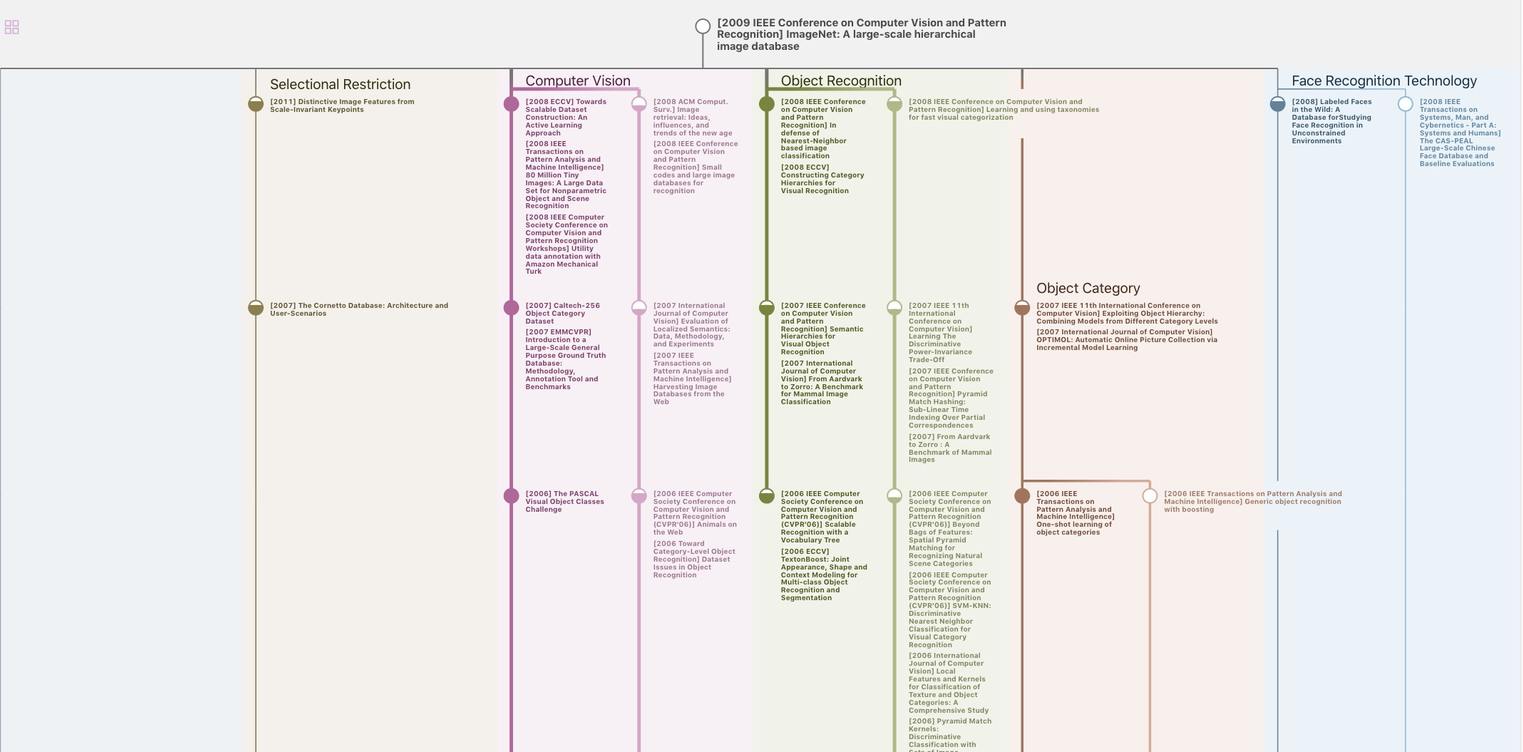
生成溯源树,研究论文发展脉络
Chat Paper
正在生成论文摘要