Know what you don't need: Single-Shot Meta-Pruning for attention heads
AI Open(2021)
摘要
Deep pre-trained Transformer models have achieved state-of-the-art results over a variety of natural language processing (NLP) tasks. By learning rich language knowledge with millions of parameters, these models are usually overparameterized and significantly increase the computational overhead in applications. It is intuitive to address this issue by model compression. In this work, we propose a method, called Single-Shot Meta-Pruning, to compress deep pre-trained Transformers before fine-tuning. Specifically, we focus on pruning unnecessary attention heads adaptively for different downstream tasks. To measure the informativeness of attention heads, we train our Single-Shot Meta-Pruner (SMP) with a meta-learning paradigm aiming to maintain the distribution of text representations after pruning. Compared with existing compression methods for pre-trained models, our method can reduce the overhead of both fine-tuning and inference. Experimental results show that our pruner can selectively prune 50% of attention heads with little impact on the performance on downstream tasks and even provide better text representations. The source code is available at https://github.com/thunlp/SMP.
更多查看译文
关键词
Pre-trained language models,Model compression
AI 理解论文
溯源树
样例
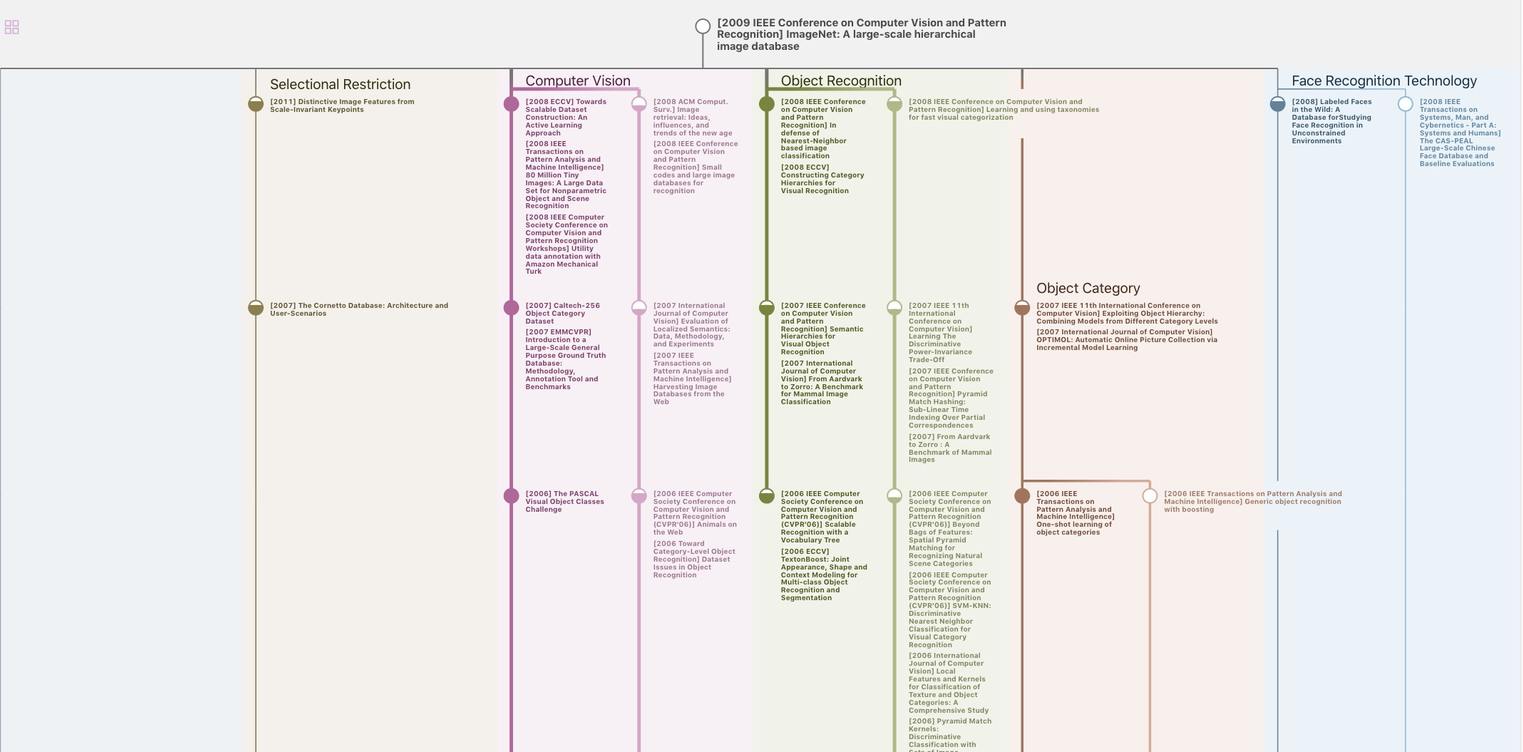
生成溯源树,研究论文发展脉络
Chat Paper
正在生成论文摘要